Predicting soil organic carbon in cultivated land across geographical and spatial scales: Integrating Sentinel-2A and laboratory Vis-NIR spectra
ISPRS JOURNAL OF PHOTOGRAMMETRY AND REMOTE SENSING(2023)
摘要
Digital mapping of soil organic carbon (SOC) is essential for visualizing the spatial distribution at different re-gions and scales. However, existing studies using remote sensing are limited by the low spectral resolution of multispectral data for accurate estimation of SOC, and the failure of laboratory visible and near-infrared and shortwave infrared (Vis-NIR) spectroscopy to perform pixel-level mapping. To address these limitations, this study proposes a SOC mapping framework that integrates satellite earth observations and proximal sensing spectral data to reconstruct images having a spectral resolution of 400-2500 nm and a spatial resolution of 10 m, then the clustering probability analysis was applied on the reconstructed images, to develop a high accuracy SOC prediction model and demonstrate its effectiveness across geographical and spatial scales. Specifically, a total of 324 topsoil samples were collected from Baoqing and Suiling counties in northeast China, whose laboratory Vis-NIR reflectance spectra and SOC were measured. Sentinel-2A images of bare soils averaged over three years before and after the samples collection (i.e., 2018 to 2021) were collected. A total of 264 samples from eight fields in Belgium were used to evaluate the performance of the model at the field scale. Then, two models were developed (i) a global regression model with direct prediction using random forest and (ii) a clustering proba-bility model with Gaussian Mixture Model-random forest. Results demonstrated that (1) reconstructed images produced more accurate prediction results than the individual laboratory Vis-NIR spectroscopy and Sentinel-2A models. (2) At the regional scale, clustering probability model achieved better performance in quantification of SOC (coefficient of determination [R2] = 0.78, and root mean square error [RMSE] = 0.78 %) than global regression model (R2 = 0.65, and RMSE = 1.06 %). (3) For field scale, the reconstructed image-based clustering probability SOC prediction model was shown to be effective when using the Belgium data, providing a R2 of 0.67, and RMSE of 0.15 %. (4) Integrating remote sensing multispectral and proximal Vis-NIR information can lead to a smoother and more continuous SOC distribution within the region, and can better show its spatial heteroge-neity within the field. This study highlights the advantages of integrating remote sensing with proximal sensing for improving the accuracy of SOC prediction and mapping, enabling applicability of the model across geographical and spatial scales with appreciable accuracy.
更多查看译文
关键词
Clustering probability model,Sentinel-2A,laboratory Vis-NIR spectral,Integrate,Multi-scales,Random forest,Digital SOC mapping
AI 理解论文
溯源树
样例
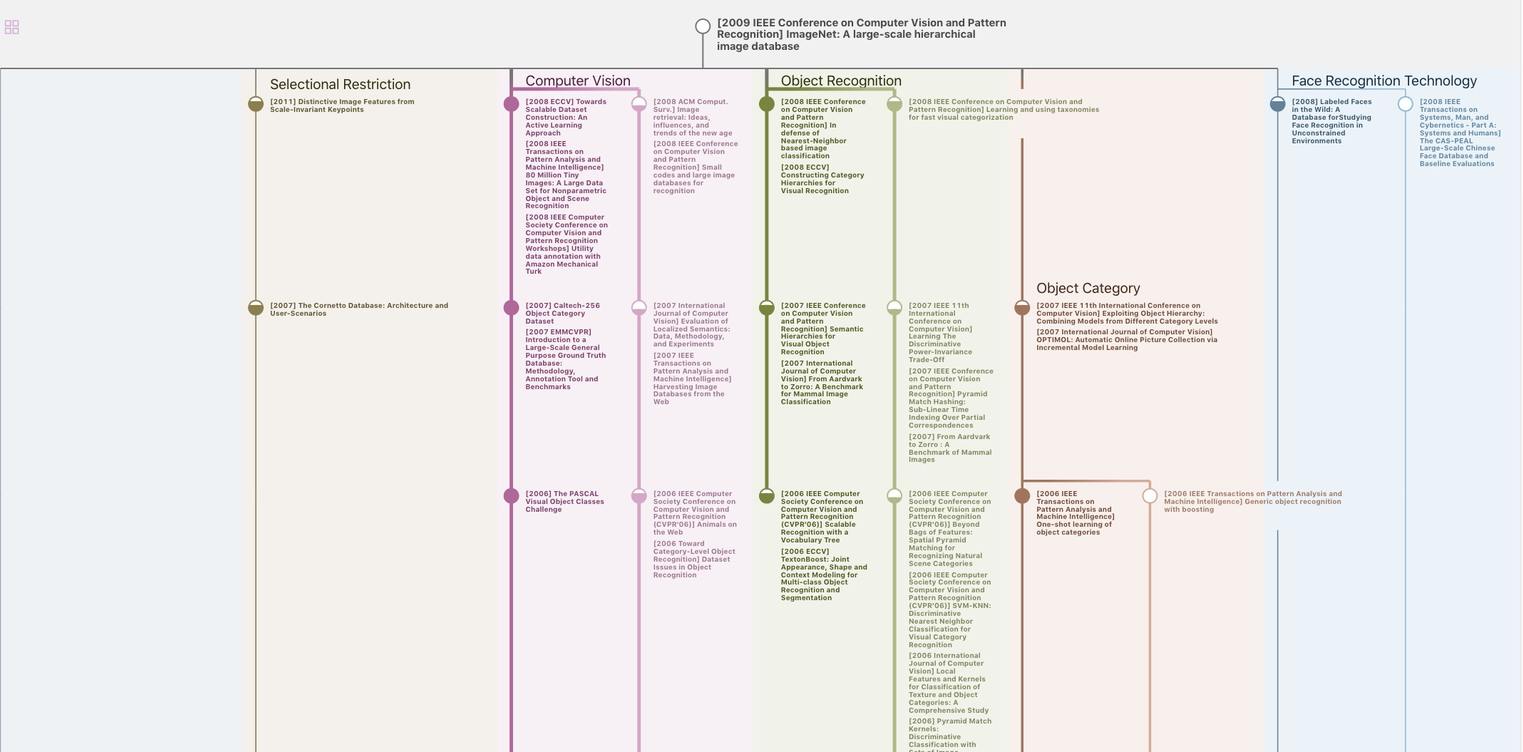
生成溯源树,研究论文发展脉络
Chat Paper
正在生成论文摘要