Joint demosaicing and denoising with gradient guidance in quad bayer cfa
2023 IEEE INTERNATIONAL CONFERENCE ON IMAGE PROCESSING, ICIP(2023)
摘要
In this study, we introduce a challenging task called Joint Demosaicking and Denoising in Quad Bayer CFA (JDD-QBC). Inspired by the effectiveness of gradient prior in previous tasks, we design a gradient prior extraction algorithm to extract robust gradient profiles directly from Quad Bayer raw images. By leveraging contextual information, our algorithm overcomes the limitations of previous gradient extraction algorithms. Building on this prior, we propose an end-to-end JDD-QBC framework called Gradient Guidance Network (G(2)-Net), consisting of two specifically designed components: a Gradient Refinement Branch (GRB) to transform the extracted gradient prior, and a Multi-Scale Backbone Branch (MSB2) to recover all missing values under the guidance of transformed prior. Extensive experiments demonstrate our proposed G(2)-Net outperforms state-of-the-art methods across a wide range of noise levels while requiring 50% less memory cost.
更多查看译文
关键词
Demosaicing,Denoising,Quad Bayer CFA,Image Signal Processing
AI 理解论文
溯源树
样例
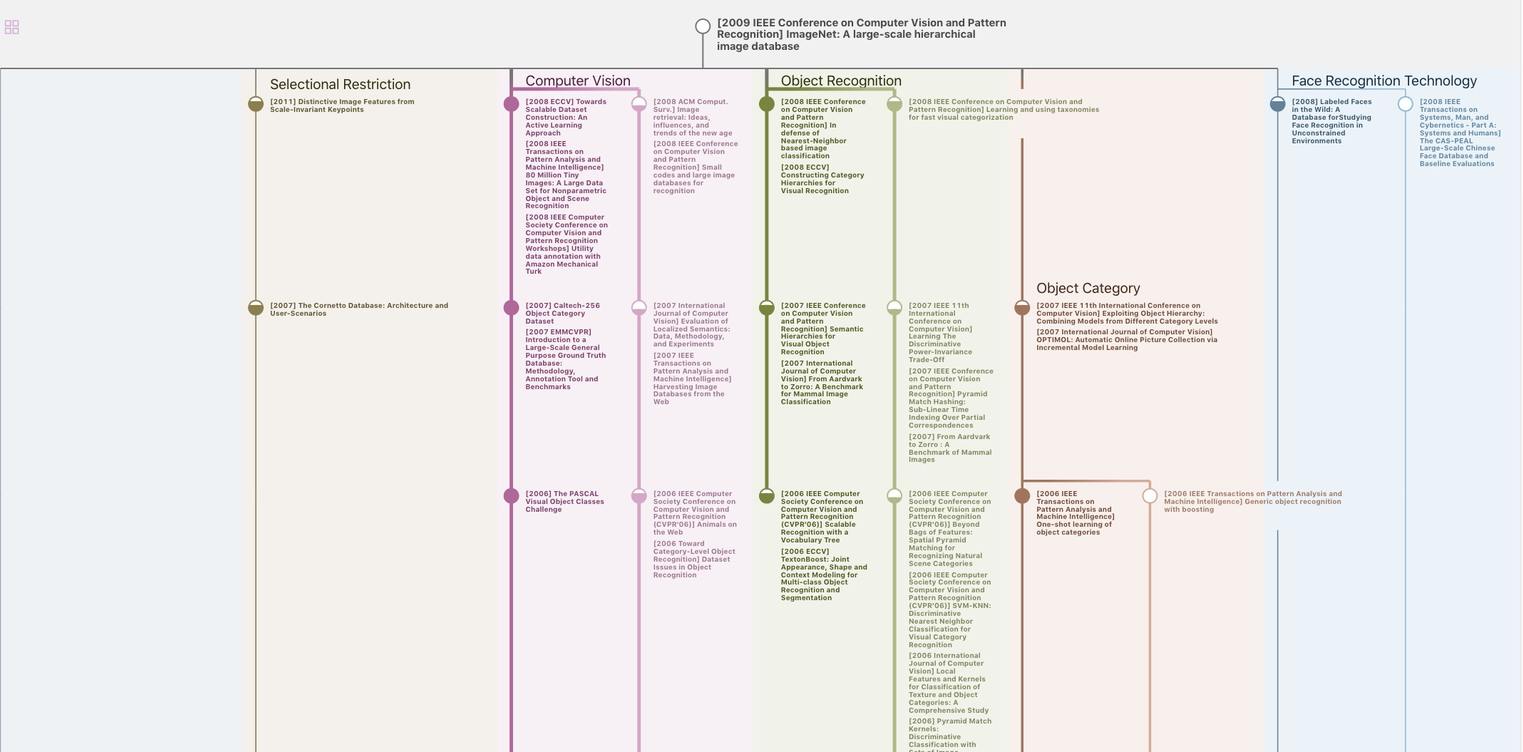
生成溯源树,研究论文发展脉络
Chat Paper
正在生成论文摘要