SdCT-GAN: Reconstructing CT from Biplanar X-Rays with Self-driven Generative Adversarial Networks
CoRR(2023)
摘要
Computed Tomography (CT) is a medical imaging modality that can generate more informative 3D images than 2D X-rays. However, this advantage comes at the expense of more radiation exposure, higher costs, and longer acquisition time. Hence, the reconstruction of 3D CT images using a limited number of 2D X-rays has gained significant importance as an economical alternative. Nevertheless, existing methods primarily prioritize minimizing pixel/voxel-level intensity discrepancies, often neglecting the preservation of textural details in the synthesized images. This oversight directly impacts the quality of the reconstructed images and thus affects the clinical diagnosis. To address the deficits, this paper presents a new self-driven generative adversarial network model (SdCT-GAN), which is motivated to pay more attention to image details by introducing a novel auto-encoder structure in the discriminator. In addition, a Sobel Gradient Guider (SGG) idea is applied throughout the model, where the edge information from the 2D X-ray image at the input can be integrated. Moreover, LPIPS (Learned Perceptual Image Patch Similarity) evaluation metric is adopted that can quantitatively evaluate the fine contours and textures of reconstructed images better than the existing ones. Finally, the qualitative and quantitative results of the empirical studies justify the power of the proposed model compared to mainstream state-of-the-art baselines.
更多查看译文
关键词
generative adversarial networks,sdct-gan,x-rays,self-driven
AI 理解论文
溯源树
样例
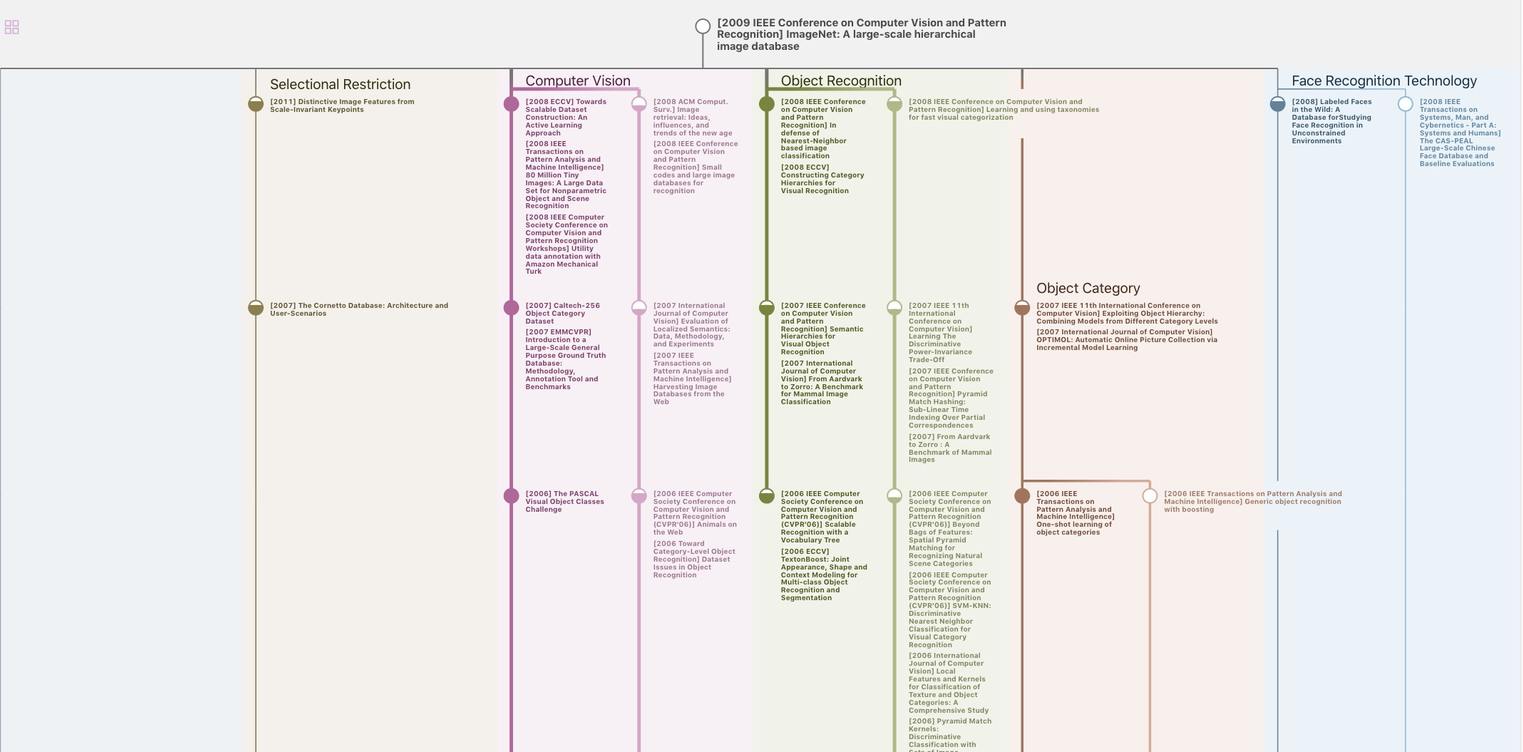
生成溯源树,研究论文发展脉络
Chat Paper
正在生成论文摘要