Remaining Useful Life Prediction Based on Incremental Learning
IEEE TRANSACTIONS ON RELIABILITY(2023)
摘要
Remaining useful life (RUL) prediction based on machine learning assumes that there are enough representative data for training models. However, it is impossible to have so many representative data considering security, economy factors, and so on. Thus, an incremental learning based RUL prediction approach is proposed to address this problem. First, a novel sequence input vector is constructed from the limited condition monitoring data, and it is proved that the input subspace have orthogonal properties, which is a necessary assumption to ensure the existence of a projector. Second, a projector is constructed to find a weight configuration for avoiding catastrophic forgetting. Finally, an integrated gate recurrent unit model is constructed to map the relationship between condition monitoring data and RUL. A benchmark-bearing case study, whose results indicate that the approach can update fundamental model with the acquisition of new degradation cases, demonstrates the effectiveness.
更多查看译文
关键词
Gate recurrent unit (GRU),incremental learning,orthogonal weight modification (OWM),projector,remaining useful life (RUL) prediction
AI 理解论文
溯源树
样例
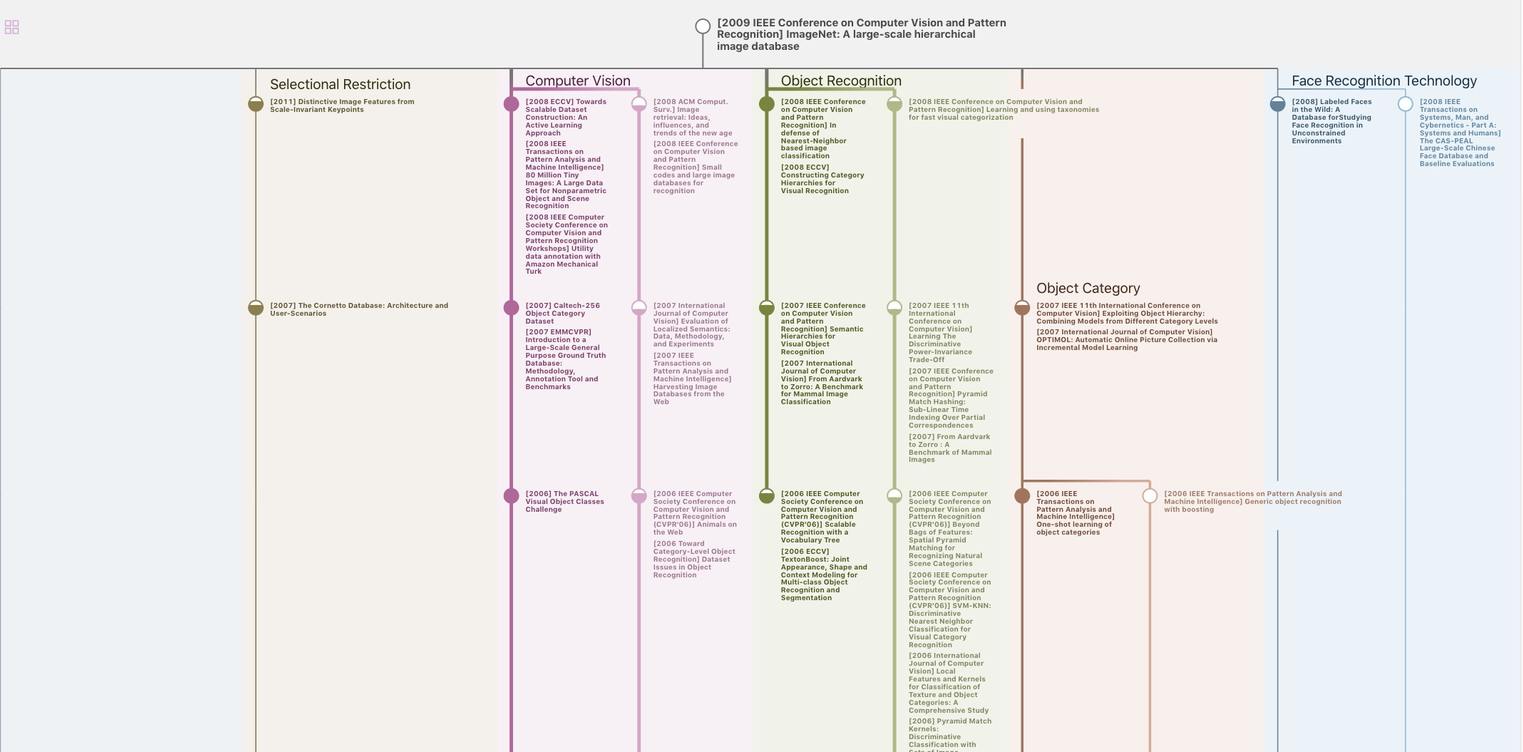
生成溯源树,研究论文发展脉络
Chat Paper
正在生成论文摘要