On sub-gaussian concentration of missing mass*
THEORY OF PROBABILITY AND ITS APPLICATIONS(2023)
摘要
The statistical inference on missing mass aims to estimate the weight of elements not observed during sampling. Since the pioneer work of Good and Turing, the problem has been studied in many areas, including statistical linguistics, ecology, and machine learning. Proving the sub-Gaussian behavior of the missing mass has been notoriously hard, and a number of complicated arguments have been proposed: logarithmic Sobolev inequalities, thermodynamic approaches, and information-theoretic transportation methods. Prior works have argued that the difficulty is inherent, and classical tools are inadequate. We show that this common belief is false, and all that we need to establish the sub-Gaussian concentration is the classical inequality of Bernstein. The strong educational value of our work is in its demonstration of this inequality in its full generality, an aspect not well recognized by researchers.
更多查看译文
关键词
concentration,sub-gaussian
AI 理解论文
溯源树
样例
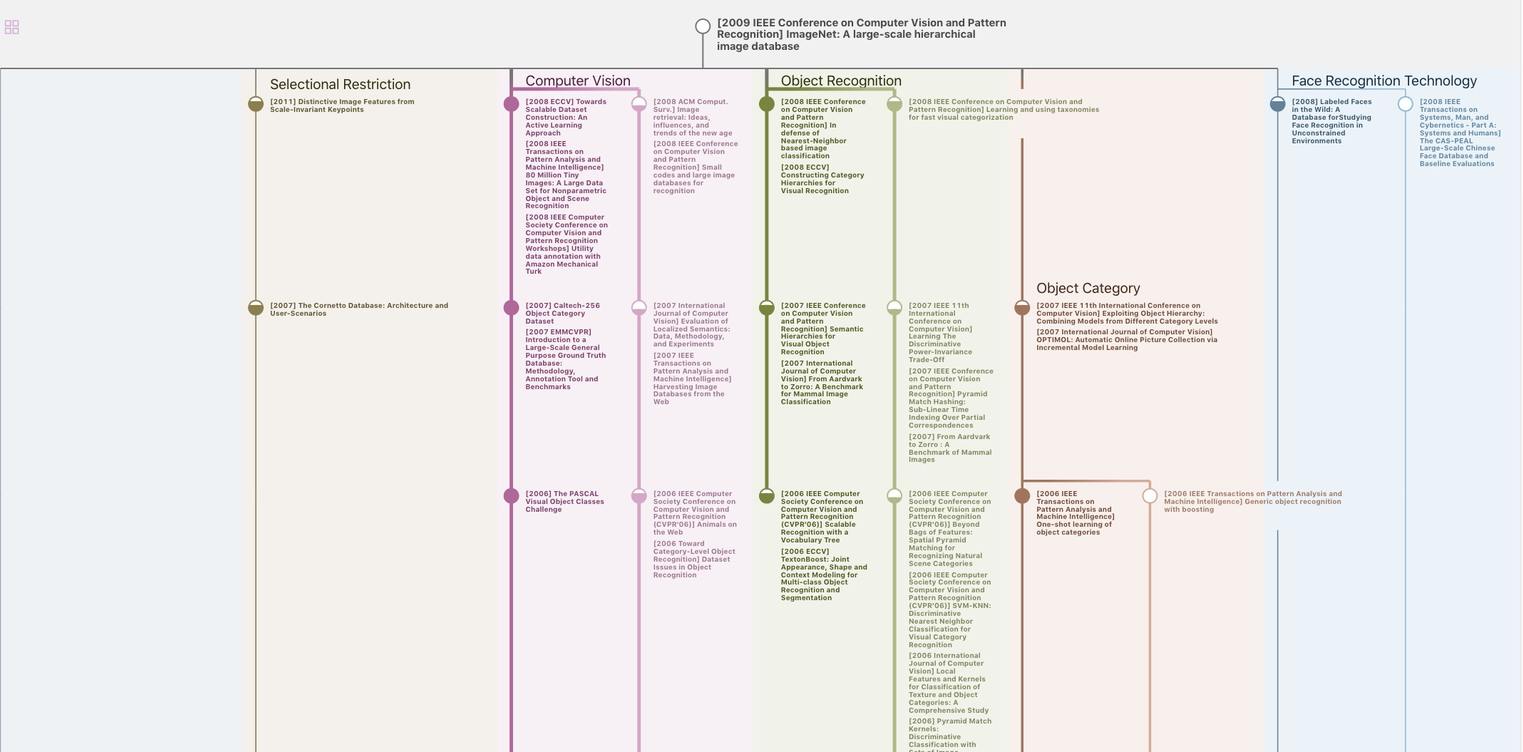
生成溯源树,研究论文发展脉络
Chat Paper
正在生成论文摘要