Enhancing Dissolved Oxygen Concentrations Prediction in Water Bodies: A Temporal Transformer Approach with Multi-Site Meteorological Data Graph Embedding
Water(2023)
摘要
Water ecosystems are highly sensitive to environmental conditions, including meteorological factors, which influence dissolved oxygen (DO) concentrations, a critical indicator of water quality. However, the complex relationships between multiple meteorological factors from various sites and DO concentrations pose a significant challenge for accurate prediction. This study introduces an innovative framework for enhancing DO concentration predictions in water bodies by integrating multi-station meteorological data. We first construct a dynamic meteorological graph with station-specific factors as node features and geographic distances as edge weights. This graph is processed using a Geo-Contextual Graph Embedding Module, leveraging a Graph Convolutional Network (GCN) to distill geographical and meteorological features from multi-station data. Extracted features are encoded and then temporally merged with historical DO values to form time-series data. Finally, a Temporal Transformer module is used for future DO concentration predictions. The proposed model shows superior performance compared to traditional methods, successfully capturing the complex relationships between meteorological factors and DO levels. It provides an effective tool for environmental scientists and policymakers in water quality monitoring and management. This study suggests that the integration of graph-based learning and a Temporal Transformer in environmental modeling is a promising direction for future research.
更多查看译文
关键词
dissolved oxygen concentration prediction,multi-site meteorological data,graph convolutional networks,temporal transformer,environmental modeling,Tianjin,China
AI 理解论文
溯源树
样例
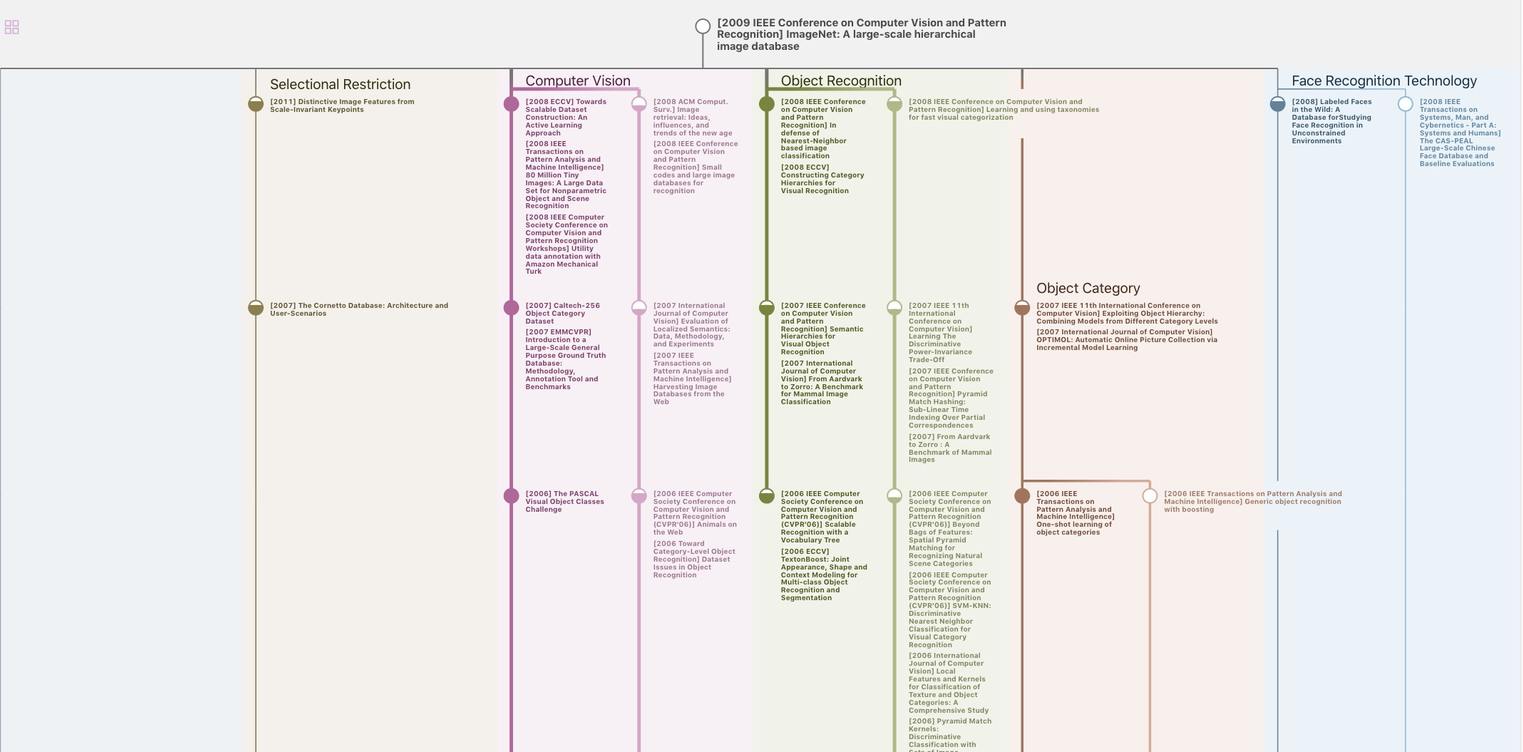
生成溯源树,研究论文发展脉络
Chat Paper
正在生成论文摘要