Task-agnostic open-set prototype for few-shot open-set recognition
2023 IEEE INTERNATIONAL CONFERENCE ON IMAGE PROCESSING, ICIP(2023)
摘要
In few-shot open-set recognition (FSOSR), a network learns to recognize closed-set samples with a few support samples while rejecting open-set samples with no class cue. Unlike conventional OSR, the FSOSR considers more practical open worlds where a closed-set class can be selected as an open-set class in another testing (task) and vice versa. Existing FSOSR methods have commonly represented the open set with task-dependent extra modules. These modules decently handle the varied closed and open classes but accompany inevitable complexity increase. This paper shows that a single open-set prototype can represent open-set samples when it satisfies a specific relation in metric space: closest to open-set, and simultaneously second nearest to close-set. We propose a task-agnostic open-set prototype with distance scaling factors and design loss terms. We extensively analyze the proposed components to demonstrate their importance. Our method achieves state-of-the-art results on miniImageNet and tieredImageNet, respectively, without task-dependent extra modules.
更多查看译文
关键词
Few-shot learning,open-set recognition,task agnostic open-set prototype
AI 理解论文
溯源树
样例
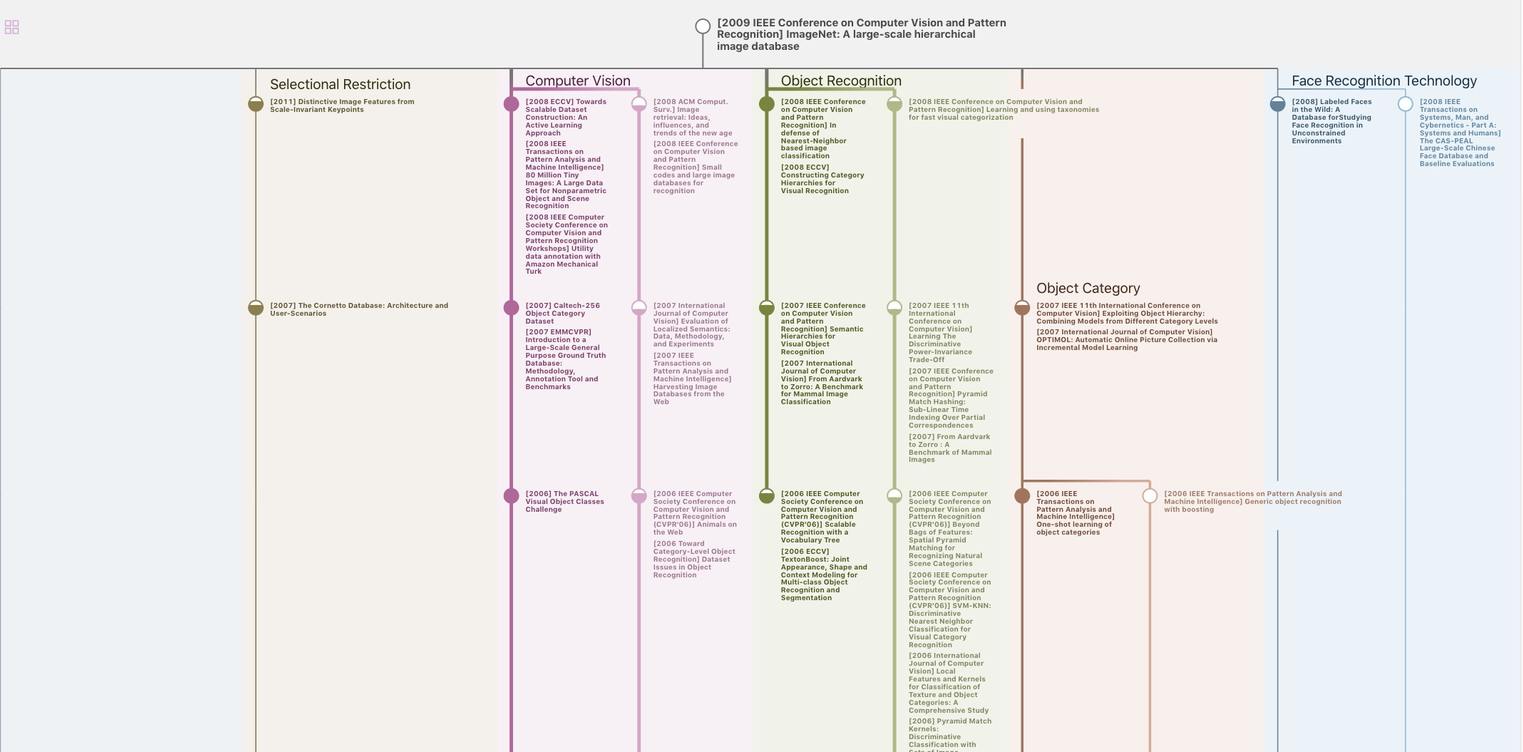
生成溯源树,研究论文发展脉络
Chat Paper
正在生成论文摘要