Towards Automatic Annotation and Detection of Fake News.
IEEE Conference on Local Computer Networks(2023)
摘要
Automated accounts or bots on Online Social Networks (OSNs) play a significant role in disseminating information, including false news, which may instigate cyber propaganda. The existing research on fake news detection does not account for the existence of bots. Also, they only focus on identifying fake news in “the articles shared in posts” rather than the post’s (textual) content and use manually labeled limited datasets. In this research, we overcome the challenge of data scarcity by proposing an automated approach for labeling data using verified fact-checked statements on OSNs such as Twitter. Moreover, we analyze the presence and impact of bots and show that bots change their behavior over time. Our experiments focus on COVID-19, collect 10.22 million COVID-19-re1ated tweets, and use our annotation model to build an extensive ground truth dataset for classification purposes. We evaluated our automatic annotation model on two existing COVID-19-re1ated misinformation datasets and achieved a ~ 2% increase in precision compared to the existing annotation models. In addition, our best classification model achieves 83% precision, 96% recall, and a ~ 4% false positive rate on our annotated dataset, outperforming existing techniques.
更多查看译文
AI 理解论文
溯源树
样例
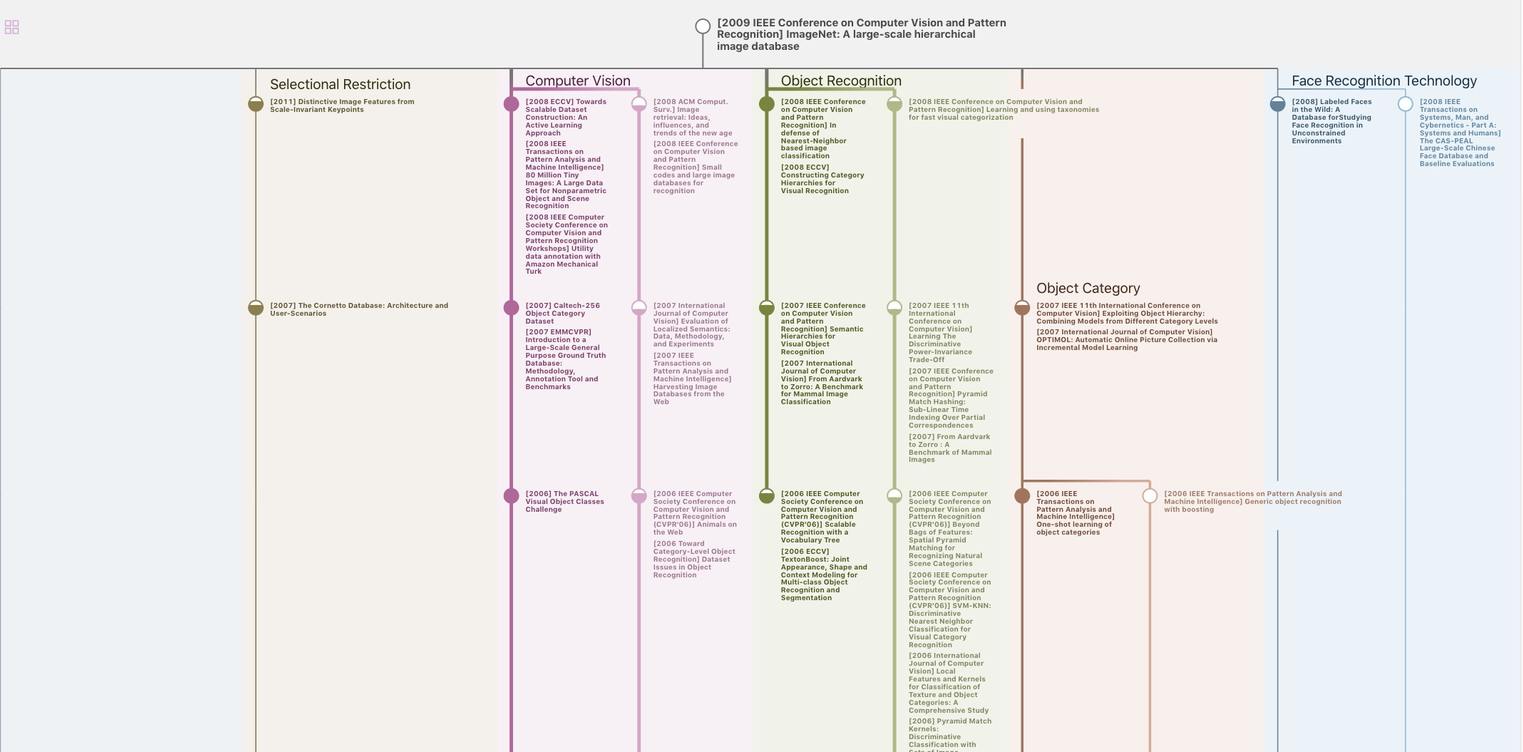
生成溯源树,研究论文发展脉络
Chat Paper
正在生成论文摘要