Predicting and explaining karst spring dissolved oxygen using interpretable deep learning approach
HYDROLOGICAL PROCESSES(2023)
摘要
Dissolved oxygen (DO) is one of the most important indicators of water quality and an essential measure for the aquatic organisms and the local ecosystem. DO concentrations in karst spring flow involves complex hydrological processes because of the heterogeneous nature of the karst system. In this study, an interpretable and explainable model that integrates the shapley additive explanations (SHAP) algorithm with the long short-term memory network model (LSTM) is proposed to evaluate the contributions of karst spring discharge, precipitation, water temperature, and specific conductance to DO concentrations in karst spring flow. The hybrid model can predict the temporal fluctuations of DO levels and provide a robust characterization of DO behaviours. To demonstrate the applicability of the proposed model, we adopted the hydrological and meteorological data from Barton Springs, situated within a highly karstified segment of the Edwards aquifer characterized by extensive fractures and openings. The optimal prediction performance is achieved with a 14-day time step, which is considered as the effective response time between various hydrological processes and DO concentrations at Barton Springs. It reveals that the influence of karst spring discharge, precipitation, water temperature, and specific conductance in previous 14 days collectively contribute to the current DO concentration in karst spring flow. The SHAP values of input features provide both local and global explanations, demonstrating the magnitude and the direction of each feature's impact on DO levels in karst spring flow. In descending order, the contributions of various hydrological processes to DO are ranked as follows: precipitation, karst spring discharge, temperature, and specific conductance. Precipitation and discharge exhibit positive SHAP values, indicating that increases in these hydrological processes contribute to higher DO levels in the karst flow. Water temperature and specific conductance have negative SHAP values, suggesting that higher water temperature and specific conductance will lead to decreased DO levels in the karst flow.
更多查看译文
关键词
deep learning,karst spring
AI 理解论文
溯源树
样例
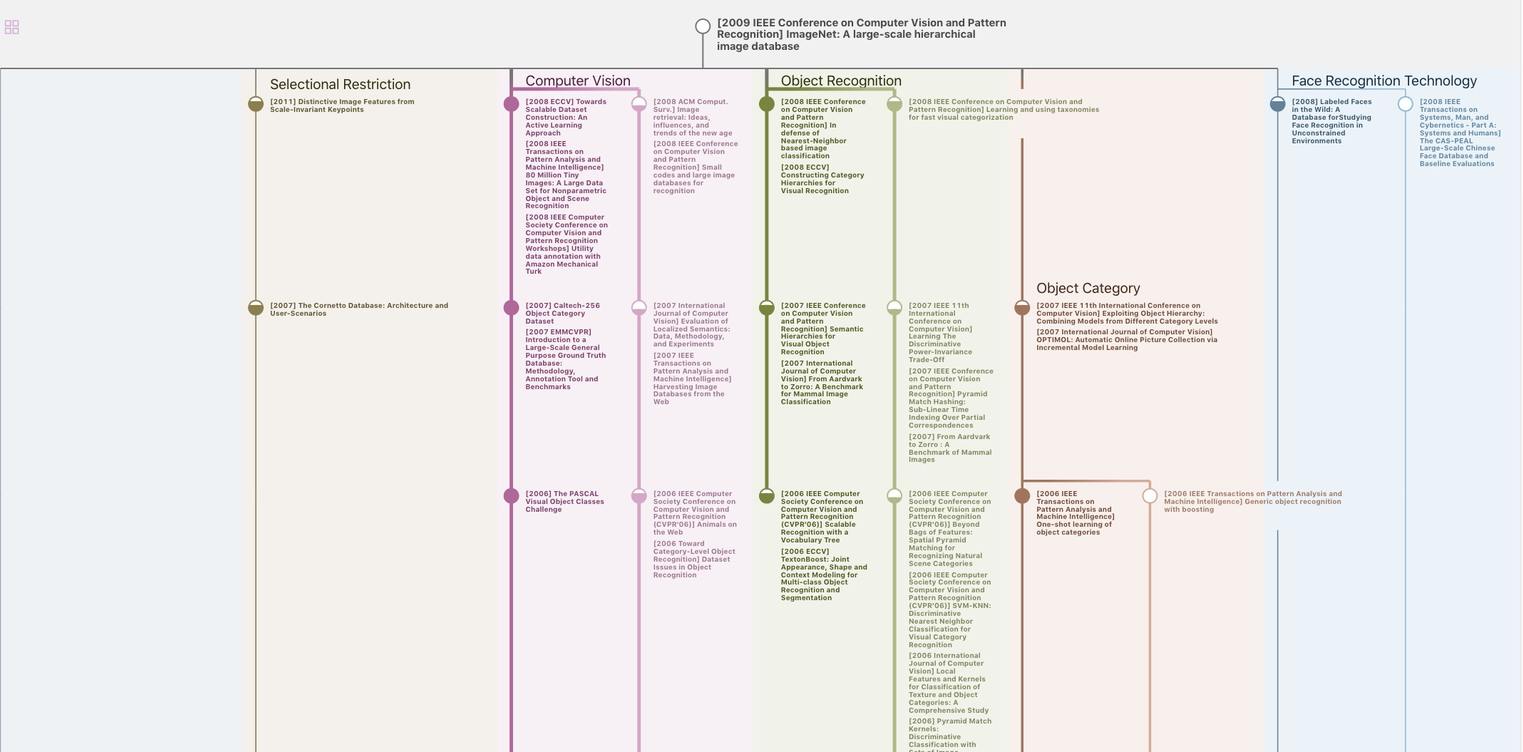
生成溯源树,研究论文发展脉络
Chat Paper
正在生成论文摘要