Word Self-Update Contrastive Adversarial Networks for Text-to-image Synthesis.
Neural Networks(2023)
摘要
Synthesizing realistic fine-grained images from text descriptions is a significant computer vision task. Although many GANs-based methods have been proposed to solve this task, generating high-quality images consistent with text information remains a difficult problem. These existing GANs-based methods ignore important words due to the use of fixed initial word features in generator, and neglect to learn semantic consistency between images and texts for discriminators. In this article, we propose a novel attentional generation and contrastive adversarial framework for fine-grained text-to-image synthesis, termed as Word Self-Update Contrastive Adversarial Networks (WSC-GAN). Specifically, we introduce a dual attention module for modeling color details and semantic information. With a new designed word self-update module, the generator can leverage visually important words to compute attention maps in the feature synthesis module. Furthermore, we contrive multi-branch contrastive discriminators to maintain better consistency between the generated image and text description. Two novel contrastive losses are proposed for our discriminators to impose image-sentence and image-word consistency constraints. Extensive experiments on CUB and MS-COCO datasets demonstrate that our method achieves better performance compared with state-of-the-art methods.
更多查看译文
关键词
Text-to-image synthesis,Contrastive learning,Word self-attention,Dual attention generator
AI 理解论文
溯源树
样例
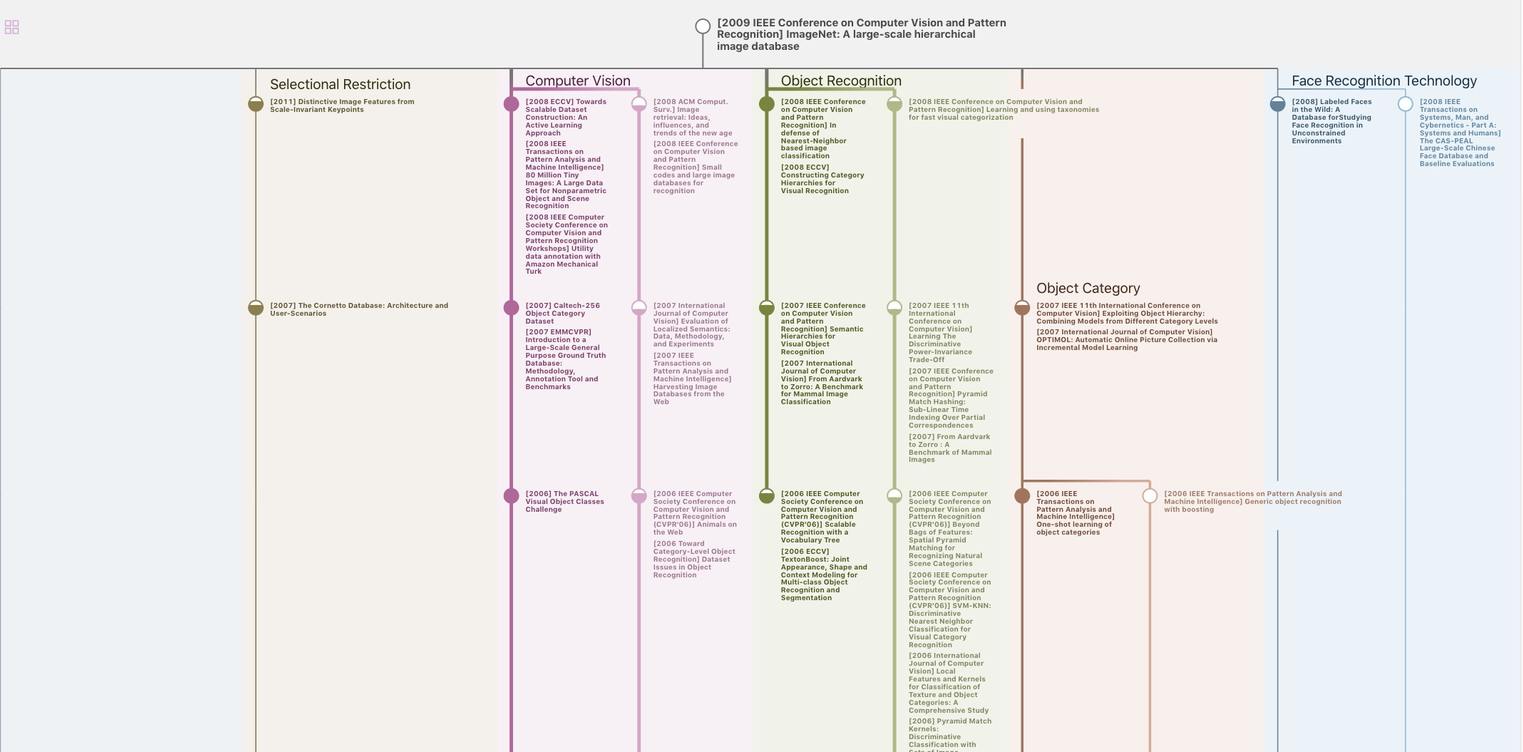
生成溯源树,研究论文发展脉络
Chat Paper
正在生成论文摘要