Efficient estimation and correction of selection-induced bias with order statistics
arXiv (Cornell University)(2023)
摘要
Model selection aims to identify a sufficiently well performing model that is possibly simpler than the most complex model among a pool of candidates. However, the decision-making process itself can inadvertently introduce non-negligible bias when the cross-validation estimates of predictive performance are marred by excessive noise. In finite data regimes, cross-validated estimates can encourage the statistician to select one model over another when it is not actually better for future data. While this bias remains negligible in the case of few models, when the pool of candidates grows, and model selection decisions are compounded (as in forward search), the expected magnitude of selection-induced bias is likely to grow too. This paper introduces an efficient approach to estimate and correct selection-induced bias based on order statistics. Numerical experiments demonstrate the reliability of our approach in both estimating selection-induced bias and quantifying the degree of over-fitting along compounded model selection decisions, with specific application to forward search. This work represents a light-weight alternative to more computationally expensive approaches to correcting selection-induced bias, such as nested cross-validation and the bootstrap. Our approach rests on several theoretic assumptions, and we provide a diagnostic to help understand when these may not be valid, and when to fall back on safer, albeit more computationally expensive approaches. The accompanying code facilitates its practical implementation and fosters further exploration in this area.
更多查看译文
关键词
efficient estimation,bias,statistics,selection-induced
AI 理解论文
溯源树
样例
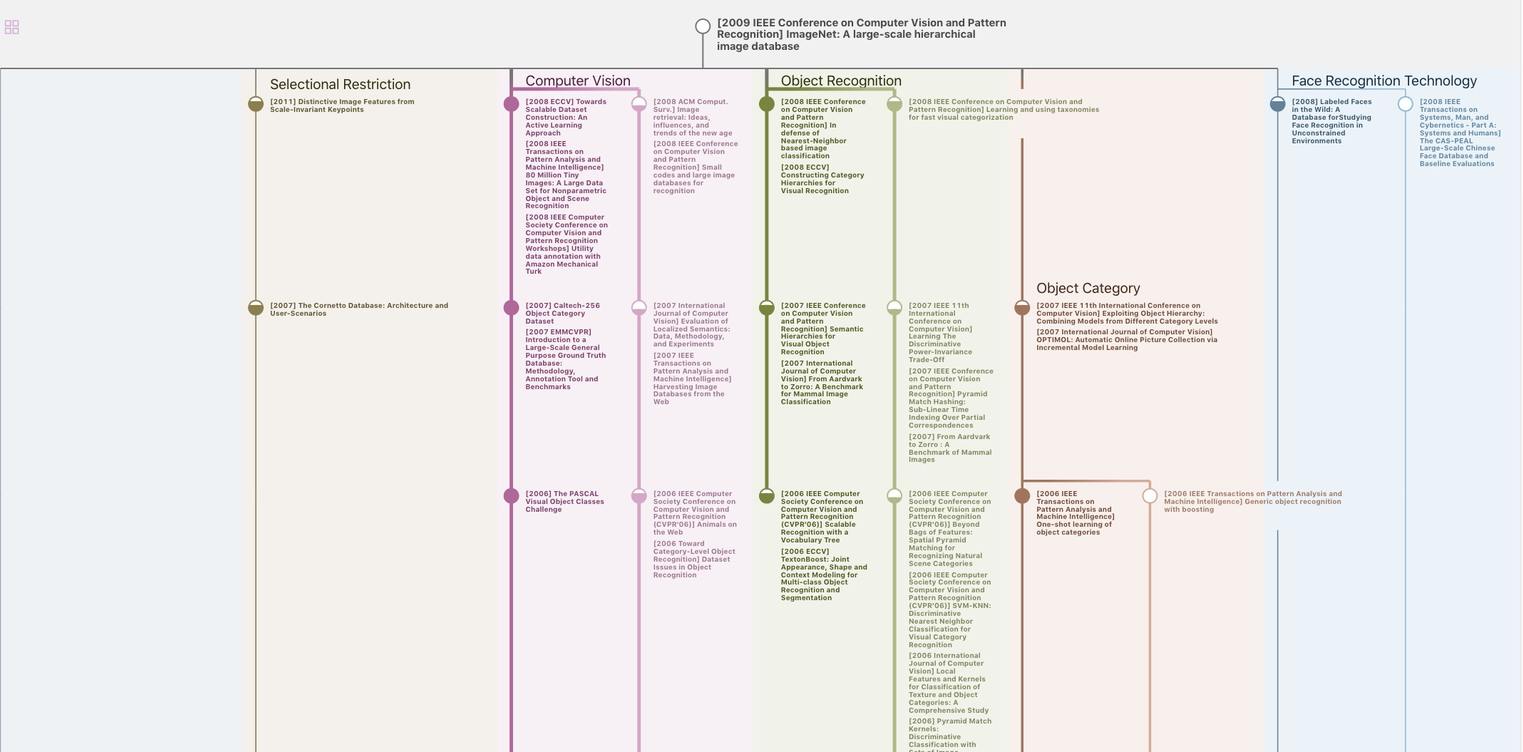
生成溯源树,研究论文发展脉络
Chat Paper
正在生成论文摘要