Neural Networks for Portfolio Analysis With Cardinality Constraints
IEEE transactions on neural networks and learning systems(2023)
摘要
Portfolio analysis is a crucial subject within modern finance. However, the classical Markowitz model, which was awarded the Nobel Prize in Economics in 1991, faces new challenges in contemporary financial environments. Specifically, it fails to consider transaction costs and cardinality constraints, which have become increasingly critical factors, particularly in the era of high-frequency trading. To address these limitations, this research is motivated by the successful application of machine learning tools in various engineering disciplines. In this work, three novel dynamic neural networks are proposed to tackle nonconvex portfolio optimization under the presence of transaction costs and cardinality constraints. The neural dynamics are intentionally designed to exploit the structural characteristics of the problem, and the proposed models are rigorously proven to achieve global convergence. To validate their effectiveness, experimental analysis is conducted using real stock market data of companies listed in the Dow Jones Index (DJI), covering the period from November 8, 2021 to November 8, 2022, encompassing an entire year. The results demonstrate the efficacy of the proposed methods. Notably, the proposed model achieves a substantial reduction in costs (which combines investment risk and reward) by as much as 56.71% compared with portfolios that are averagely selected.
更多查看译文
关键词
Cardinality constraint,neural dynamics,neural networks,nonconvex optimization,portfolio analysis
AI 理解论文
溯源树
样例
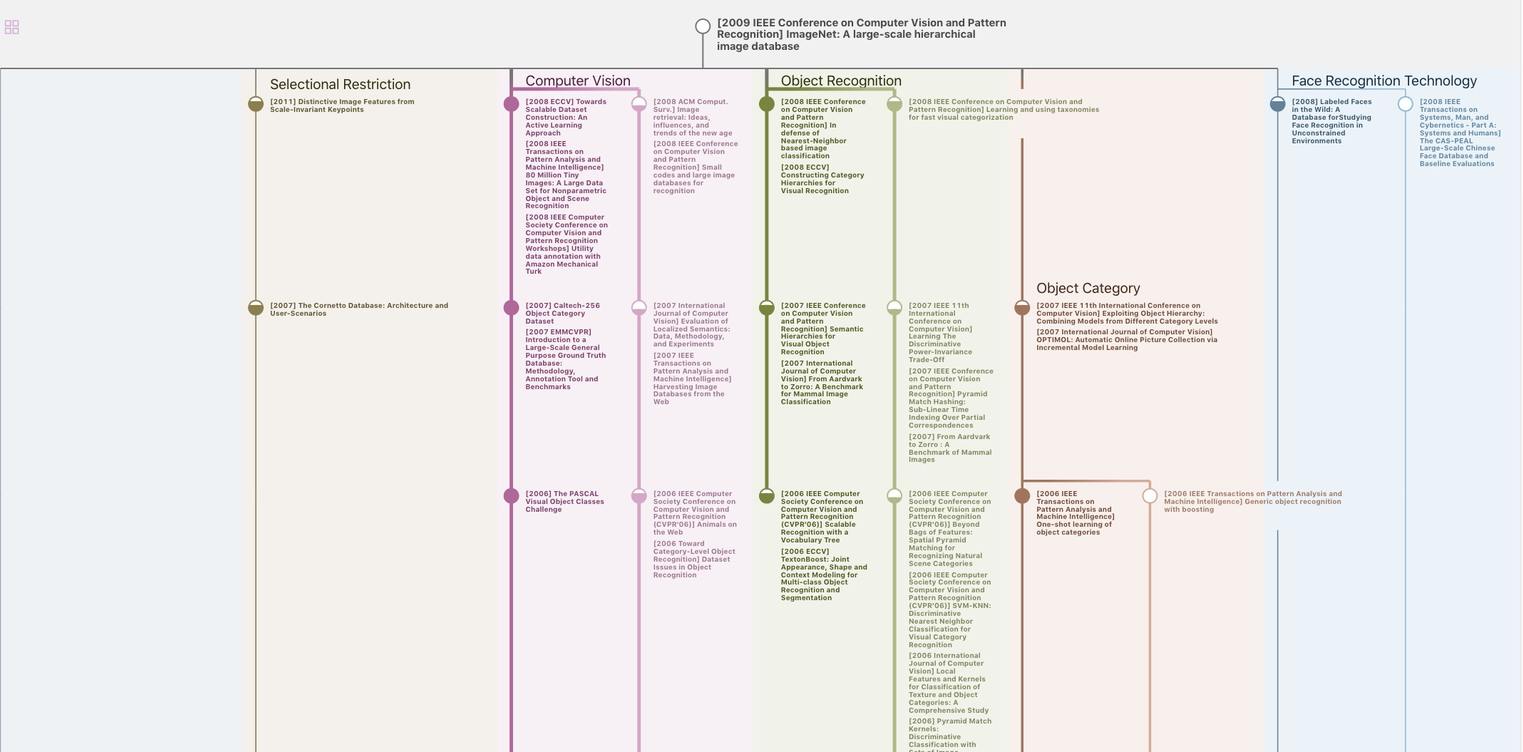
生成溯源树,研究论文发展脉络
Chat Paper
正在生成论文摘要