Recurrent Neural Network-based Prediction of O-GlcNAcylation Sites in Mammalian Proteins
bioRxiv (Cold Spring Harbor Laboratory)(2023)
摘要
Glycosylation can modify proteins in positive and essential ways, such as ensuring correct protein folding or increasing the potency of antibodies, or in negative ways, such as promoting cancers. In particular, O-GlcNAcylation has the potential to be an important target for therapeutics, but O-GlcNAcylation sites are not known. In spite of the importance of O-GlcNAcylation, current predictive models are insufficient as they fail to generalize, and many are no longer available. This article constructs MLP and RNN models to predict the presence of O-GlcNAcylation sites based on protein sequences. Multiple different datasets are evaluated separately and assessed in terms of strengths and issues. The models trained in this work achieve considerably better metrics than previously published models, with at least a two-fold increase in F1 score relative to previously published models; the specific gains vary depending on the dataset. The best model achieves an F1 score of 36.17%, multiple times greater than any previously published model and 7.6 times higher than when not using any model. We release all of the software used in this work on our GitHub ([github.com/PedroSeber/O-GlcNAcylation_Prediction][1]), allowing the reproduction of this article and facilitating future studies in the prediction of O-GlcNAcylation sites.
Author Summary Glycosylation is a reaction in which a sugar is attached to a functional group of another molecule. This post-translational modification plays a critical role in determining protein structure, function, and stability. On the other hand, improper glycosylation or deglycosylation is associated with many diseases. O-GlcNAcylation is a type of glycosylation in which N-Acetylglucosamine is the first glycan added to an oxygen atom of a protein. A greater understanding of glycosylation could improve treatments, through drugs with novel modes of action or by improving existing therapeutics. In particular, there are no methods for predicting in advance which oxygen sites will be O-GlcNAcylated. Past studies have attempted to predict the presence of O-GlcNAcylation sites using AI models but the associated models have had poor predictive performance. We construct recurrent neural network-based models that improve predictive performance by more than a factor of 7. The software is released as open source, so that researchers can use the software to support their efforts in drug discovery, design, and manufacturing.
### Competing Interest Statement
The authors have declared no competing interest.
[1]: http://github.com/PedroSeber/O-GlcNAcylation_Prediction
更多查看译文
关键词
mammalian proteins,network-based,o-glcnacylation
AI 理解论文
溯源树
样例
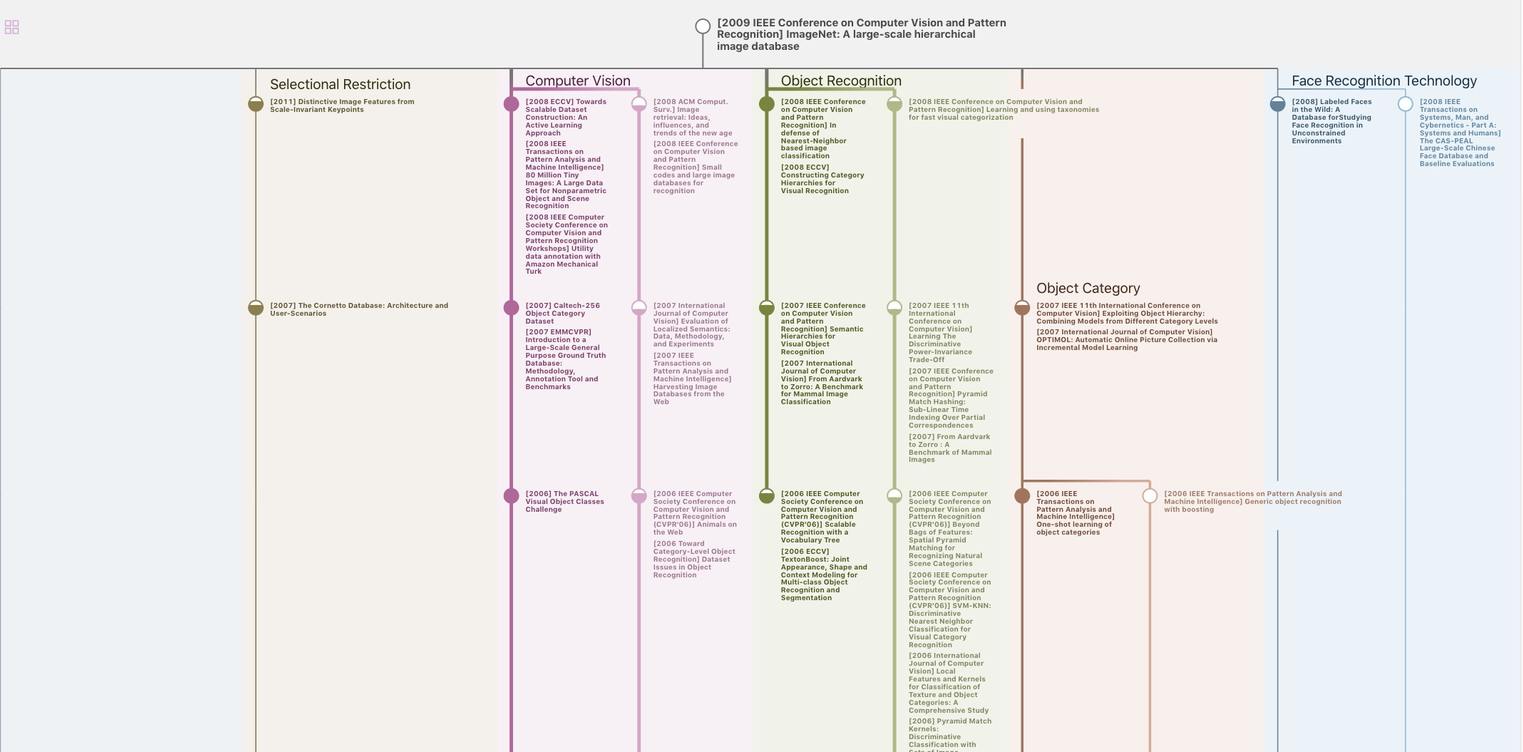
生成溯源树,研究论文发展脉络
Chat Paper
正在生成论文摘要