CCD-GAN for Domain Adaptation in Time-Frequency Localization-Based Wideband Spectrum Sensing
IEEE Communications Letters(2023)
摘要
In practical spectrum sensing scenarios, sample distribution in the training dataset, i.e., source spectrum domain, is generally different from that of the test dataset, i.e., target spectrum domain, which results in the domain adaptation problem. For the emerging time-frequency localization (TFL) based wideband spectrum sensing, we propose a Consistency Constrained Dual Generative Adversarial Network (CCD-GAN) to address this problem. To achieve multi-level feature alignment in the TFL task, we introduce consistency constraints into a dual GAN, which takes into account domain consistency, content consistency, and TFL distribution consistency. Moreover, we retrain a lightweight detector and use transfer learning to improve the retraining efficiency. Finally, experimental simulation proves the effectiveness of CCD-GAN in solving domain adaptation problems, and shows the improvement of generalization ability and convergence speed brought by transfer learning.
更多查看译文
关键词
Spectrum sensing,time-frequency localization,domain adaptation,adversarial learning,transfer learning
AI 理解论文
溯源树
样例
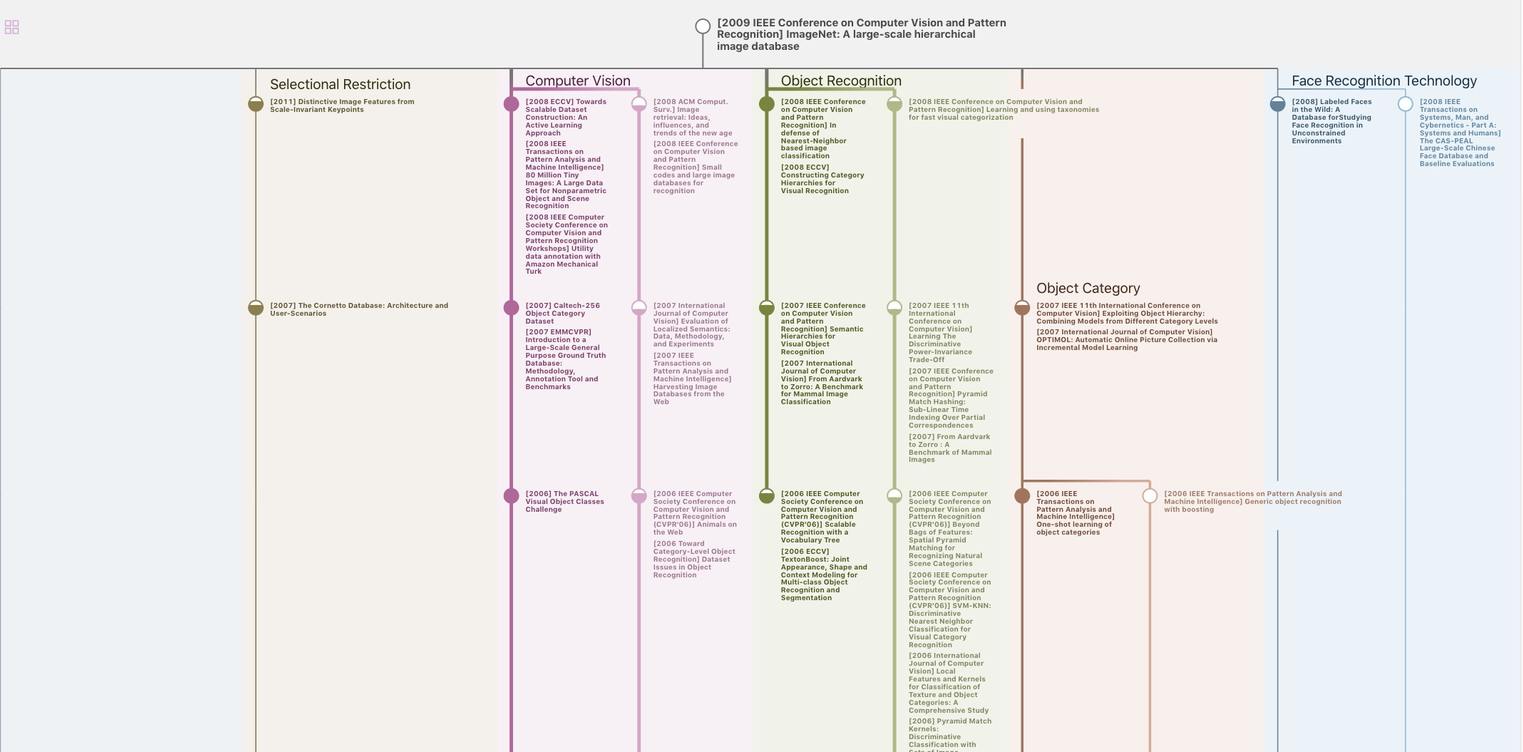
生成溯源树,研究论文发展脉络
Chat Paper
正在生成论文摘要