Task-Driven Compression for Collision Encoding Based on Depth Images
ADVANCES IN VISUAL COMPUTING, ISVC 2023, PT II(2023)
摘要
This paper contributes a novel learning-based method for aggressive task-driven compression of depth images and their encoding as images tailored to collision prediction for robotic systems. A novel 3D image processing methodology is proposed that accounts for the robot's size in order to appropriately "inflate" the obstacles represented in the depth image and thus obtain the distance that can be traversed by the robot in a collision-free manner along any given ray within the camera frustum. Such depth-and-collision image pairs are used to train a neural network that follows the architecture of Variational Autoencoders to compress-and-transform the information in the original depth image to derive a latent representation that encodes the collision information for the given depth image. We compare our proposed task-driven encoding method with classical task-agnostic methods and demonstrate superior performance for the task of collision image prediction from extremely low-dimensional latent spaces. A set of comparative studies show that the proposed approach is capable of encoding depth image-and-collision image tuples from complex scenes with thin obstacles at long distances better than the classical methods at compression ratios as high as 4050:1.
更多查看译文
关键词
Task-driven compression,Collision prediction,Robotics
AI 理解论文
溯源树
样例
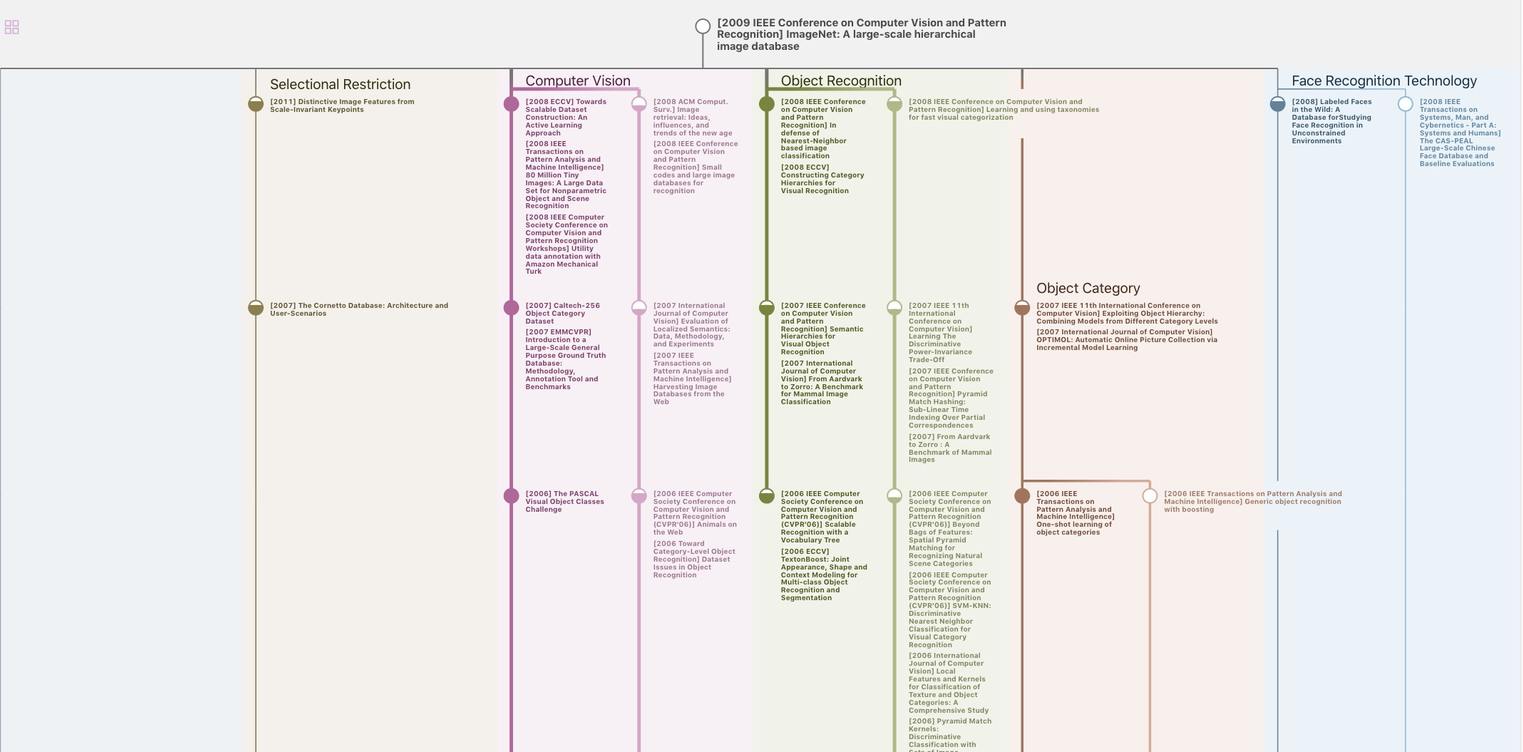
生成溯源树,研究论文发展脉络
Chat Paper
正在生成论文摘要