Compact Representation of n-th order TGV
arXiv (Cornell University)(2023)
摘要
Although regularization methods based on derivatives are favored for their robustness and computational simplicity, research exploring higher-order derivatives remains limited. This scarcity can possibly be attributed to the appearance of oscillations in reconstructions when directly generalizing TV-1 to higher orders (3 or more). Addressing this, Bredies et. al introduced a notable approach for generalizing total variation, known as Total Generalized Variation (TGV). This technique introduces a regularization that generates estimates embodying piece-wise polynomial behavior of varying degrees across distinct regions of an image.Importantly, to our current understanding, no sufficiently general algorithm exists for solving TGV regularization for orders beyond 2. This is likely because of two problems: firstly, the problem is complex as TGV regularization is defined as a minimization problem with non-trivial constraints, and secondly, TGV is represented in terms of tensor-fields which is difficult to implement. In this work we tackle the first challenge by giving two simple and implementable representations of n th order TGV
更多查看译文
关键词
representation
AI 理解论文
溯源树
样例
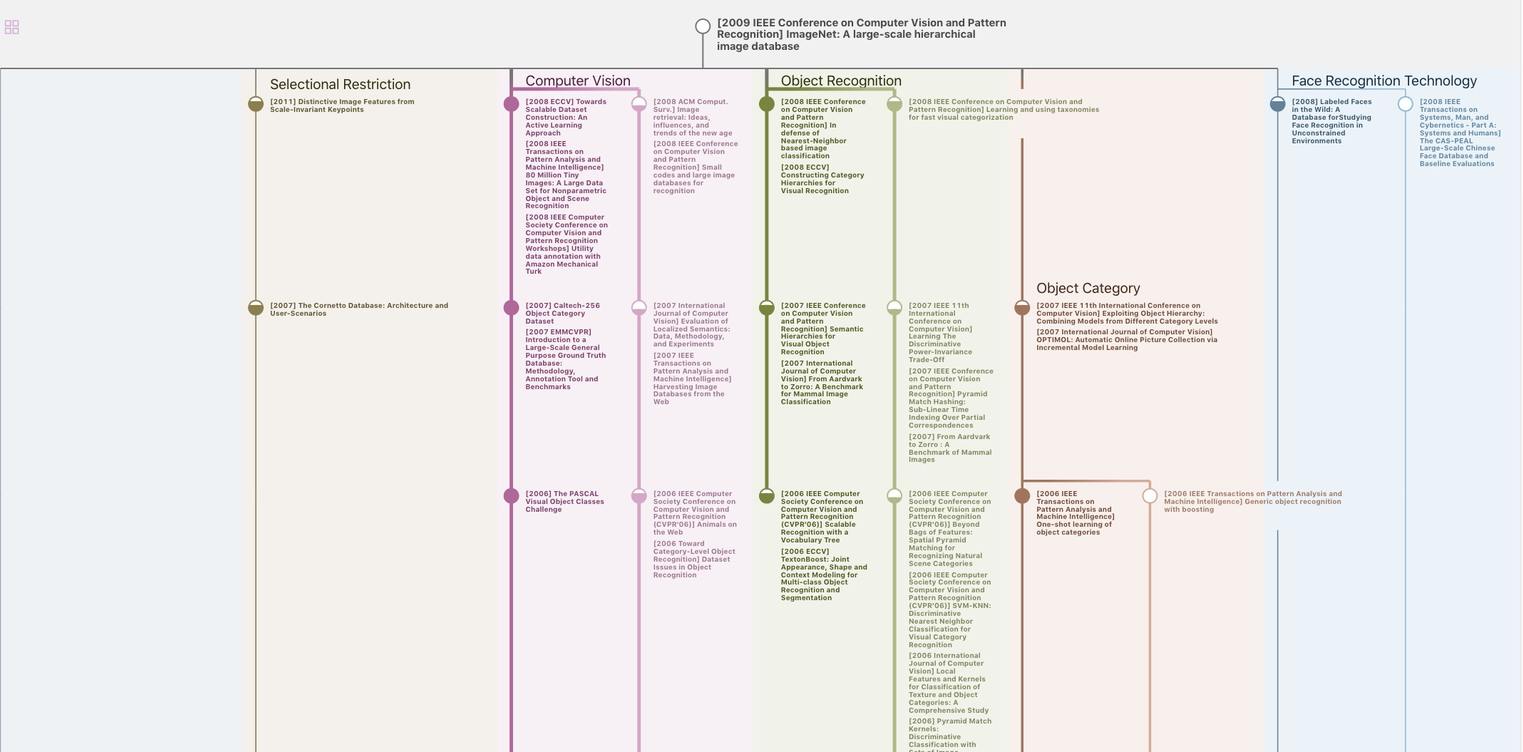
生成溯源树,研究论文发展脉络
Chat Paper
正在生成论文摘要