A Demand Forecasting Strategy Based on a Retrofit Architecture for Remote Monitoring of Legacy Building Circuits
SUSTAINABILITY(2023)
摘要
Energy demand forecasting is crucial for planning and optimizing the use of energy resources in building facilities. However, integrating digital solutions and learning techniques into legacy buildings presents significant challenges due to limited or outdated resources, hampering predictive analytics in these buildings and their circuits. To fill this gap, this article proposes an innovative demand forecasting strategy using an AIoT retrofit architecture based on the SmartLVGrid metamodel. This architecture allows remote monitoring of legacy building circuits, facilitating the collection, processing and storage of data in the cloud. We use several learning algorithms, including linear regression, support vector regressor, random forest regressor, XGBoost regressor, and long short-term memory (LSTM) neural network, to predict energy demand 15 min ahead, identifying potential overruns of contracted demand in accordance with Brazilian regulations. After Bayesian optimization, the LSTM neural network outperformed other models for most of the selected datasets and detected 32 out of 38 demand overruns on the test set. XGBoost and random forest followed closely, detecting 30 demand overruns. Overall, our cost-effective solution optimizes energy usage and efficiently mitigates potential demand exceedances in building installations. This is achieved through a step-by-step approach to upgrading existing aging facilities, which promotes energy efficiency and sustainability.
更多查看译文
关键词
retrofit architecture,demand forecasting strategy,remote monitoring
AI 理解论文
溯源树
样例
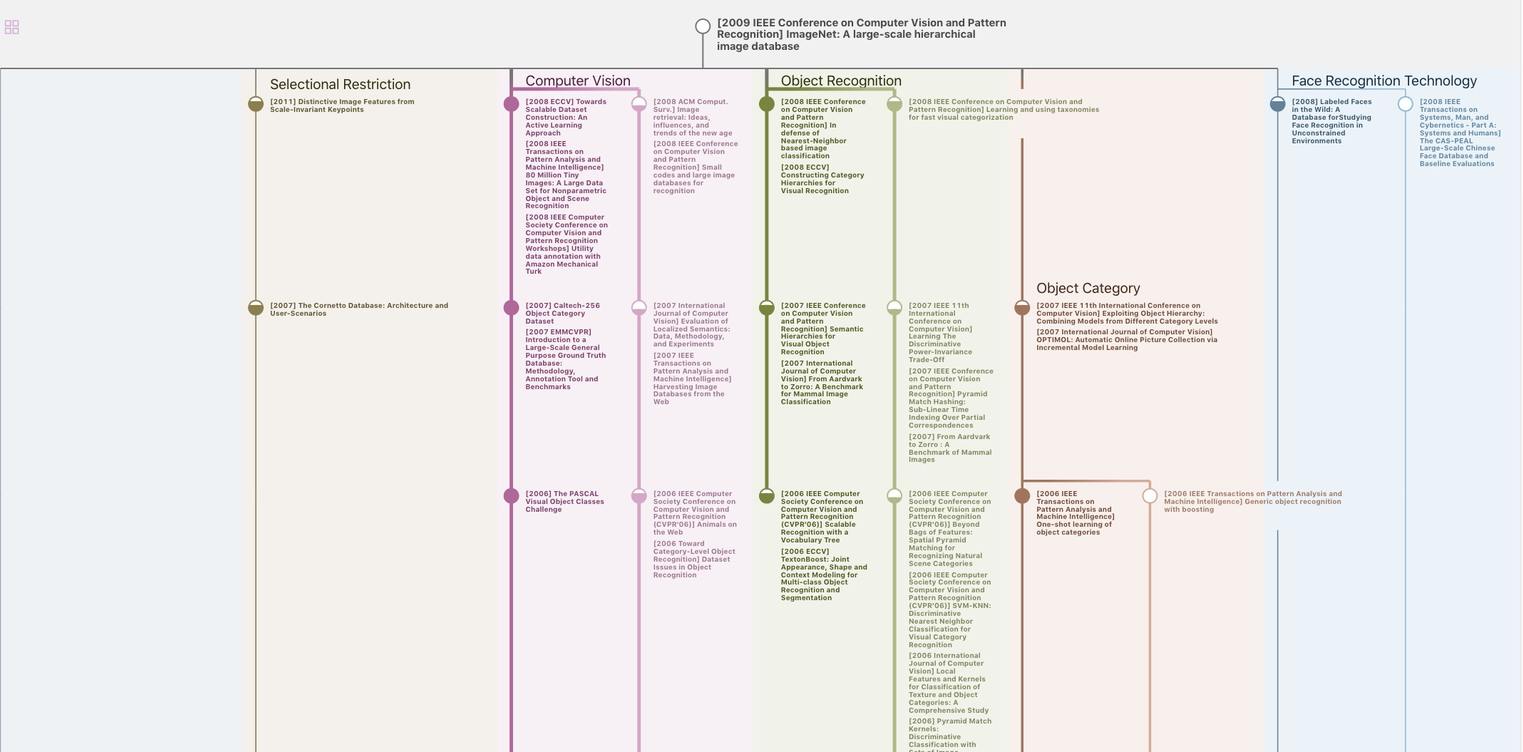
生成溯源树,研究论文发展脉络
Chat Paper
正在生成论文摘要