A Ginseng Appearance Quality Grading Method Based on an Improved ConvNeXt Model
AGRONOMY-BASEL(2023)
摘要
In order to solve the problem of the small degree of variability between the features of ginseng grading classes and the resulting need for heavy reliance on professionals, this study established a ginseng dataset containing 5116 images with three classes in different contexts and proposed a ginseng-grading model based on an improved ConvNeXt framework. Firstly, a Channel Shuffle module was embedded in the backbone network after down-sampling to fully fuse the channel features and improve the model's grading accuracy. The model's characterization ability enriched the feature space of the convolutional block and further improved the model's accuracy. Finally, the original activation function, GELU, was replaced with the PreLU activation function to increase the nonlinear variability of the neural network model and improve the model's accuracy and efficiency. The experimental results show that the method demonstrated accuracy improvements of 2.46% and 4.32%, respectively, compared with the current advanced networks, Vision Transformer and Swim Transformer. Furthermore, the accuracy, precision, recall, and specificity of ginseng classification reached values of 94.44%, 91.58%, 91.04%, and 95.82%, respectively, and the loss rate was reduced to 0.24. A comparison with expert appraisal results showed high consistency, thus verifying our model's accuracy and reliability in ginseng quality assessment and its ability to provide technical support for intelligent ginseng quality grading.
更多查看译文
关键词
deep learning,ginseng grading,ConvNeXt,Channel Shuffle,structural re-parameterization,activation function
AI 理解论文
溯源树
样例
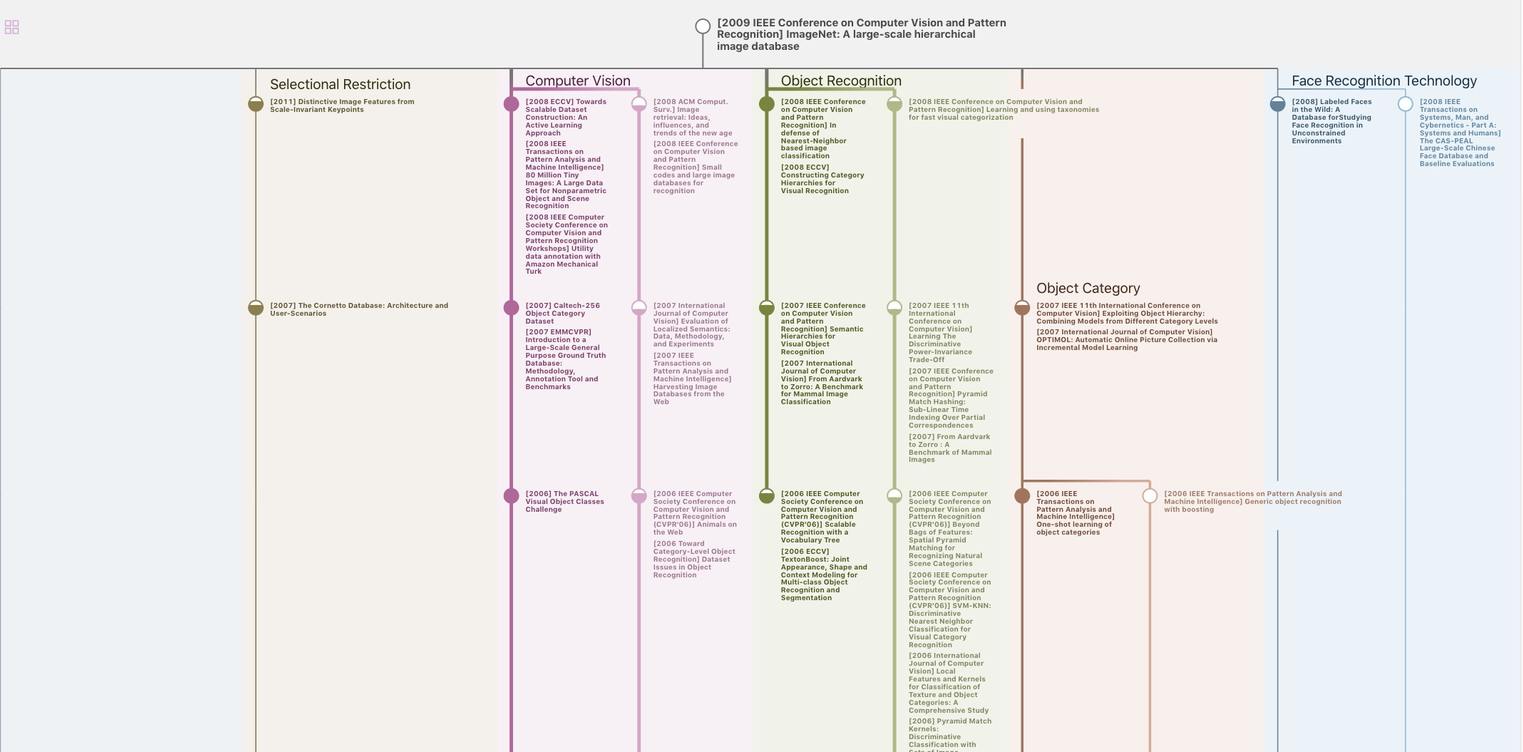
生成溯源树,研究论文发展脉络
Chat Paper
正在生成论文摘要