AI for mammography screening: enter evidence from prospective trials.
The Lancet. Digital health(2023)
摘要
Systematic reviews of the performance of contemporary artificial intelligence (AI) algorithms for mammography screening have highlighted various limitations of early clinical studies, raising concerns about biases and applicability of the evidence to screening practice.1Anderson AW Marinovich ML Houssami N et al.Independent external validation of artificial intelligence algorithms for automated interpretation of screening mammography: a systematic review.J Am Coll Radiol. 2022; 19: 259-273Summary Full Text Full Text PDF PubMed Scopus (8) Google Scholar, 2Freeman K Geppert J Stinton C et al.Use of artificial intelligence for image analysis in breast cancer screening programmes: systematic review of test accuracy.BMJ. 2021; 374n1872PubMed Google Scholar, 3Houssami N Kirkpatrick-Jones G Noguchi N Lee CI Artificial Intelligence (AI) for the early detection of breast cancer: a scoping review to assess AI's potential in breast screening practice.Expert Rev Med Devices. 2019; 16: 351-362Crossref PubMed Scopus (92) Google Scholar Limitations included use of small and highly selected (cancer-enriched) datasets to train or test the AI algorithms, limited independent validation, inadequate ascertainment of cancer outcomes, and few comparisons of algorithms with screen-readers in real-world practice.1Anderson AW Marinovich ML Houssami N et al.Independent external validation of artificial intelligence algorithms for automated interpretation of screening mammography: a systematic review.J Am Coll Radiol. 2022; 19: 259-273Summary Full Text Full Text PDF PubMed Scopus (8) Google Scholar, 2Freeman K Geppert J Stinton C et al.Use of artificial intelligence for image analysis in breast cancer screening programmes: systematic review of test accuracy.BMJ. 2021; 374n1872PubMed Google Scholar, 3Houssami N Kirkpatrick-Jones G Noguchi N Lee CI Artificial Intelligence (AI) for the early detection of breast cancer: a scoping review to assess AI's potential in breast screening practice.Expert Rev Med Devices. 2019; 16: 351-362Crossref PubMed Scopus (92) Google Scholar Some of these limitations were addressed by retrospective, large-scale cohort studies that evaluated AI algorithms in consecutive screening participants from populations that were independent of the datasets used to train the AI.4Larsen M Aglen CF Lee CI et al.Artificial intelligence evaluation of 122 969 mammography examinations from a population-based screening program.Radiology. 2022; 303: 502-511Crossref PubMed Scopus (19) Google Scholar, 5Marinovich ML Wylie E Lotter W et al.Artificial intelligence (AI) for breast cancer screening: BreastScreen population-based cohort study of cancer detection.EBioMedicine. 2023; 90104498Summary Full Text Full Text PDF Scopus (0) Google Scholar Such studies suggested that AI could detect breast cancer, including interval cancers that were not detected at screening by human readers.4Larsen M Aglen CF Lee CI et al.Artificial intelligence evaluation of 122 969 mammography examinations from a population-based screening program.Radiology. 2022; 303: 502-511Crossref PubMed Scopus (19) Google Scholar, 5Marinovich ML Wylie E Lotter W et al.Artificial intelligence (AI) for breast cancer screening: BreastScreen population-based cohort study of cancer detection.EBioMedicine. 2023; 90104498Summary Full Text Full Text PDF Scopus (0) Google Scholar Retrospective studies also suggested that AI, when integrated into screening workflows as a replacement for one of two human readers (noting that most screening programmes use double reading) can potentially lower rates of false positive recall (hence also unnecessary investigations in screening participants) and reduce overall screen-reading volume. Reduced screen-reading volume promises economic and efficiency benefits for screening programmes. However, retrospective studies are limited in the extent to which they can replicate real-world screening workflow, particularly the resolution of discordant findings between AI and human readers, and reader interpretation of the mammogram with knowledge of the AI result. Use of historical screen-reading results to simulate arbitration or AI-supported decision making is problematic and likely to underestimate the contribution of AI to screen detection in breast cancer screening practice. In The Lancet Digital Health, the study by Karin Dembrower and colleagues,6Dembrower K Crippa A Colón E Eklund M Strand F Artificial intelligence for breast cancer detection in screening mammography in Sweden: a prospective, population-based, paired-reader, non-inferiority study.Lancet Digit Health. 2023; (published online Sept 8.)https://doi.org/10.1016/S2589-7500(23)00153-XGoogle Scholar along with the recent publication of interim results from a randomised controlled trial,7Lång K Josefsson V Larsson A-M et al.Artificial intelligence-supported screen reading versus standard double reading in the Mammography Screening with Artificial Intelligence trial (MASAI): a clinical safety analysis of a randomised, controlled, non-inferiority, single-blinded, screening accuracy study.Lancet Oncol. 2023; 24: 936-944Summary Full Text Full Text PDF PubMed Google Scholar both from Sweden, heralds the arrival of prospective trials of AI in the breast screening setting. The authors report data from a prospectively recruited cohort in which the paired results of screening with and without AI were collected for each screening participant. In a notable methodological strength, discordance between two human readers was prospectively arbitrated by consensus discussion, as was discordance between the first reader and AI. Given that all consensus discussions were informed by AI findings in this study,6Dembrower K Crippa A Colón E Eklund M Strand F Artificial intelligence for breast cancer detection in screening mammography in Sweden: a prospective, population-based, paired-reader, non-inferiority study.Lancet Digit Health. 2023; (published online Sept 8.)https://doi.org/10.1016/S2589-7500(23)00153-XGoogle Scholar this potentially limits interpretation of outcomes in the no-AI strategy, possibly biasing comparisons with AI screening towards the null. By recalling and verifying positive results from both screening strategies, relative true positive (screen-detected cancer) and false positive (unnecessary recall) proportions could be estimated.8Bossuyt PM Irwig L Craig J Glasziou P Comparative accuracy: assessing new tests against existing diagnostic pathways.BMJ. 2006; 332: 1089-1092Crossref PubMed Google Scholar The results show that cancer detection for human plus AI reading, with consensus discussion, was superior to radiologist double-reading.6Dembrower K Crippa A Colón E Eklund M Strand F Artificial intelligence for breast cancer detection in screening mammography in Sweden: a prospective, population-based, paired-reader, non-inferiority study.Lancet Digit Health. 2023; (published online Sept 8.)https://doi.org/10.1016/S2589-7500(23)00153-XGoogle Scholar Along with these encouraging cancer detection findings, the study reports a concomitant small but statistically significant reduction in false-positive recall (despite a higher abnormal interpretation proportion for AI before consensus discussion), amplifying signals from retrospective data5Marinovich ML Wylie E Lotter W et al.Artificial intelligence (AI) for breast cancer screening: BreastScreen population-based cohort study of cancer detection.EBioMedicine. 2023; 90104498Summary Full Text Full Text PDF Scopus (0) Google Scholar that some form of human arbitration is crucial for limiting recall from additional AI false-positives. Importantly, the study also highlights challenges in AI abnormality threshold selection that are of practical importance for implementation.5Marinovich ML Wylie E Lotter W et al.Artificial intelligence (AI) for breast cancer screening: BreastScreen population-based cohort study of cancer detection.EBioMedicine. 2023; 90104498Summary Full Text Full Text PDF Scopus (0) Google Scholar Breast cancer screening programmes worldwide are likely to welcome the findings from these prospective AI trials.6Dembrower K Crippa A Colón E Eklund M Strand F Artificial intelligence for breast cancer detection in screening mammography in Sweden: a prospective, population-based, paired-reader, non-inferiority study.Lancet Digit Health. 2023; (published online Sept 8.)https://doi.org/10.1016/S2589-7500(23)00153-XGoogle Scholar, 7Lång K Josefsson V Larsson A-M et al.Artificial intelligence-supported screen reading versus standard double reading in the Mammography Screening with Artificial Intelligence trial (MASAI): a clinical safety analysis of a randomised, controlled, non-inferiority, single-blinded, screening accuracy study.Lancet Oncol. 2023; 24: 936-944Summary Full Text Full Text PDF PubMed Google Scholar The findings provide scientific justification for planning programme-embedded prospective trials to assess the effectiveness of AI in various workflows and screen-reading strategies. However, although AI seems poised to improve efficiencies in breast screening programmes, the health impact of AI screen-reading is unknown. With resourcing needs and efficiencies in large volume screen-reading in mind, long-term breast cancer mortality outcomes are unlikely to be awaited. However, surrogate endpoints, such as interval cancer rates, will be crucial to understanding potential health impact (if any) and, importantly, to reassure those providing screening programmes about the safety of substituting AI for human readers. The design of Dembrower and colleagues' study included triple reading by two radiologists plus AI and was not randomised (all participants had AI screen reading) so will not provide insights into the effect of integrating AI (versus standard screen reading) on interval cancer rates. Lång and colleagues7Lång K Josefsson V Larsson A-M et al.Artificial intelligence-supported screen reading versus standard double reading in the Mammography Screening with Artificial Intelligence trial (MASAI): a clinical safety analysis of a randomised, controlled, non-inferiority, single-blinded, screening accuracy study.Lancet Oncol. 2023; 24: 936-944Summary Full Text Full Text PDF PubMed Google Scholar are yet to report the interval cancer rates from their randomised controlled trial, and those responsible for screening programmes will look for evidence from that trial that AI has not led to an increase in interval cancer rates. Although many would expect that the increased detection of cancer by AI, shown in both prospective trials,6Dembrower K Crippa A Colón E Eklund M Strand F Artificial intelligence for breast cancer detection in screening mammography in Sweden: a prospective, population-based, paired-reader, non-inferiority study.Lancet Digit Health. 2023; (published online Sept 8.)https://doi.org/10.1016/S2589-7500(23)00153-XGoogle Scholar, 7Lång K Josefsson V Larsson A-M et al.Artificial intelligence-supported screen reading versus standard double reading in the Mammography Screening with Artificial Intelligence trial (MASAI): a clinical safety analysis of a randomised, controlled, non-inferiority, single-blinded, screening accuracy study.Lancet Oncol. 2023; 24: 936-944Summary Full Text Full Text PDF PubMed Google Scholar would have the desirable effect of reducing interval cancers, this might not occur if that increase in detection includes a disproportionate amount of in-situ malignancy, as reported in the randomised controlled trial.7Lång K Josefsson V Larsson A-M et al.Artificial intelligence-supported screen reading versus standard double reading in the Mammography Screening with Artificial Intelligence trial (MASAI): a clinical safety analysis of a randomised, controlled, non-inferiority, single-blinded, screening accuracy study.Lancet Oncol. 2023; 24: 936-944Summary Full Text Full Text PDF PubMed Google Scholar With the emergence of new evidence from prospective trials of AI for breast screening, population screening programmes and imaging services will also need to consider participants' views and expectations of the performance of AI before it can be implemented in the screening process.9Carter SM Carolan L Saint James Aquino Y et al.Australian women's judgements about using artificial intelligence to read mammograms in breast cancer screening.Digit Health. 2023; 9 (20552076231191057)Google Scholar Maintaining public trust in cancer screening programmes is crucial to ensuring that potential benefits from AI screening are fully realised. NH receives funding via a National Breast Cancer Foundation Chair in Breast Cancer Prevention grant (EC-21-001) and National Health and Medical Research Council Investigator (Leader) grant (1194410). MLM receives funding via a National Breast Cancer Foundation Investigator Initiated Research Scheme grant (IIRS-20-011). Artificial intelligence for breast cancer detection in screening mammography in Sweden: a prospective, population-based, paired-reader, non-inferiority studyReplacing one radiologist with AI for independent reading of screening mammograms resulted in a 4% higher non-inferior cancer detection rate compared with radiologist double reading. Our study suggests that AI in the study setting has potential for controlled implementation, which would include risk management and real-world follow-up of performance. Full-Text PDF Open Access
更多查看译文
关键词
mammography,screening,ai,trials
AI 理解论文
溯源树
样例
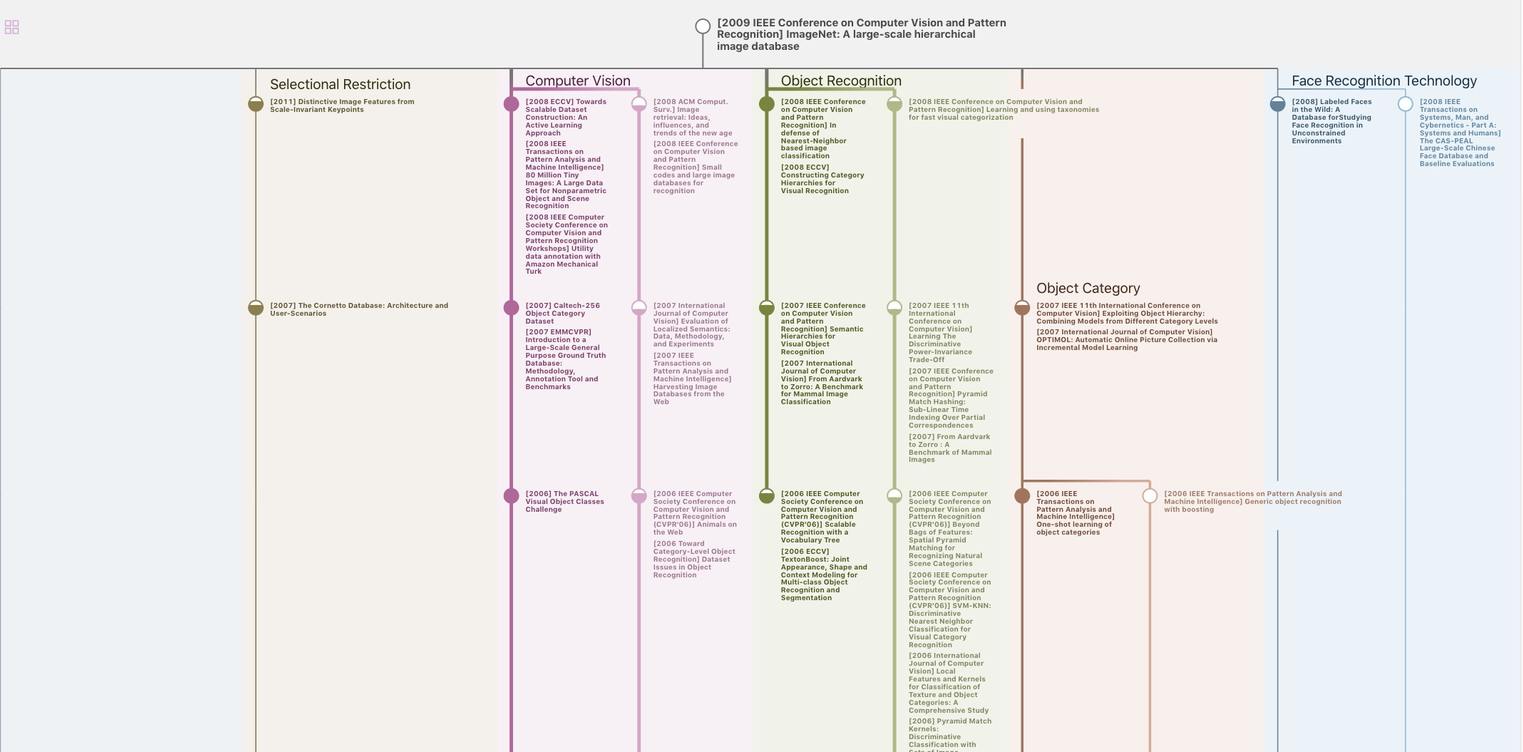
生成溯源树,研究论文发展脉络
Chat Paper
正在生成论文摘要