SADIR: Shape-Aware Diffusion Models for 3D Image Reconstruction
SHAPE IN MEDICAL IMAGING, SHAPEMI 2023(2023)
Key words
Texture Analysis,Image Segmentation,Statistical Shape Models,Deformable Image Registration,Diffusion MRI
AI Read Science
Must-Reading Tree
Example
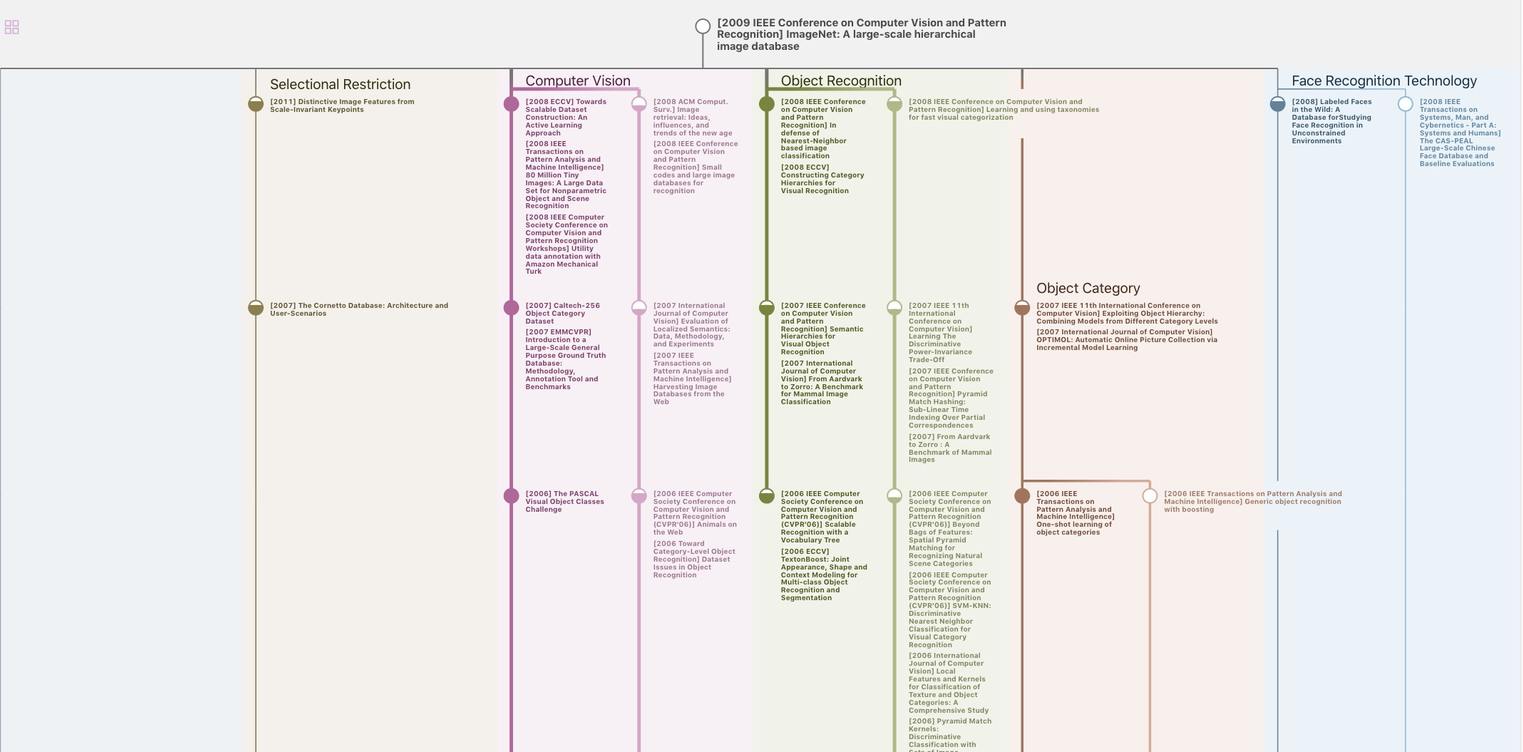
Generate MRT to find the research sequence of this paper
Chat Paper
Summary is being generated by the instructions you defined