AD-YOLOv5s based UAV detection for low altitude security
INTERNATIONAL JOURNAL OF MICRO AIR VEHICLES(2023)
摘要
UAV (Unmanned Aerial Vehicle) black flight at low altitude could cause serious safety risks. Consequently, it is crucial to detect and manage low altitude small UAVs. The existing methods of low altitude small UAV detection suffer from problems such as high false alarm rate, and poor real-time performance. In order to solve the above problems, we present a novel approach, named AD-YOLOv5s, to achieve low altitude small UAV detection with high precision and high real-time performance. Firstly, the feature enhancement method is used to expand the dataset. We optimize the model feature fusion, the prediction head structure, and the loss function. Based on the CBAM (Convolutional Block Attention Module) attention mechanism, feature enhancement is performed to improve the detection accuracy. Secondly, the ghost module and depthwise separable convolution are used to reduce the number of parameters of the model, and we propose the method of lightweight design of model to improve the detection speed. Compared with the YOLOv5s model, the experiment result shows that our proposed AD-YOLOv5s model improves the value of mAP by 2.2% and the value of Recall by 1.8%, reduces the value of GFLOPs by 29.9% and parameters by 38.8%, and achieves 27.6 FPS when the proposed model deploy on a low-cost edge computing device (jetson nano).
更多查看译文
关键词
Low Altitude Security, object detection, embedded deployment, deep learning, UAV
AI 理解论文
溯源树
样例
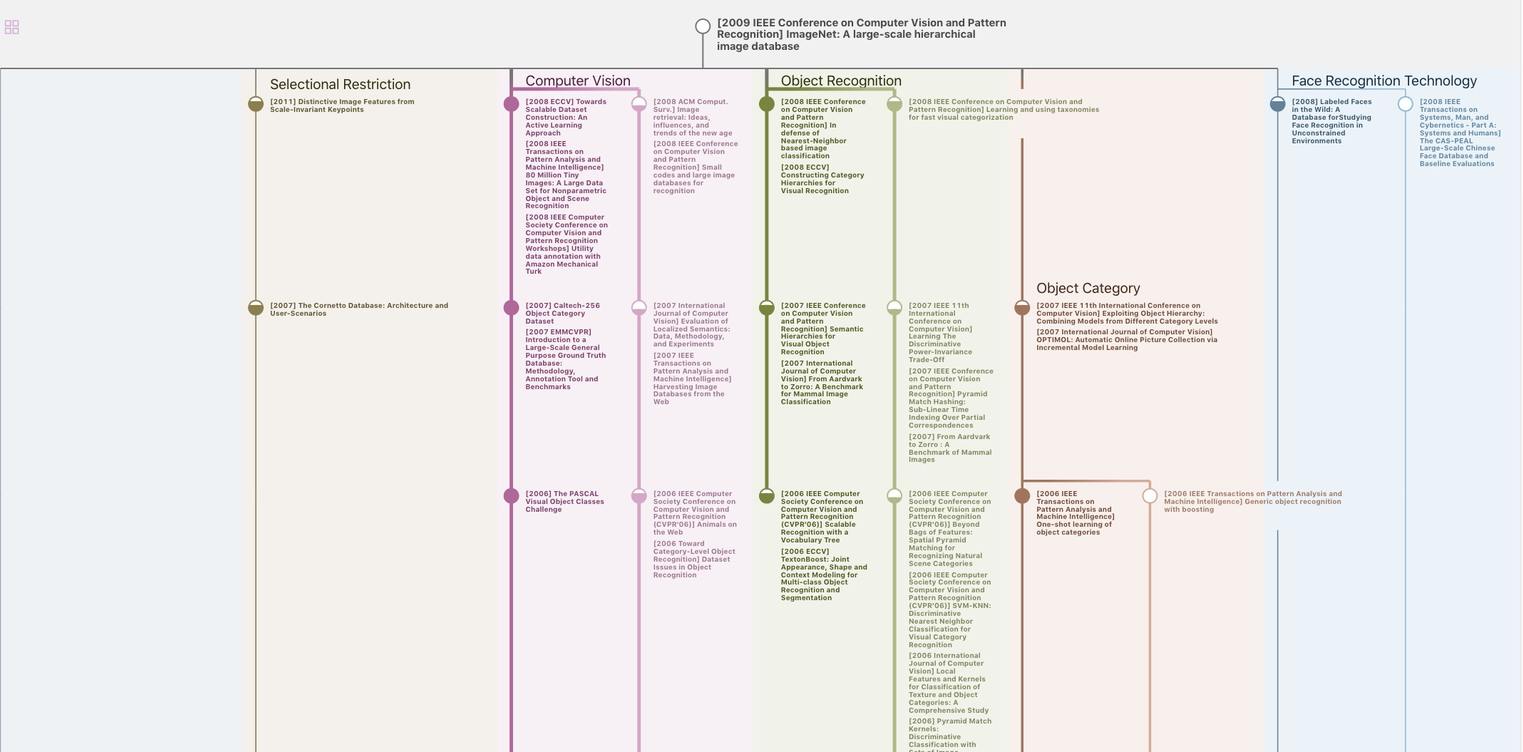
生成溯源树,研究论文发展脉络
Chat Paper
正在生成论文摘要