Temporal Patience: Efficient Adaptive Deep Learning for Embedded Radar Data Processing
CoRR(2023)
摘要
Radar sensors offer power-efficient solutions for always-on smart devices, but processing the data streams on resource-constrained embedded platforms remains challenging. This paper presents novel techniques that leverage the temporal correlation present in streaming radar data to enhance the efficiency of Early Exit Neural Networks for Deep Learning inference on embedded devices. These networks add additional classifier branches between the architecture's hidden layers that allow for an early termination of the inference if their result is deemed sufficient enough by an at-runtime decision mechanism. Our methods enable more informed decisions on when to terminate the inference, reducing computational costs while maintaining a minimal loss of accuracy. Our results demonstrate that our techniques save up to 26% of operations per inference over a Single Exit Network and 12% over a confidence-based Early Exit version. Our proposed techniques work on commodity hardware and can be combined with traditional optimizations, making them accessible for resource-constrained embedded platforms commonly used in smart devices. Such efficiency gains enable real-time radar data processing on resource-constrained platforms, allowing for new applications in the context of smart homes, Internet-of-Things, and human-computer interaction.
更多查看译文
关键词
efficient adaptive deep learning,deep learning,embedded radar
AI 理解论文
溯源树
样例
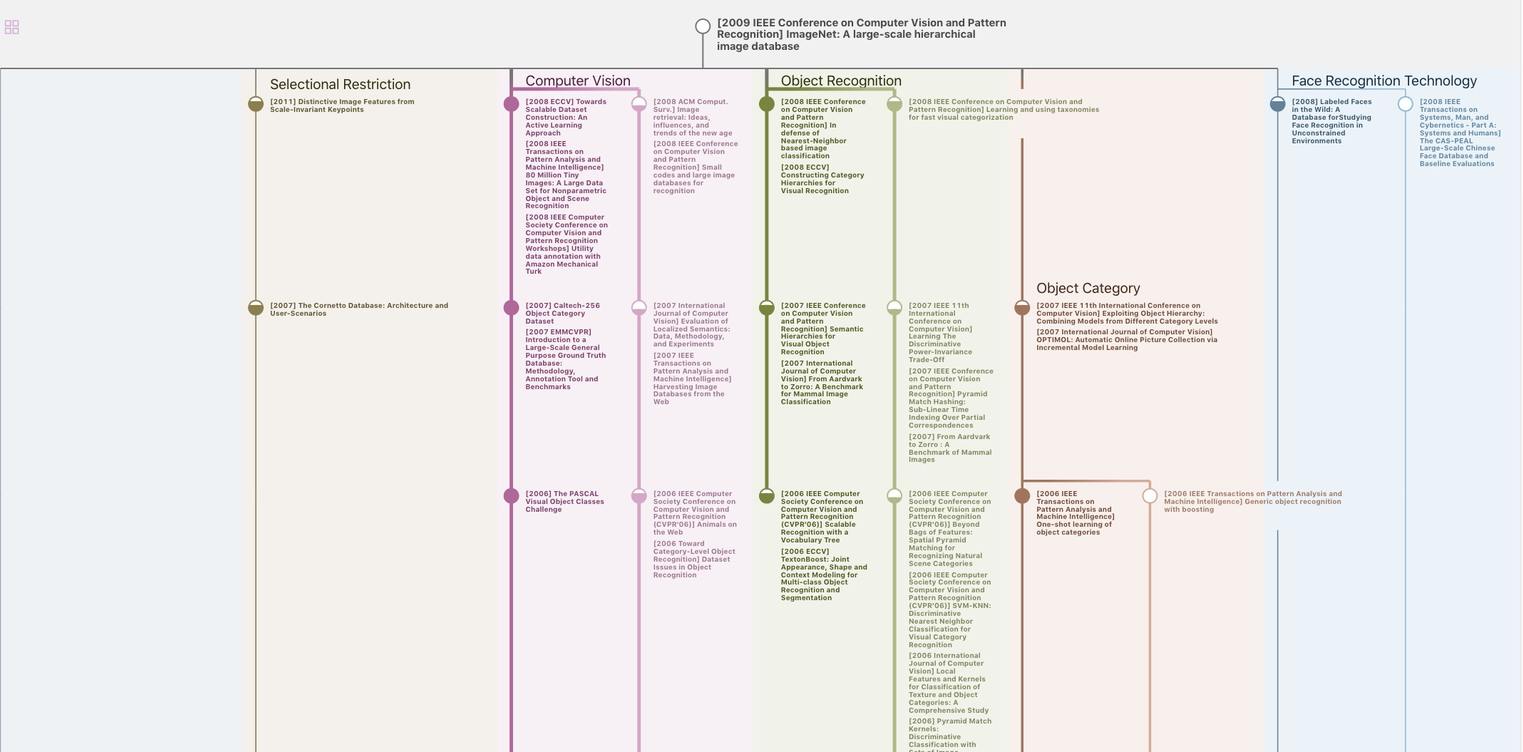
生成溯源树,研究论文发展脉络
Chat Paper
正在生成论文摘要