Discrete Log Anomaly Detection: A Novel Time-Aware Graph-Based Link Prediction Approach
Information Sciences(2023)
摘要
With the implementation of online-service information systems, it is important to detect system anomalies. Logs, serving as the system runtime information, are the key resources to understand system statuses. The existing automatic log anomaly detection approaches focused on the temporal features deriving from sequential nature of logs/events, which can be suitable for limited-sized information systems with homogeneous structure. However, with increasing complexity of systems, the time-aware relationships among discrete logs/events become non-trivial features. Different from the previous works, a novel framework for log anomaly detection is proposed from the perspective of link prediction. First, a temporal event graph is constructed to model the time-aware relationships between events, which is derived from discrete event sequences and possesses the evolutionary properties of sequential events. Second, we pre-train a graph convolutional neural network by using graph contrastive learning to capture local topological features. Third, a temporal convolutional network is used to learn the temporal features. Finally, based on generative adversarial network, link prediction is implemented by generating the next moment event graph, which also is the basis for anomaly detection. Experiments are conducted on public datasets to verify the validity of the model, and superior results are achieved compared to the state-of-the-art methods.
更多查看译文
关键词
Anomaly detection,System log,Link prediction,Graph Neural Network
AI 理解论文
溯源树
样例
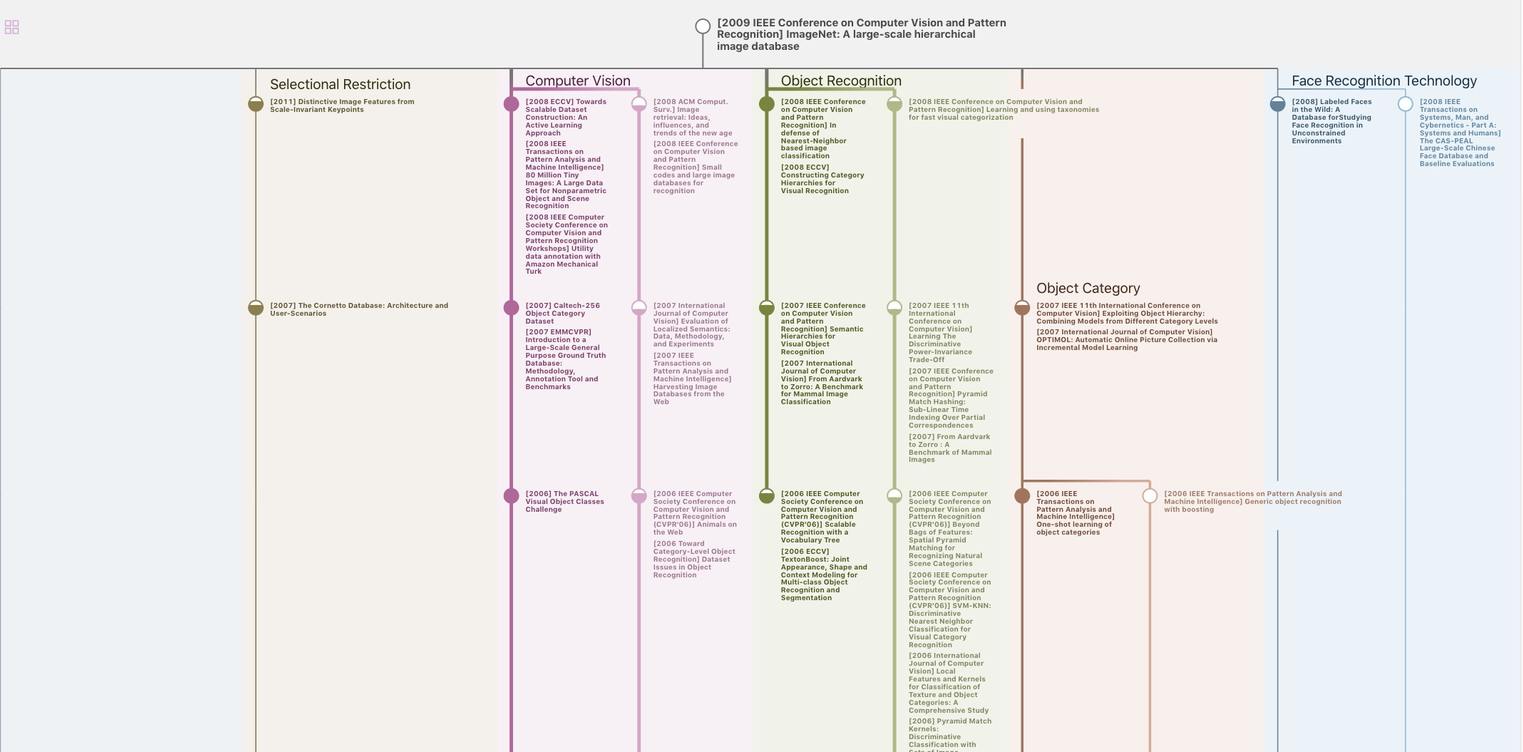
生成溯源树,研究论文发展脉络
Chat Paper
正在生成论文摘要