A hybrid ensemble-based automated deep learning approach to generate 3D geo-models and uncertainty analysis
ENGINEERING WITH COMPUTERS(2023)
摘要
There is an increasing interest in creating high-resolution 3D subsurface geo-models using multisource retrieved data, i.e., borehole, geophysical techniques, geological maps, and rock properties, for emergency managements. However, dedicating meaningful, and thus interpretable 3D subsurface views from such integrated heterogeneous data requires developing a new methodology for convenient post-modeling analyses. To this end, in the current paper a hybrid ensemble-based automated deep learning approach for 3D modeling of subsurface geological bedrock using multisource data is proposed. The uncertainty then was quantified using a novel ensemble randomly automated deactivating process implanted on the jointed weight database. The applicability of the automated process in capturing the optimum topology is then validated by creating 3D subsurface geo-model using laser-scanned bedrock-level data from Sweden. In comparison with intelligent quantile regression and traditional geostatistical interpolation algorithms, the proposed hybrid approach showed higher accuracy for visualizing and post-analyzing the 3D subsurface model. Due to the use of integrated multi-source data, the approach presented here and the subsequently created 3D model can be a representative reconcile for geoengineering applications.
更多查看译文
关键词
3D subsurface geo-model,Hybrid ensemble deep learning,Automated process,Uncertainty quantification,Sweden
AI 理解论文
溯源树
样例
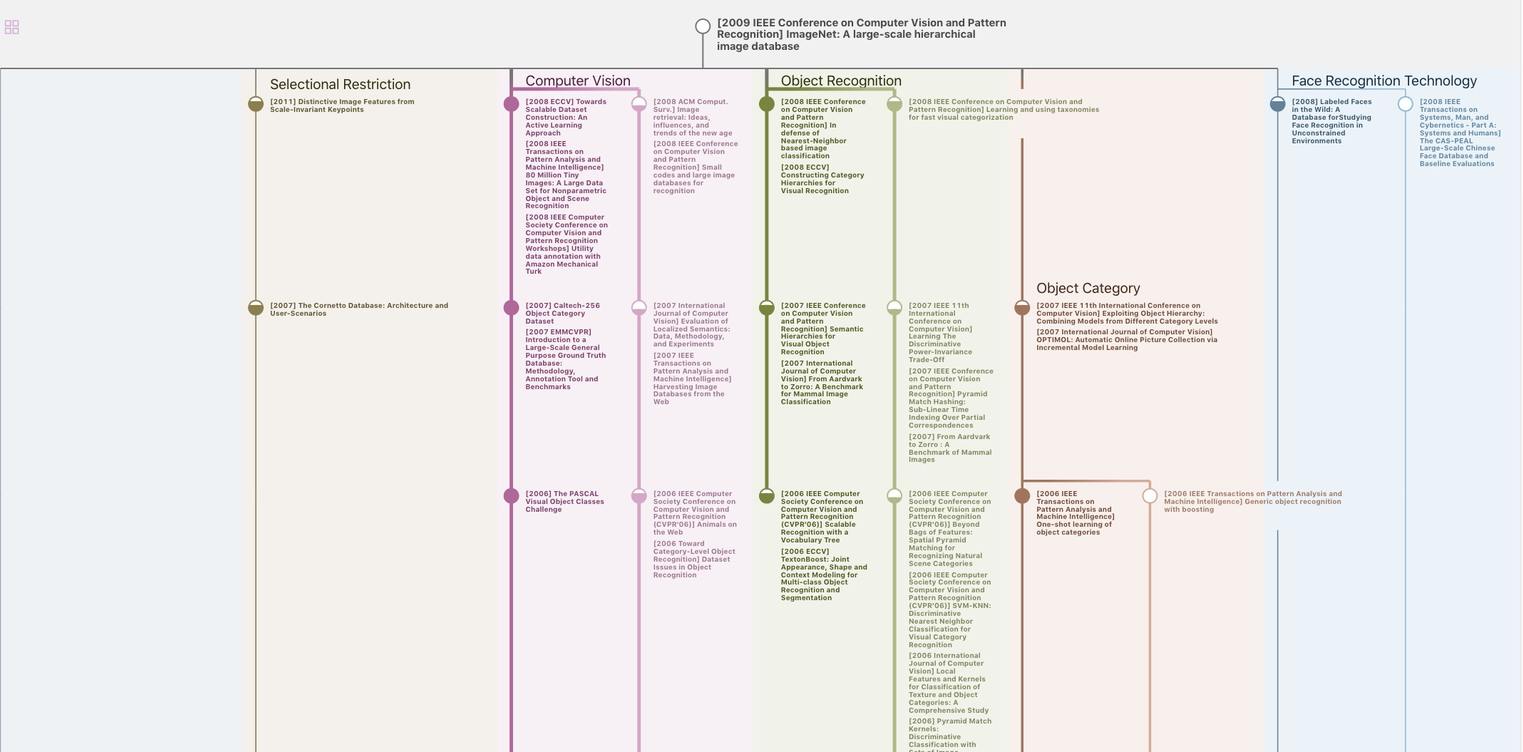
生成溯源树,研究论文发展脉络
Chat Paper
正在生成论文摘要