A Framework for RQD Calculation Based on Deep Learning
MINING METALLURGY & EXPLORATION(2023)
摘要
The rock quality designation (RQD) is a commonly used index for the classification and evaluation of rock mass quality and is widely adopted in mining and geological engineering. The traditional method of obtaining RQD still requires manual measurement of core length and calculation of RQD, which is inefficient. To address this problem, we propose a framework for drilling core image segmentation and RQD estimation from digital images of cores in core boxes based on the deep learning algorithm. The proposed framework is generated by combining the Mask Region-Based Convolutional Neural Networks (Mask R-CNN) instance segmentation algorithm, the U-Shaped Convolutional Neural Networks (U-Net) semantic segmentation algorithm, and the image processing functions of the Open Source Computer Vision Library (OpenCV). To demonstrate the accuracy of the proposed method, seven boreholes in the Xiushuihe vanadium-titanium magnetite mine, located in the Sichuan Province, China, were used as the case study. According to the comparison of the manual measurements and calculations of the cores taken from seven boreholes used to conduct this study, the framework can record the length of the drill cores and calculate RQD within an average error rate of 3.42%, while it saves about 85% of the working time. The results illustrate that the proposed framework enhances the efficiency of RQD calculation while satisfying the engineering requirements.
更多查看译文
关键词
RQD,Deep learning algorithm,Image segmentation,OpenCV
AI 理解论文
溯源树
样例
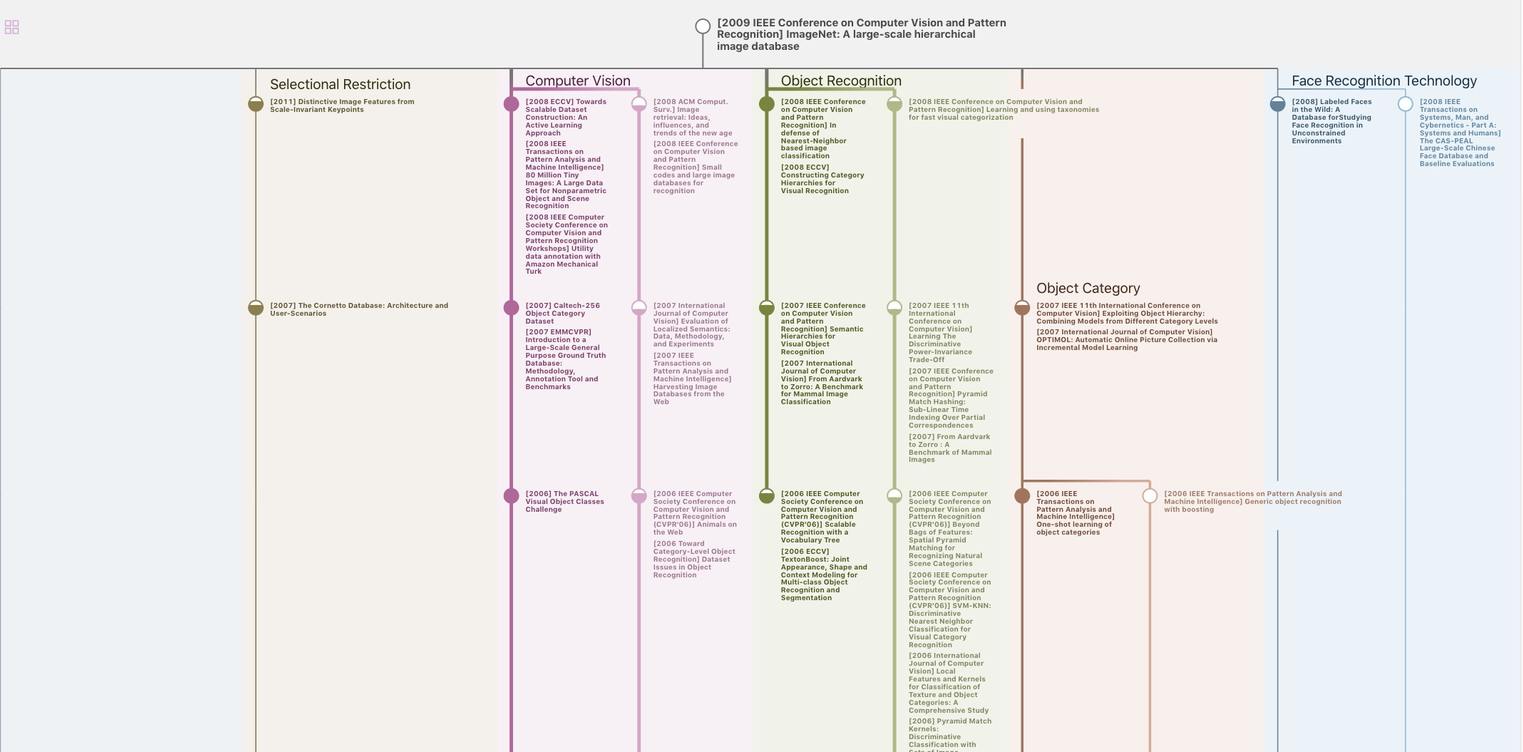
生成溯源树,研究论文发展脉络
Chat Paper
正在生成论文摘要