Rapid and Accurate Prediction of the Axial Magnetic Anisotropy in Cobalt(II) Complexes Using a Machine-Learning Approach.
Inorganic chemistry(2023)
摘要
Estimating the magnetic anisotropy for single-ion magnets is complex due to its multireference nature. This study demonstrates that deep neural networks (DNNs) can provide accurate axial magnetic anisotropy () values, closely matching the complete-active-space self-consistent-field (CASSCF) quality using density functional theory (DFT) data. We curated an 86-parameter database (UFF1) with electronic data from over 33000 cobalt(II) compounds. The DNN achieved an of 0.906 and a mean absolute error of 18.1 cm in comparison to reference CASSCF values. Remarkably, it is 11 times more accurate than DFT methods and 7700 times faster. This approach hints at DNNs predicting the anisotropy in larger molecules, even when trained on smaller ligands.
更多查看译文
关键词
axial magnetic anisotropy,complexes,machine-learning
AI 理解论文
溯源树
样例
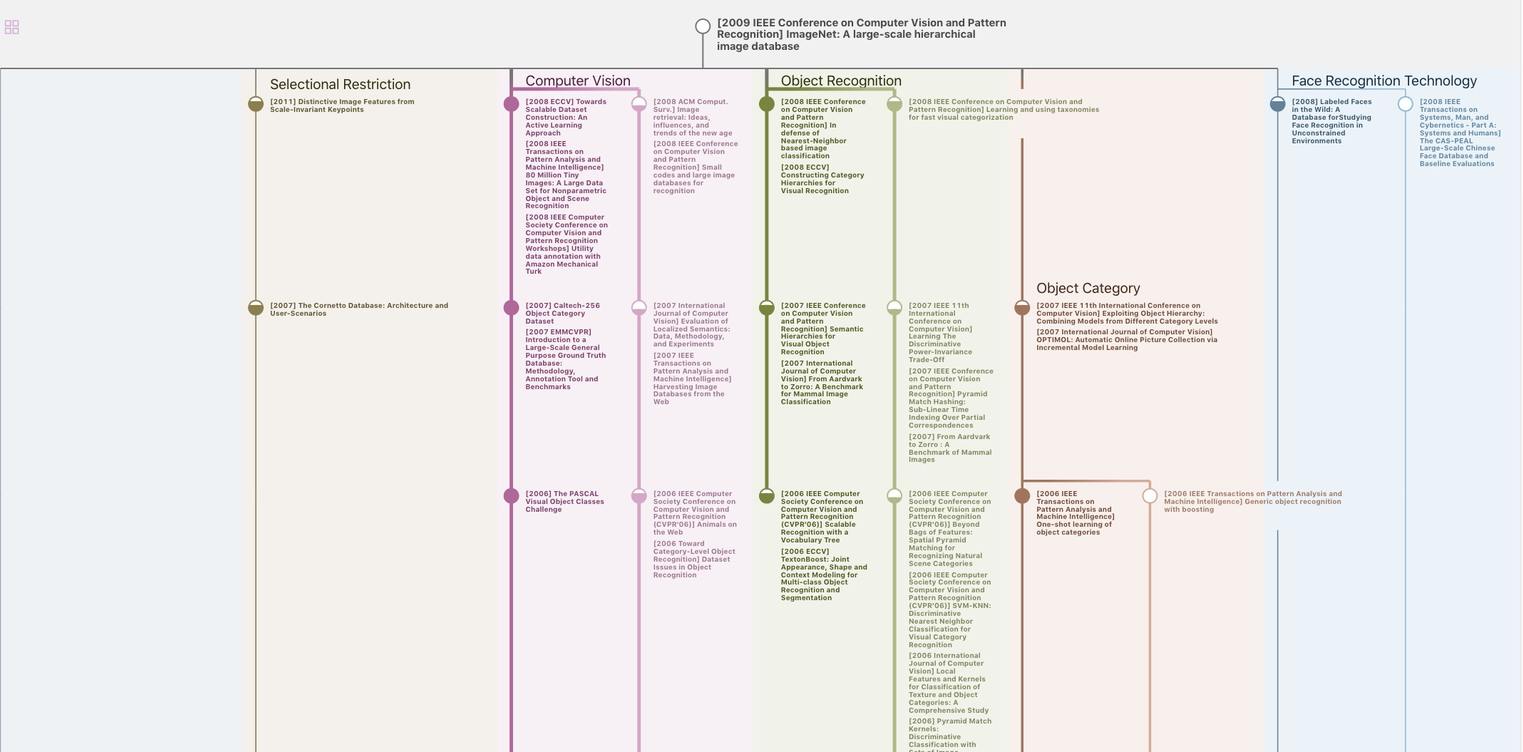
生成溯源树,研究论文发展脉络
Chat Paper
正在生成论文摘要