Seismic Urban Damage Map Generation Based on Satellite Images and Gabor Convolutional Neural Networks
INTERNATIONAL JOURNAL OF APPLIED EARTH OBSERVATION AND GEOINFORMATION(2023)
摘要
Rapid assessment of urban damages after a strong earthquake is a necessary and crucial task to reduce the number of fatalities and recover socioeconomic services. In this paper, a novel deep-learning-based framework is proposed for detecting and mapping damages in urban buildings and roads using post-earthquake high-resolution satellite imagery. The method begins with overlaying a pre-event vector map on an input image to extract the building and road objects. The core machine learning components include two separate convolutional neural networks (CNN), integrated with Gabor filters, which extract debris pixels associated with building and road objects. These debris pixels are analyzed to generate the final damage maps, which show multiple damage degrees for buildings and roads. Two different datasets were used to thoroughly evaluate the proposed method's overall effectiveness. The overall accuracy of 95% for detecting the debris pixels in building and road areas proves the effectiveness of the proposed CNN models for debris detection in comparison to the traditional Machine Learning (ML) methods. The proposed method successfully labelled 84% of the buildings and 87% of the roads when compared with a manually generated multiple damage map.
更多查看译文
关键词
Remote sensing,Earthquake,Buildings,Roads,Damage,Deep learning,Conventional Neural Network,Gabor Filter
AI 理解论文
溯源树
样例
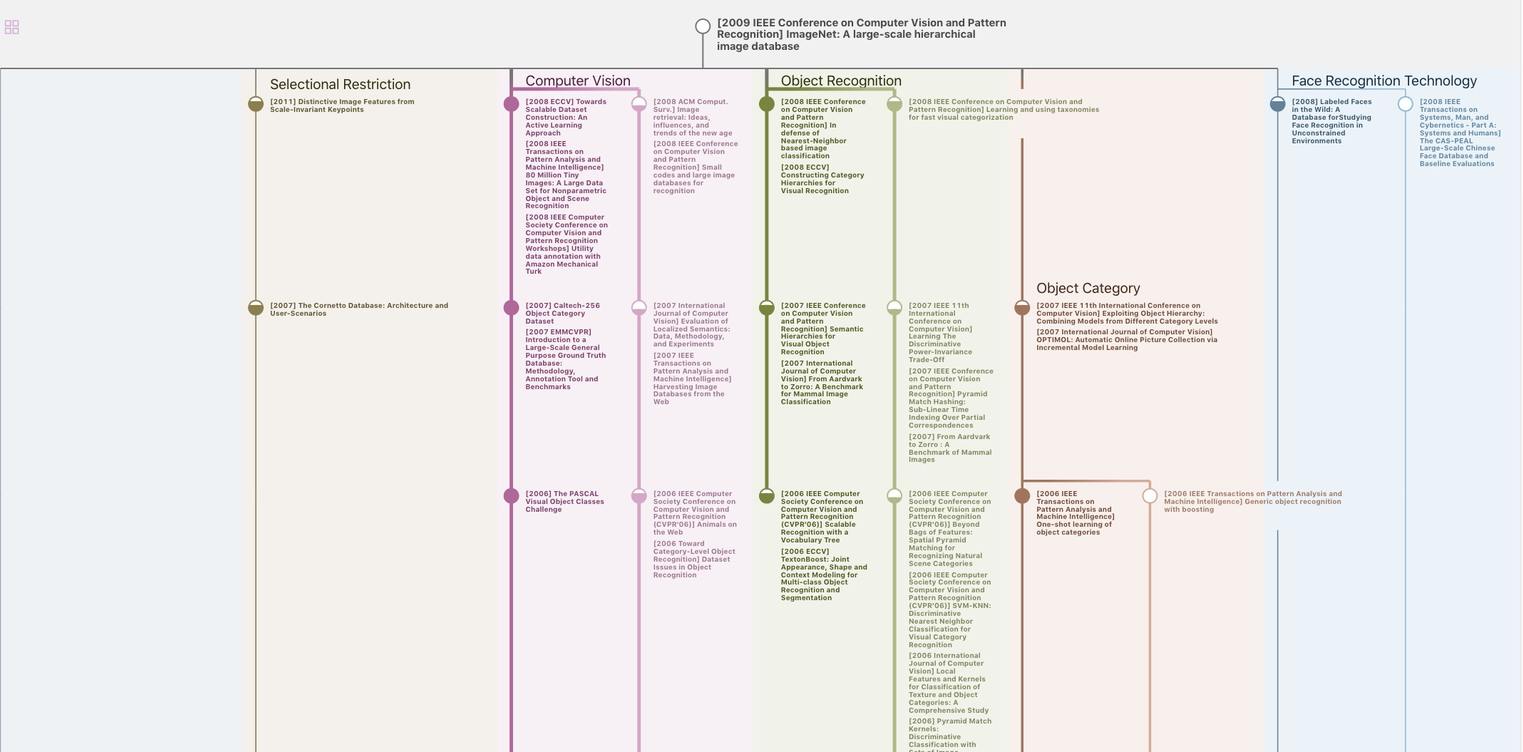
生成溯源树,研究论文发展脉络
Chat Paper
正在生成论文摘要