Physics-Informed Neural Networks for System Identification of Structural Systems with a Multiphysics Damping Model
Journal of engineering mechanics(2023)
摘要
Structural system identification is critical in resilience assessments and structural health monitoring, especially following natural hazards. Among the nonlinear structural behaviors, structural damping is a complex behavior that can be modeled as a multiphysics system wherein the structure interacts with an external thermal bath and undergoes thermalization. In this paper, we propose a novel physics-informed neural network approach for nonlinear structural system identification and demonstrate its application in multiphysics cases where the damping term is governed by a separated dynamics equation. The proposed approach, called PIDynNet, improves the estimation of the parameters of nonlinear structural systems by integrating auxiliary physics-based loss terms, one for the structural dynamics and one for the thermal transfer. These physics-based loss terms form the overall loss function in addition to a supervised data-based loss term. To ensure effective learning during the identification process, subsampling and early stopping strategies are developed. The proposed framework also has the generalization capability to predict nonlinear responses for unseen ground excitations. Two numerical experiments of nonlinear systems are conducted to demonstrate the comparative performance of PIDynNet.
更多查看译文
关键词
Structural Damage Detection,Nonlinear Dynamics,Structural Dynamics,Vibration-based Damage Identification,Defect Detection
AI 理解论文
溯源树
样例
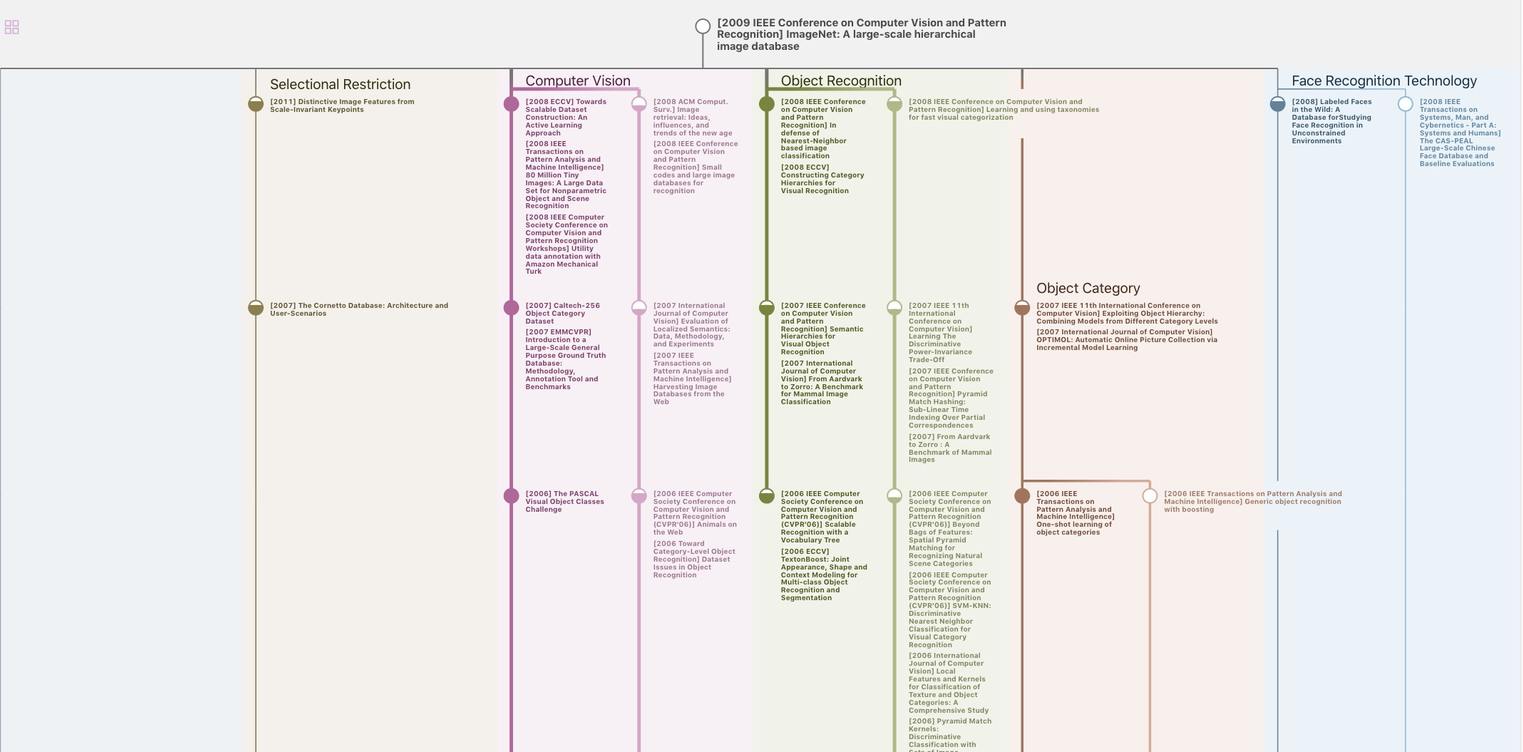
生成溯源树,研究论文发展脉络
Chat Paper
正在生成论文摘要