Experimental modal transferring of industrial robot with data augmentation through domain adaptation and transfer boosting
MECHANICAL SYSTEMS AND SIGNAL PROCESSING(2023)
摘要
Pose-dependent modal properties of the robotic milling system determine the milling stability, which significantly affects the machining accuracy and surface quality. Experimental modal analysis is an effective way to obtain the actual modal properties, however, the full experimental modal measurement in the entire robot workspace is repetitive and time-consuming, and the modal properties of a measured workspace can hardly transfer to an unmeasured workspace because of domain discrepancy and data imbalance. In this paper, the mathematical formalization of modal properties transferring problem between different workspaces is recommended as heterogeneous transfer, and a transfer learning method of modal properties is proposed by integrating domain adaptation and transfer boosting to eliminate domain discrepancy and data imbalance. Firstly, a workspace is selected as the source workspace in which pose-dependent modal properties are obtained as the source data through a series of sufficient impact tests. For a new workspace, namely the target workspace, a few impact tests would be sufficient to obtain the modal properties as the target data. Then, both the inputs of source and target data are projected onto a reproducing kernel Hilbert space (RKHS) through transfer component analysis (TCA) to get the IN-Domain (IND) inputs. The source data is used to generate pseudo data with data augmentation through affine transformation, and the pseudo data is regarded as coarse data for the target workspace. Finally, the precise modal properties models are trained with TrAdaBoost.R2 in which the training data was obtained by mixing pseudo data and target data, and the unmeasured modal properties in the target space are predicted. The effectiveness of the proposed method is verified through experimental modal properties transferring between different workspaces and modal orders for the robotic milling system, and the comparisons with Gaussian process regression (GPR) demonstrate the outperformance of the proposed method.
更多查看译文
关键词
experimental modal transferring,domain adaptation,data augmentation,industrial robot
AI 理解论文
溯源树
样例
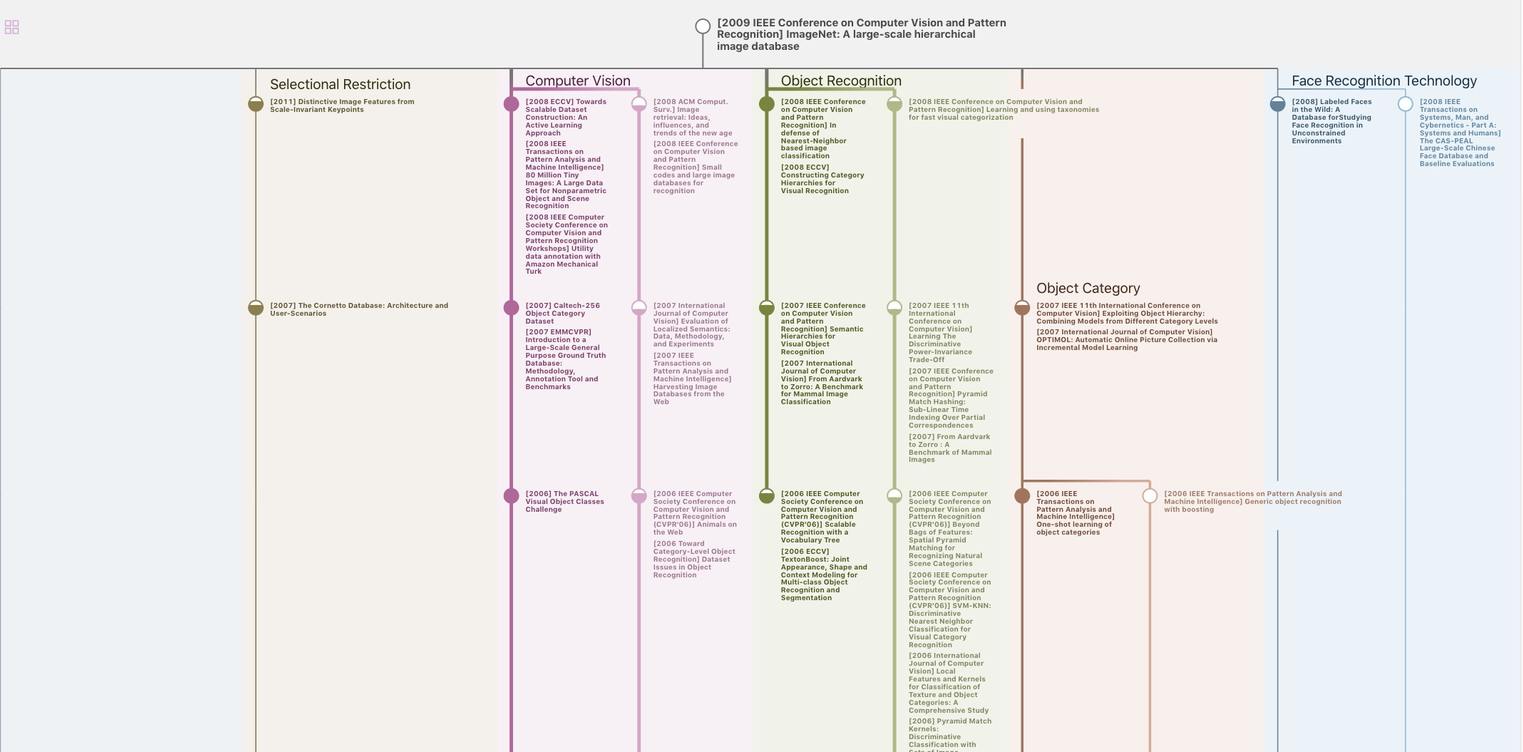
生成溯源树,研究论文发展脉络
Chat Paper
正在生成论文摘要