Variety-aware GAN and online learning augmented self-training model for knowledge graph entity alignment
Information Processing & Management(2023)
摘要
Recently, self-training strategies are adopted in some entity alignment methods, which address the scarcity of training data by selecting newly-aligned pairs from the predicted alignment of unlabeled data. However, the boundary between positive and negative pairs in predicted alignment is difficult to determine, which may lead to inappropriate alignment in newly-aligned pairs. Besides, some pre-aligned pairs have been fitted by the method during training iteration, and combining all pre-aligned pairs with newly-aligned pairs to retrain the method may result in overfitting problems. To address these problems, a Self-training Entity Alignment Framework based on Variety-aware GAN and Online Learning Algorithm named SEAGAN is proposed in this paper. To select reliable newly-aligned pairs from the predicted alignment, a variety-aware GAN with a metric of match variety that eliminates negative pairs differing significantly from positive pairs is designed. It leverages the distribution of entity pairs to determine the boundary between different types of pairs. Moreover, SEAGAN designs an online learning algorithm that combines newly-aligned pairs with their one-hop and two-hop neighbor entities in pre-aligned pairs to update model parameters, which alleviates overfitting problems. We conduct extensive experiments on four real-world datasets to compare SEAGAN with fifteen state-of-the-art methods. Experiment results show that SEAGAN has better performance than state-of-the-art methods on metrics of Prec@1, Prec@5, and MRR.
更多查看译文
关键词
Entity alignment,Self-training,Generative adversarial network,Online learning
AI 理解论文
溯源树
样例
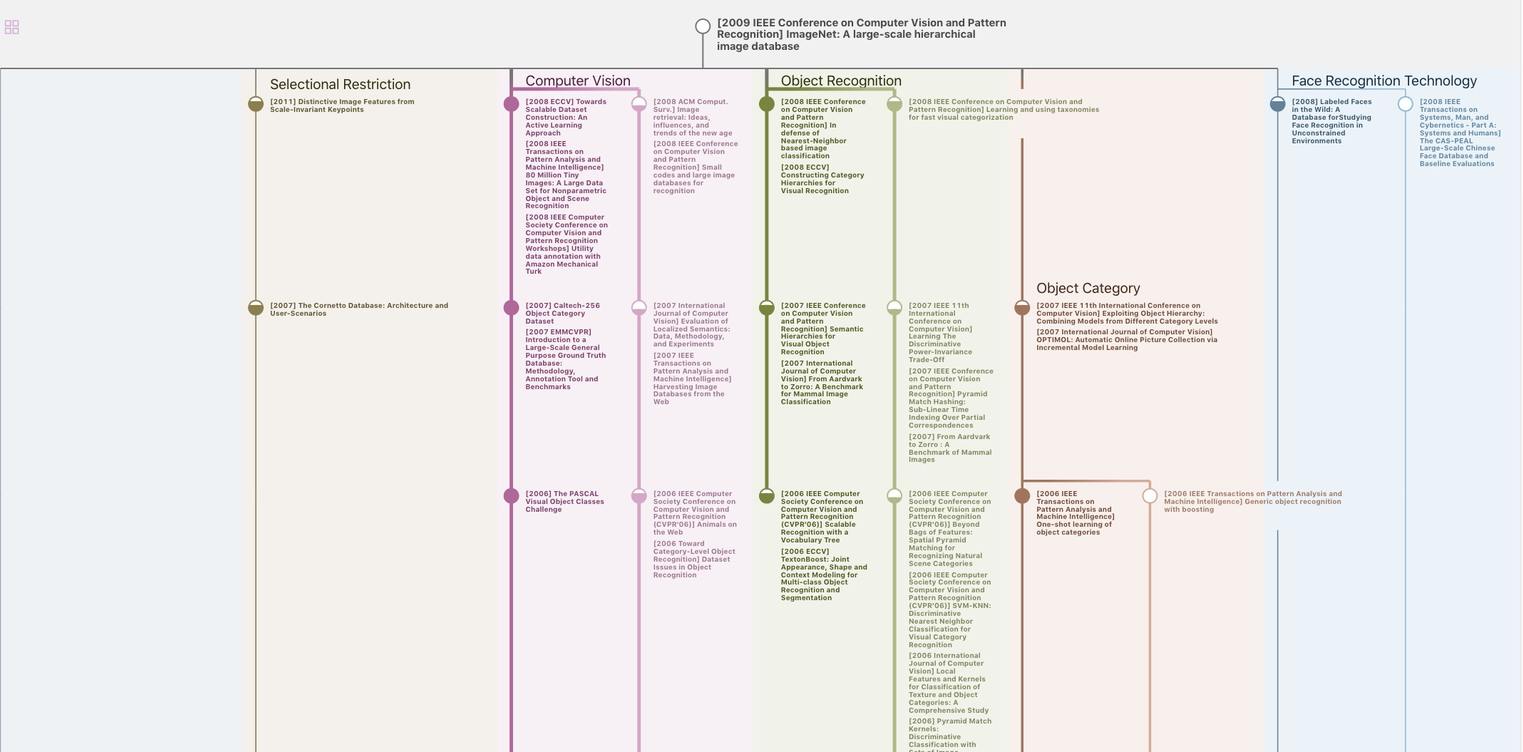
生成溯源树,研究论文发展脉络
Chat Paper
正在生成论文摘要