192. Machine learning to automatically generate billing codes for a variety of orthopedic surgery procedure operative notes: a study of 922 patients
The Spine Journal(2023)
摘要
BACKGROUND CONTEXT Generation of CPT codes from operative notes for billing purposes is a labor-intensive process prone to human error. Recent advances in natural language processing (NLP) machine learning algorithms have potential to enhance and introduce standardization in the process and could result in decreased healthcare costs for patients. PURPOSE Demonstrate that NLP is a powerful augmentation tool for billing in orthopedic surgery. STUDY DESIGN/SETTING Retrospective cohort study. PATIENT SAMPLE We collected 922 operative notes from patients who underwent elective ACDF, PCDF, or CDA from 2015 to 2020 and included CPT codes generated by the billing code department. OUTCOME MEASURES Area Under the Receiver Operating Curve (AUROC) scores were calculated for all trials and iterations of the model. This measures the true positive rate vs the false negative rate which approximates the predictive power of a machine learning model. METHODS We trained XLNet, a generalized autoregressive pretraining method, on this dataset and tested its performance in four procedural combinations using a receiver operating characteristic analysis and calculating the AUROC score. Heatmaps were generated to demonstrate the attention that the model was placing on each word in the operative note with a darker color representing greater attention. RESULTS The performance of the model approached human accuracy, with trial 1 (ACDF) achieving an AUROC score of .82 and a class-by-class accuracy of 77%; trial 2 (PCDF) achieving an AUROC score of .83 and a class-by-class accuracy of 71%; trial 3 (ACDF and CDA) achieving an AUROC score of .95 and a class-by-class accuracy of 87%; and trial 4 (ACDF, PCDF, and CDA) achieving a AUROC score of .95 and a class-by-class accuracy of 88%. In some cases, the model was able to generate CPT codes that the billing department had missed. CONCLUSIONS We show that the XLNet model can be successfully applied to orthopedic surgeon's operative notes to generate CPT billing codes. As NLP models as a whole continue to improve, billing can be greatly augmented with artificial intelligence assisted generation of CPT billing codes which will help minimize error and promote standardization in the process. FDA Device/Drug Status This abstract does not discuss or include any applicable devices or drugs. Generation of CPT codes from operative notes for billing purposes is a labor-intensive process prone to human error. Recent advances in natural language processing (NLP) machine learning algorithms have potential to enhance and introduce standardization in the process and could result in decreased healthcare costs for patients. Demonstrate that NLP is a powerful augmentation tool for billing in orthopedic surgery. Retrospective cohort study. We collected 922 operative notes from patients who underwent elective ACDF, PCDF, or CDA from 2015 to 2020 and included CPT codes generated by the billing code department. Area Under the Receiver Operating Curve (AUROC) scores were calculated for all trials and iterations of the model. This measures the true positive rate vs the false negative rate which approximates the predictive power of a machine learning model. We trained XLNet, a generalized autoregressive pretraining method, on this dataset and tested its performance in four procedural combinations using a receiver operating characteristic analysis and calculating the AUROC score. Heatmaps were generated to demonstrate the attention that the model was placing on each word in the operative note with a darker color representing greater attention. The performance of the model approached human accuracy, with trial 1 (ACDF) achieving an AUROC score of .82 and a class-by-class accuracy of 77%; trial 2 (PCDF) achieving an AUROC score of .83 and a class-by-class accuracy of 71%; trial 3 (ACDF and CDA) achieving an AUROC score of .95 and a class-by-class accuracy of 87%; and trial 4 (ACDF, PCDF, and CDA) achieving a AUROC score of .95 and a class-by-class accuracy of 88%. In some cases, the model was able to generate CPT codes that the billing department had missed. We show that the XLNet model can be successfully applied to orthopedic surgeon's operative notes to generate CPT billing codes. As NLP models as a whole continue to improve, billing can be greatly augmented with artificial intelligence assisted generation of CPT billing codes which will help minimize error and promote standardization in the process.
更多查看译文
关键词
billing codes,machine learning,orthopedic surgery,operative notes,orthopedic surgery procedure
AI 理解论文
溯源树
样例
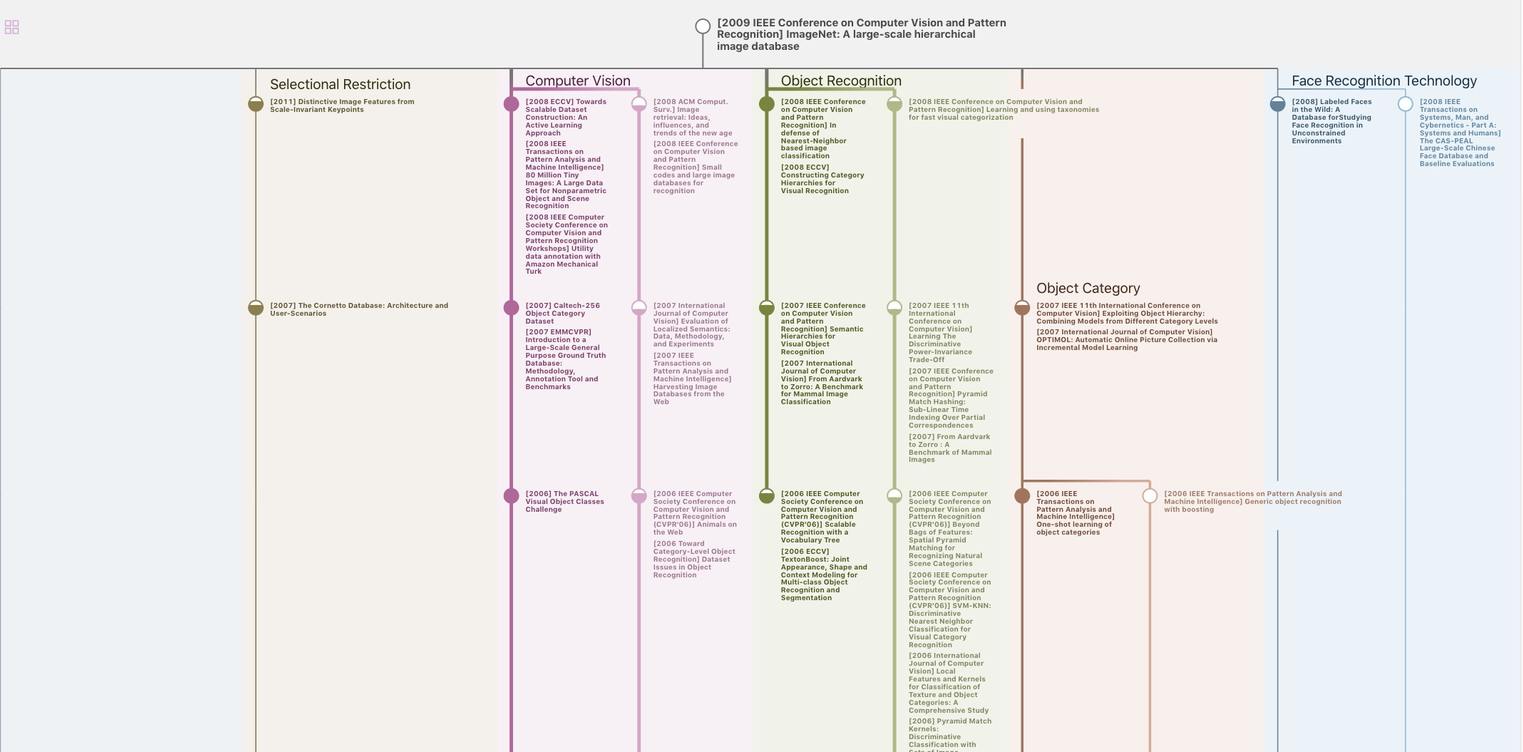
生成溯源树,研究论文发展脉络
Chat Paper
正在生成论文摘要