Motion Compensated Unsupervised Deep Learning for 5D MRI
MEDICAL IMAGE COMPUTING AND COMPUTER ASSISTED INTERVENTION, MICCAI 2023, PT X(2023)
摘要
We propose an unsupervised deep learning algorithm for the motion-compensated reconstruction of 5D cardiac MRI data from 3D radial acquisitions. Ungated free-breathing 5D MRI simplifies the scan planning, improves patient comfort, and offers several clinical benefits over breath-held 2D exams, including isotropic spatial resolution and the ability to reslice the data to arbitrary views. However, the current reconstruction algorithms for 5D MRI take very long computational time, and their outcome is greatly dependent on the uniformity of the binning of the acquired data into different physiological phases. The proposed algorithm is a more data-efficient alternative to current motion-resolved reconstructions. This motion-compensated approach models the data in each cardiac/respiratory bin as Fourier samples of the deformed version of a 3D image template. The deformation maps are modeled by a convolutional neural network driven by the physiological phase information. The deformation maps and the template are then jointly estimated from the measured data. The cardiac and respiratory phases are estimated from 1D navigators using an auto-encoder. The proposed algorithm is validated on 5D bSSFP datasets acquired from two subjects.
更多查看译文
关键词
Free Running MRI,5D MRI,Cardiac MRI
AI 理解论文
溯源树
样例
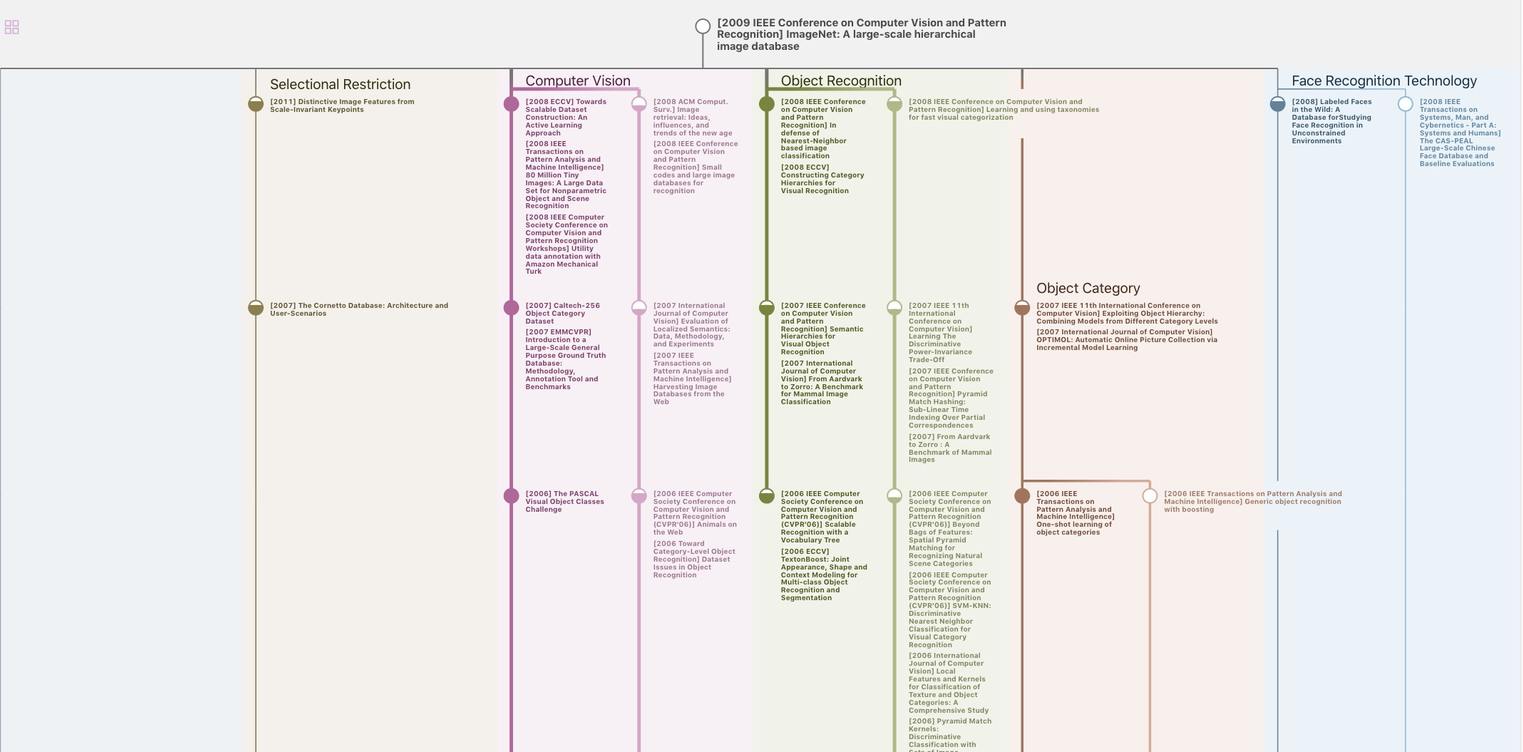
生成溯源树,研究论文发展脉络
Chat Paper
正在生成论文摘要