Prompt Learning With Knowledge Memorizing Prototypes For Generalized Few-Shot Intent Detection
CoRR(2023)
摘要
Generalized Few-Shot Intent Detection (GFSID) is challenging and realistic because it needs to categorize both seen and novel intents simultaneously. Previous GFSID methods rely on the episodic learning paradigm, which makes it hard to extend to a generalized setup as they do not explicitly learn the classification of seen categories and the knowledge of seen intents. To address the dilemma, we propose to convert the GFSID task into the class incremental learning paradigm. Specifically, we propose a two-stage learning framework, which sequentially learns the knowledge of different intents in various periods via prompt learning. And then we exploit prototypes for categorizing both seen and novel intents. Furthermore, to achieve the transfer knowledge of intents in different stages, for different scenarios we design two knowledge preservation methods which close to realistic applications. Extensive experiments and detailed analyses on two widely used datasets show that our framework based on the class incremental learning paradigm achieves promising performance.
更多查看译文
关键词
knowledge memorizing prototypes,learning,detection,few-shot
AI 理解论文
溯源树
样例
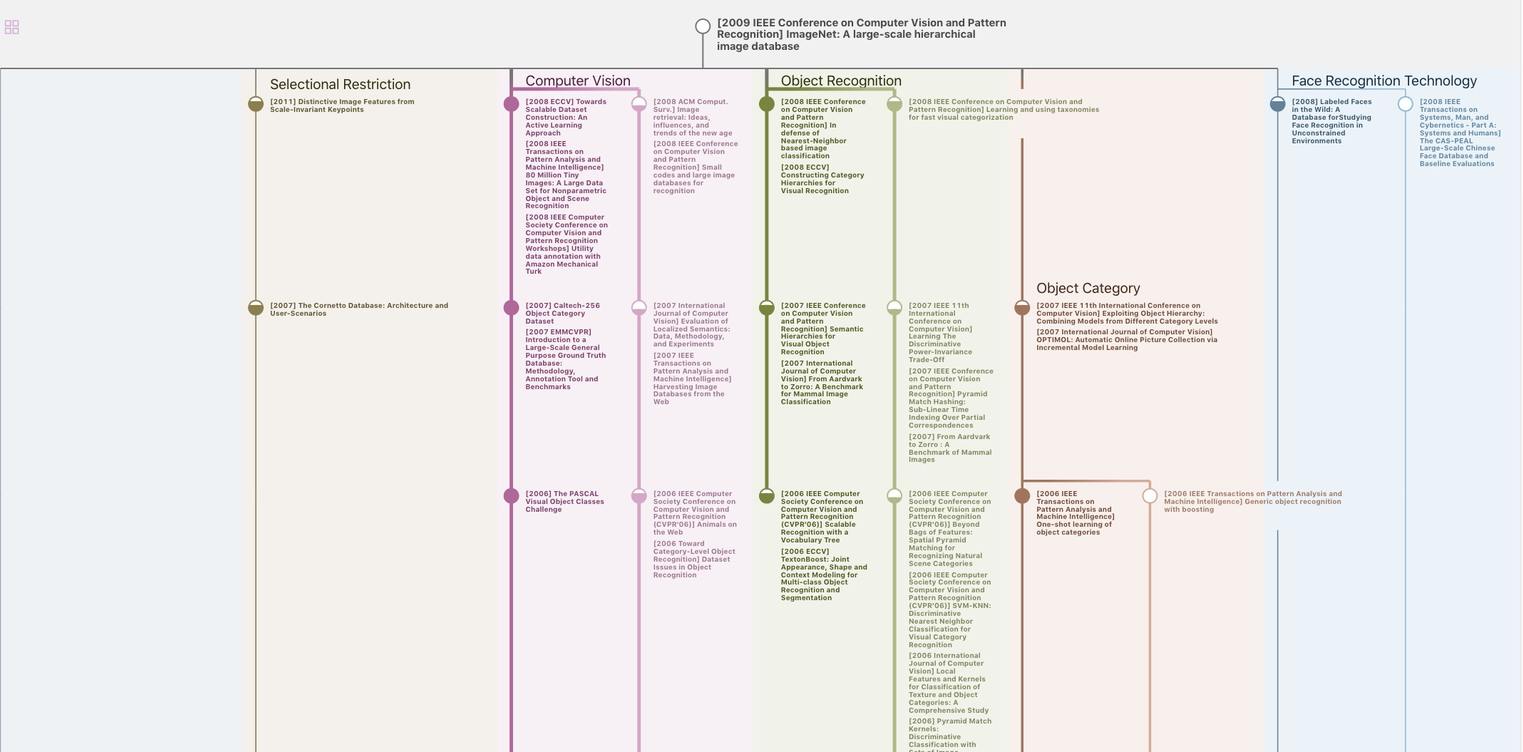
生成溯源树,研究论文发展脉络
Chat Paper
正在生成论文摘要