Gauss-Seidel based spatially varying optimal regularization improves reconstruction in diffuse optical tomography
IMAGING SCIENCE JOURNAL(2023)
摘要
The inverse problem associated with Diffuse optical tomography image reconstruction is known to be highly nonlinear, under-determined, and ill-posed. The Levenberg-Marquardt technique is employed in solving it and is known to produce low-resolution reconstructed images. To stabilize the inversion of the large matrix, a heuristically chosen regularization parameter is used. A novel methodology is developed using Gauss-Seidel, Modified Richardson, and Kaczmarz recursive methods to solve the inverse problem and to obtain spatially varying regularization parameters, which are optimally obtained for every node automatically, which is otherwise not possible. The proposed methods are thoroughly compared with the existing traditional methods in both 2-D and 3-D imaging domains using numerically simulated noisy data and also real-life phantom data. Of all the proposed methods, the Gauss-Seidel-based method provides a quantitatively accurate estimation of spatially varying regularization using the model-resolution-matrix-based method and hence improves the quality of the reconstructed images with better resolution characteristics.
更多查看译文
关键词
Diffuse optical tomography, inverse problem, regularization, recursive methods, optimization, near infrared, image reconstruction, gauss-seidel
AI 理解论文
溯源树
样例
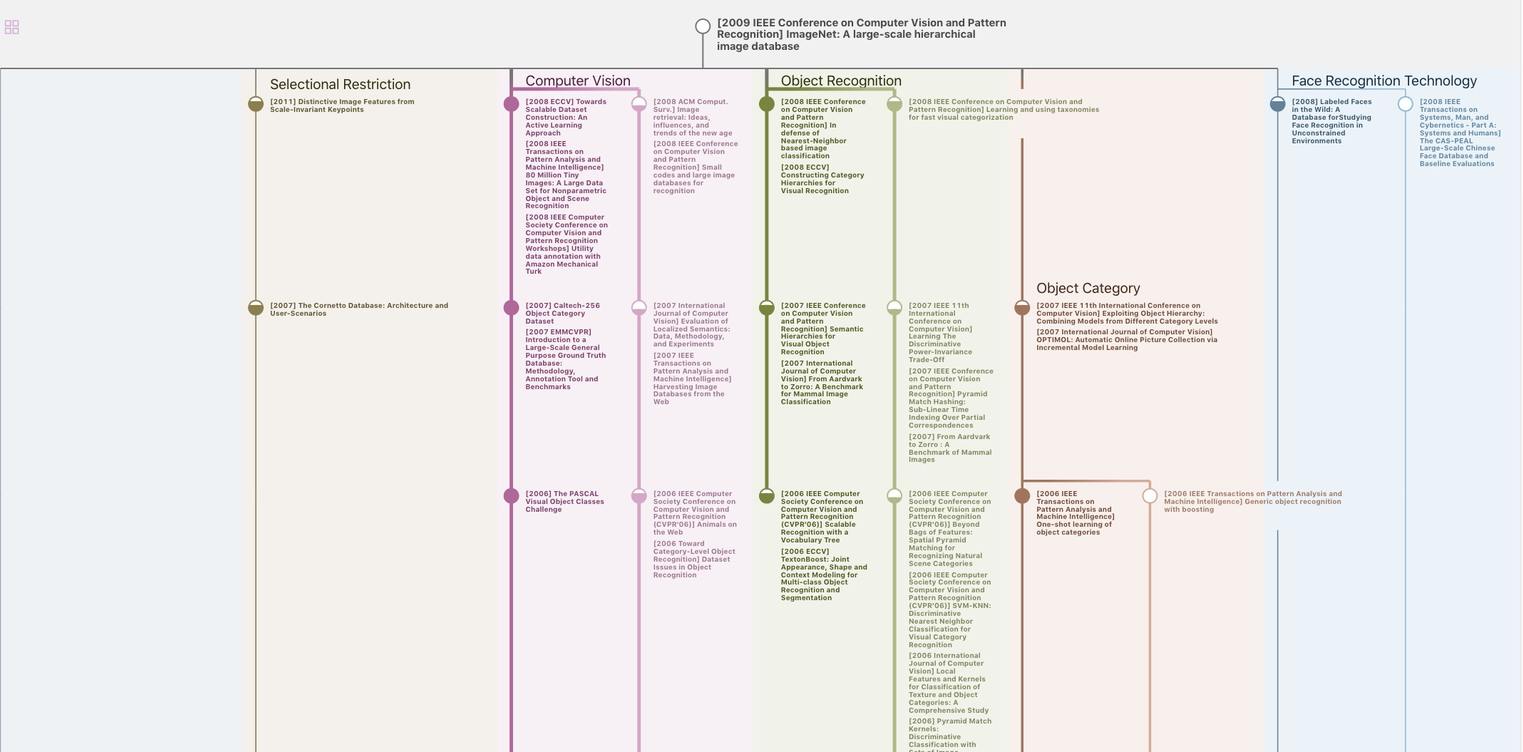
生成溯源树,研究论文发展脉络
Chat Paper
正在生成论文摘要