A State Representation for Diminishing Rewards
NeurIPS(2023)
摘要
A common setting in multitask reinforcement learning (RL) demands that an agent rapidly adapt to various stationary reward functions randomly sampled from a fixed distribution. In such situations, the successor representation (SR) is a popular framework which supports rapid policy evaluation by decoupling a policy's expected discounted, cumulative state occupancies from a specific reward function. However, in the natural world, sequential tasks are rarely independent, and instead reflect shifting priorities based on the availability and subjective perception of rewarding stimuli. Reflecting this disjunction, in this paper we study the phenomenon of diminishing marginal utility and introduce a novel state representation, the $\lambda$ representation ($\lambda$R) which, surprisingly, is required for policy evaluation in this setting and which generalizes the SR as well as several other state representations from the literature. We establish the $\lambda$R's formal properties and examine its normative advantages in the context of machine learning, as well as its usefulness for studying natural behaviors, particularly foraging.
更多查看译文
关键词
state representation
AI 理解论文
溯源树
样例
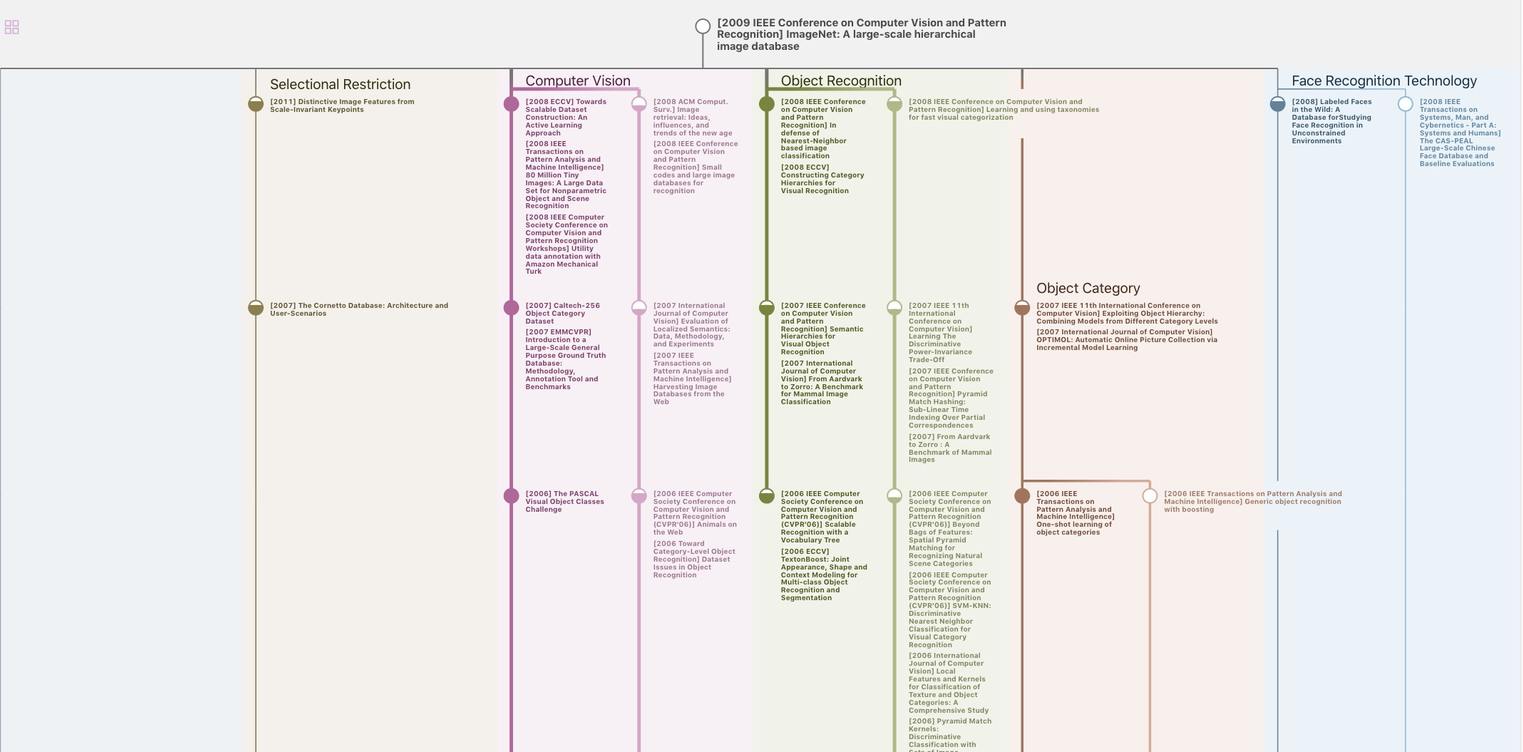
生成溯源树,研究论文发展脉络
Chat Paper
正在生成论文摘要