Latent Topic-Aware Multioutput Learning
IEEE TRANSACTIONS ON SYSTEMS MAN CYBERNETICS-SYSTEMS(2023)
摘要
Multioutput learning targeting predicting multiple outputs for each input has attracted increasing attention due to its ability to handle diverse data types in outputs. Most feature extraction-based methods for tackling multioutput problems neglect the local correlation between different outputs, while most sample extraction-based methods overlook the gap between inputs and outputs. To overcome these two major limitations, in this article, we propose a topic-aware method where we assume inputs and outputs can be jointly embedded in a topic space. Both feature and sample extraction are performed in a latent topic space that encodes output correlation at a topic level and aligns inputs and outputs in an explainable manner. Different from current topic models, we extract independent and interdependent topics based on informative topics. These two components are verified as useful in performance improvement in an ablation study. The proposed method can also be easily extended and applied in multiview learning. The experimental results of the proposed topic-aware model on multiple benchmarks for different multioutput tasks in single-/multi-views with rho-values less than 0.05 indicate that the improvement achieved by the proposed method over compared baselines is statistically significant.
更多查看译文
关键词
learning,topic-aware
AI 理解论文
溯源树
样例
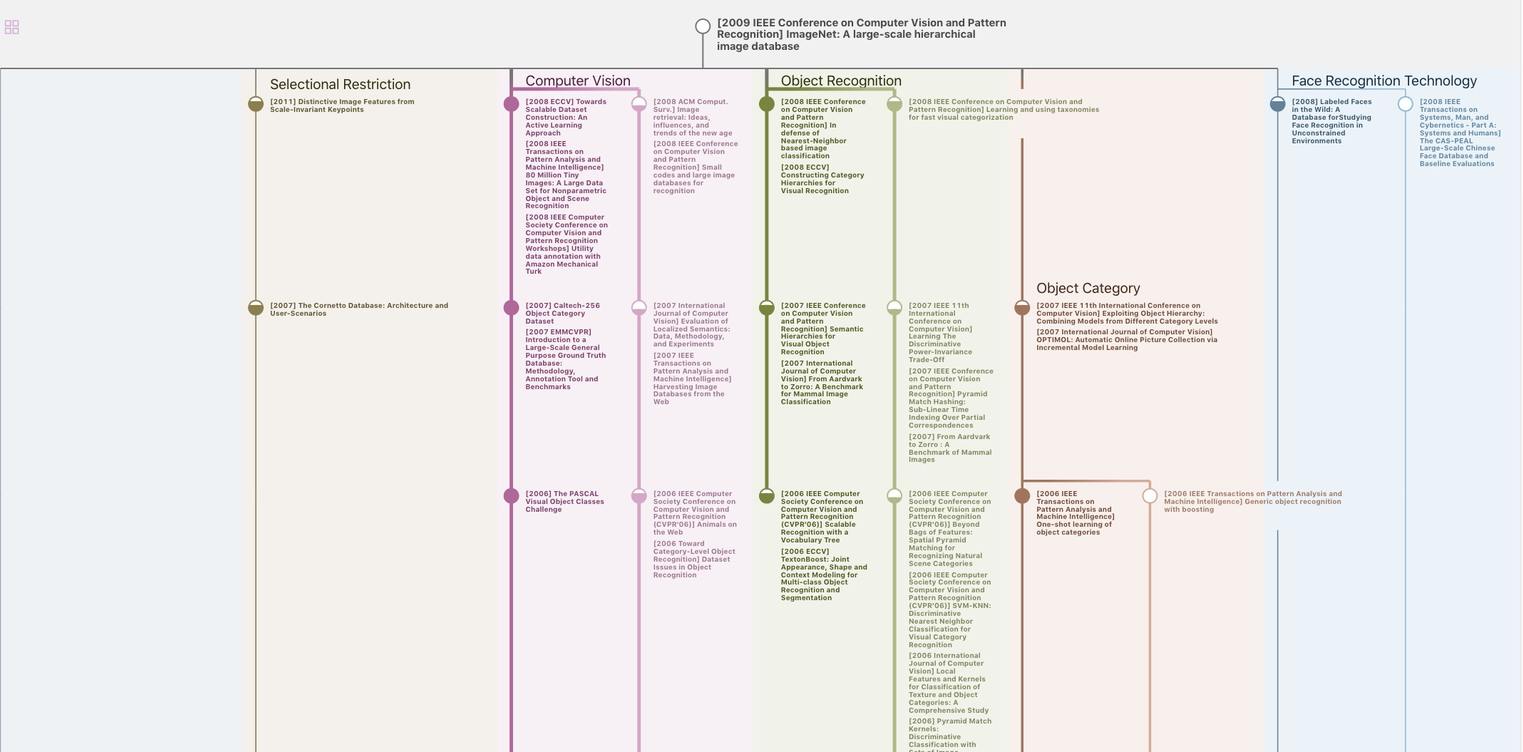
生成溯源树,研究论文发展脉络
Chat Paper
正在生成论文摘要