How to Evaluate Semantic Communications for Images with ViTScore Metric?
arXiv (Cornell University)(2023)
摘要
Semantic communications (SC) have been expected to be a new paradigm shifting to catalyze the next generation communication, whose main concerns shift from accurate bit transmission to effective semantic information exchange in communications. However, the previous and widely-used metrics for images are not applicable to evaluate the image semantic similarity in SC. Classical metrics to measure the similarity between two images usually rely on the pixel level or the structural level, such as the PSNR and the MS-SSIM. Straightforwardly using some tailored metrics based on deep-learning methods in CV community, such as the LPIPS, is infeasible for SC. To tackle this, inspired by BERTScore in NLP community, we propose a novel metric for evaluating image semantic similarity, named Vision Transformer Score (ViTScore). We prove theoretically that ViTScore has 3 important properties, including symmetry, boundedness, and normalization, which make ViTScore convenient and intuitive for image measurement. To evaluate the performance of ViTScore, we compare ViTScore with 3 typical metrics (PSNR, MS-SSIM, and LPIPS) through 5 classes of experiments. Experimental results demonstrate that ViTScore can better evaluate the image semantic similarity than the other 3 typical metrics, which indicates that ViTScore is an effective performance metric when deployed in SC scenarios.
更多查看译文
关键词
vitscore metric,semantic communications
AI 理解论文
溯源树
样例
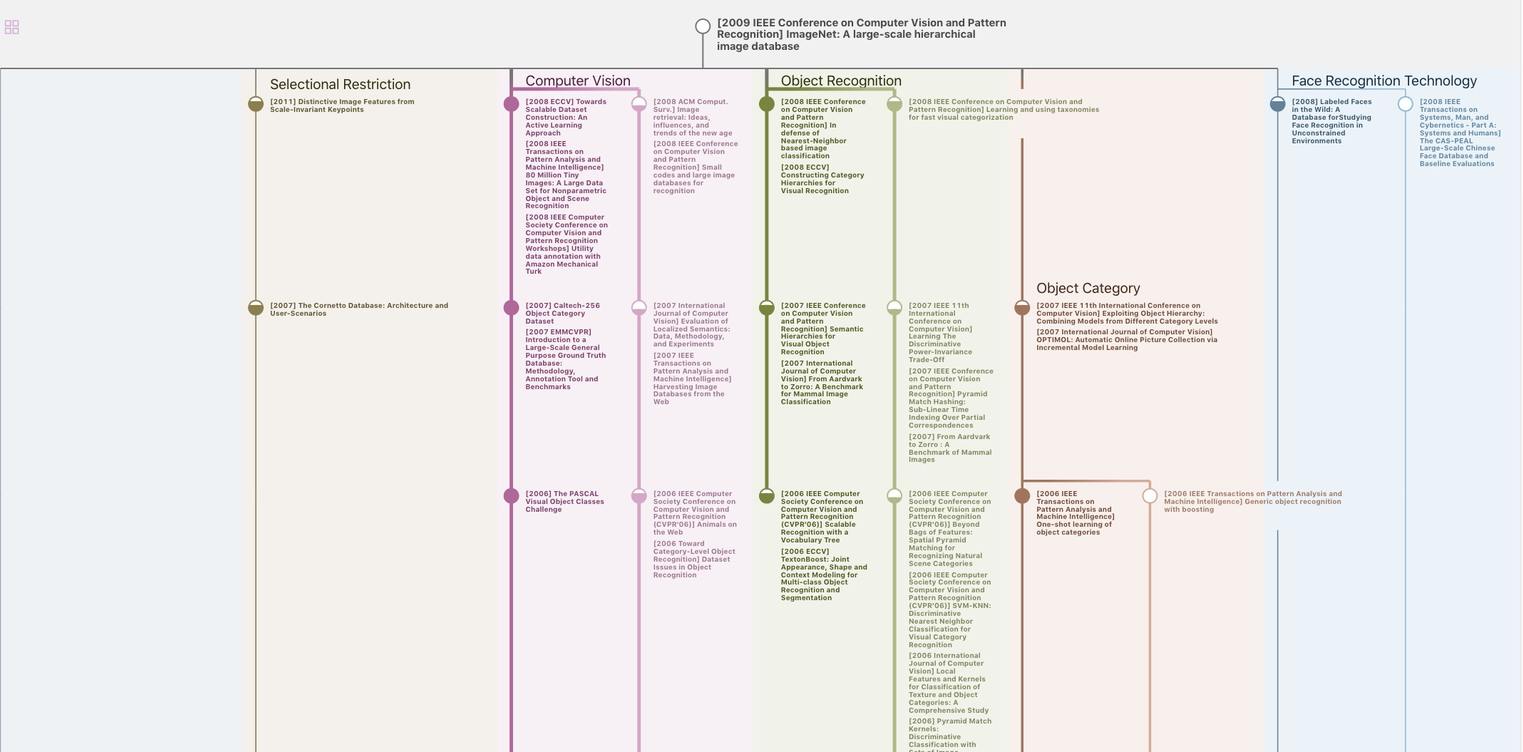
生成溯源树,研究论文发展脉络
Chat Paper
正在生成论文摘要