Fortifying gravitational-wave tests of general relativity against astrophysical assumptions
PHYSICAL REVIEW D(2023)
摘要
Most tests of general relativity with gravitational-wave observations rely on inferring the degree to which a signal deviates from general relativity in conjunction with the astrophysical parameters of its source, such as the component masses and spins of a compact binary. Due to features of the signal, measurements of these deviations are often highly correlated with the properties of astrophysical sources. As a consequence, prior assumptions about astrophysical parameters will generally affect the inferred magnitude of the deviations. Incorporating information about the underlying astrophysical population is necessary to avoid biases in the inference of deviations from general relativity. Current tests assume that the astrophysical population follows an unrealistic fiducial prior chosen to ease sampling of the posterior-for example, a prior flat in component masses-which is inconsistent with both astrophysical expectations and the distribution inferred from observations. We propose a framework for fortifying tests of general relativity by simultaneously inferring the astrophysical population using a catalog of detections. Although this method applies broadly, we demonstrate it concretely on massive graviton constraints and parametrized tests of deviations to the post-Newtonian phase coefficients. Using observations from LIGO-Virgo-KAGRA's third observing run, we show that concurrent inference of the astrophysical distribution strengthens constraints and improves overall consistency with general relativity. We provide updated constraints on deviations from the theory, finding that, upon modeling the astrophysical population, the 90%-credible upper limit on the mass of the graviton improves by 25% to m(g) <= 9.6 x 10(-24) eV=c(2) and the inferred population-level post-Newtonian deviations move similar to 0.4 sigma closer to zero.
更多查看译文
关键词
general relativity,tests,gravitational-wave
AI 理解论文
溯源树
样例
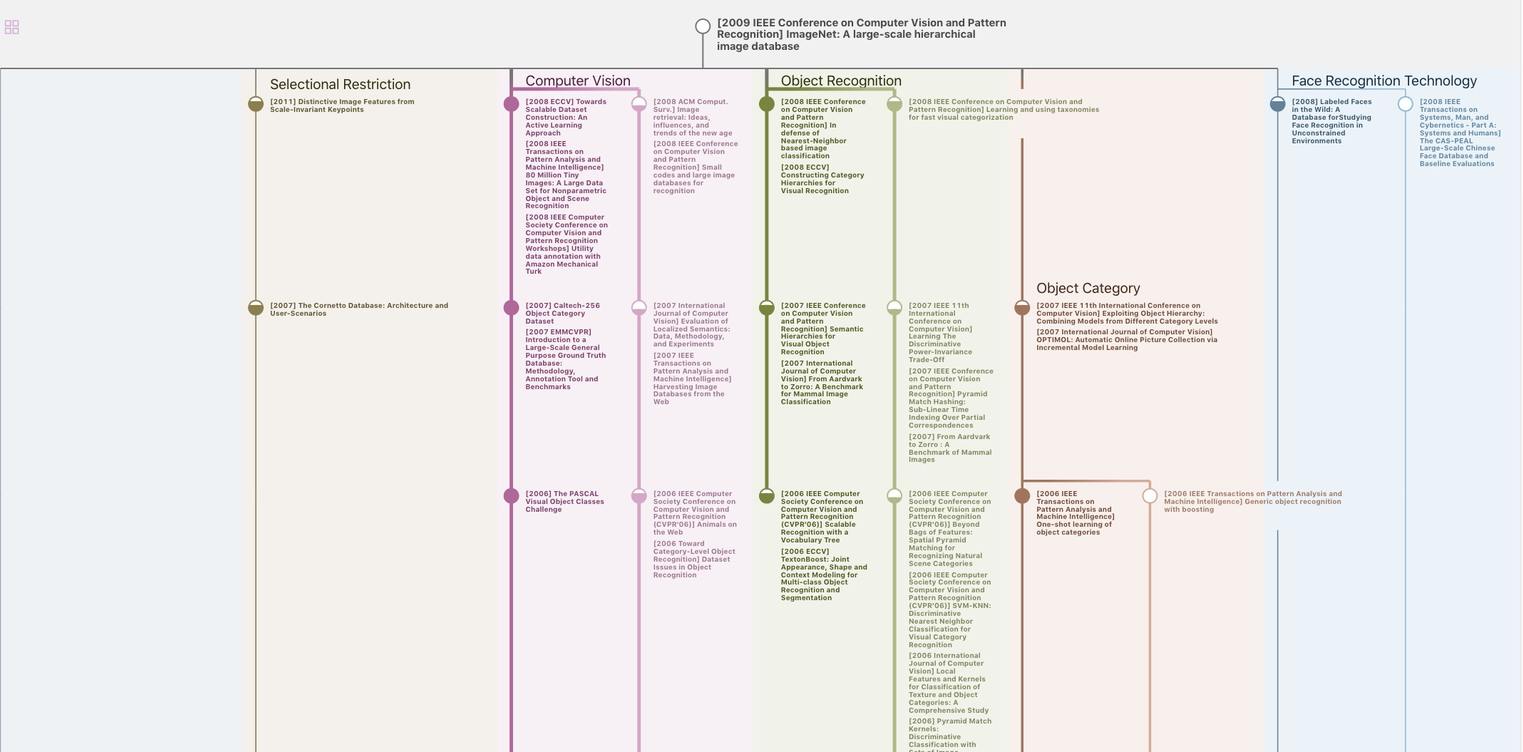
生成溯源树,研究论文发展脉络
Chat Paper
正在生成论文摘要