Bayesian optimization with active learning of Ta-Nb-Hf-Zr-Ti system for spin transport properties
arXiv (Cornell University)(2023)
摘要
Designing materials with enhanced spin charge conversion, i.e., with high spin Hall conductivity (SHC) and low longitudinal electric conductivity (hence large spin Hall angle (SHA)), is a challenging task, especially in the presence of a vast chemical space for compositionally complex alloys (CCAs). In this work, focusing on the Ta-Nb-Hf-Zr-Ti system, we confirm that CCAs exhibit significant spin Hall conductivities and propose a multi-objective Bayesian optimization approach (MOBO) incorporated with active learning (AL) in order to screen for the optimal compositions with significant SHC and SHA. As a result, within less than 5 iterations we are able to target the TaZr-dominated systems displaying both high magnitudes of SHC (~-2.0 (10$^{-3}$ $\Omega$ cm)$^{-1}$) and SHA (~0.03). The SHC is mainly ascribed to the extrinsic skew scattering mechanism. Our work provides an efficient route for identifying new materials with significant SHE, which can be straightforwardly generalized to optimize other properties in a vast chemical space.
更多查看译文
关键词
bayesian optimization,active learning,spin,ta-nb-hf-zr
AI 理解论文
溯源树
样例
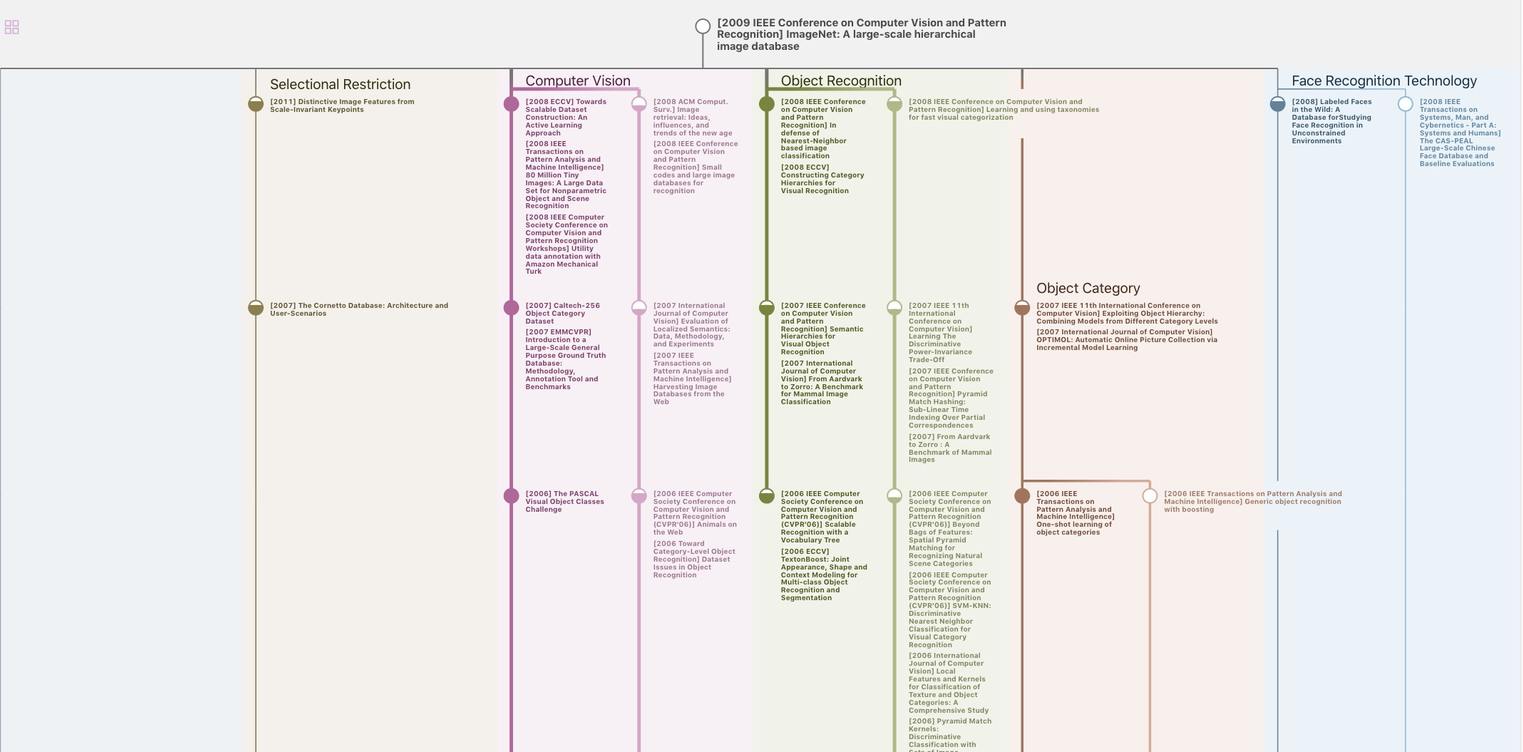
生成溯源树,研究论文发展脉络
Chat Paper
正在生成论文摘要