Provider Fairness and Beyond-Accuracy Trade-offs in Recommender Systems
CoRR(2023)
摘要
Recommender systems, while transformative in online user experiences, have raised concerns over potential provider-side fairness issues. These systems may inadvertently favor popular items, thereby marginalizing less popular ones and compromising provider fairness. While previous research has recognized provider-side fairness issues, the investigation into how these biases affect beyond-accuracy aspects of recommendation systems - such as diversity, novelty, coverage, and serendipity - has been less emphasized. In this paper, we address this gap by introducing a simple yet effective post-processing re-ranking model that prioritizes provider fairness, while simultaneously maintaining user relevance and recommendation quality. We then conduct an in-depth evaluation of the model's impact on various aspects of recommendation quality across multiple datasets. Specifically, we apply the post-processing algorithm to four distinct recommendation models across four varied domain datasets, assessing the improvement in each metric, encompassing both accuracy and beyond-accuracy aspects. This comprehensive analysis allows us to gauge the effectiveness of our approach in mitigating provider biases. Our findings underscore the effectiveness of the adopted method in improving provider fairness and recommendation quality. They also provide valuable insights into the trade-offs involved in achieving fairness in recommender systems, contributing to a more nuanced understanding of this complex issue.
更多查看译文
关键词
provider fairness,recommender systems,beyond-accuracy,trade-offs
AI 理解论文
溯源树
样例
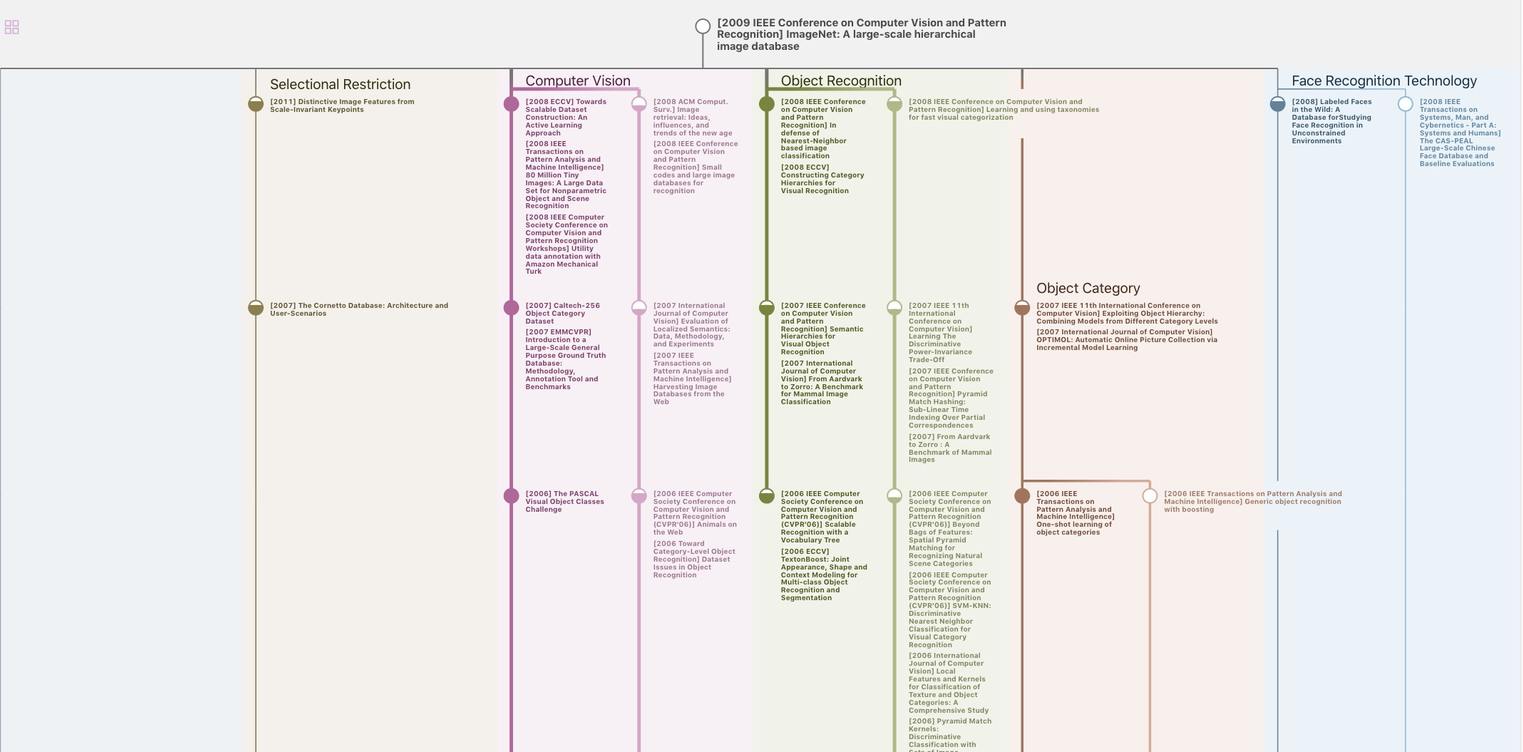
生成溯源树,研究论文发展脉络
Chat Paper
正在生成论文摘要