Content Reduction, Surprisal and Information Density Estimation for Long Documents.
CoRR(2023)
摘要
Many computational linguistic methods have been proposed to study the information content of languages. We consider two interesting research questions: 1) how is information distributed over long documents, and 2) how does content reduction, such as token selection and text summarization, affect the information density in long documents. We present four criteria for information density estimation for long documents, including surprisal, entropy, uniform information density, and lexical density. Among those criteria, the first three adopt the measures from information theory. We propose an attention-based word selection method for clinical notes and study machine summarization for multiple-domain documents. Our findings reveal the systematic difference in information density of long text in various domains. Empirical results on automated medical coding from long clinical notes show the effectiveness of the attention-based word selection method.
更多查看译文
关键词
content reduction,information density estimation,documents
AI 理解论文
溯源树
样例
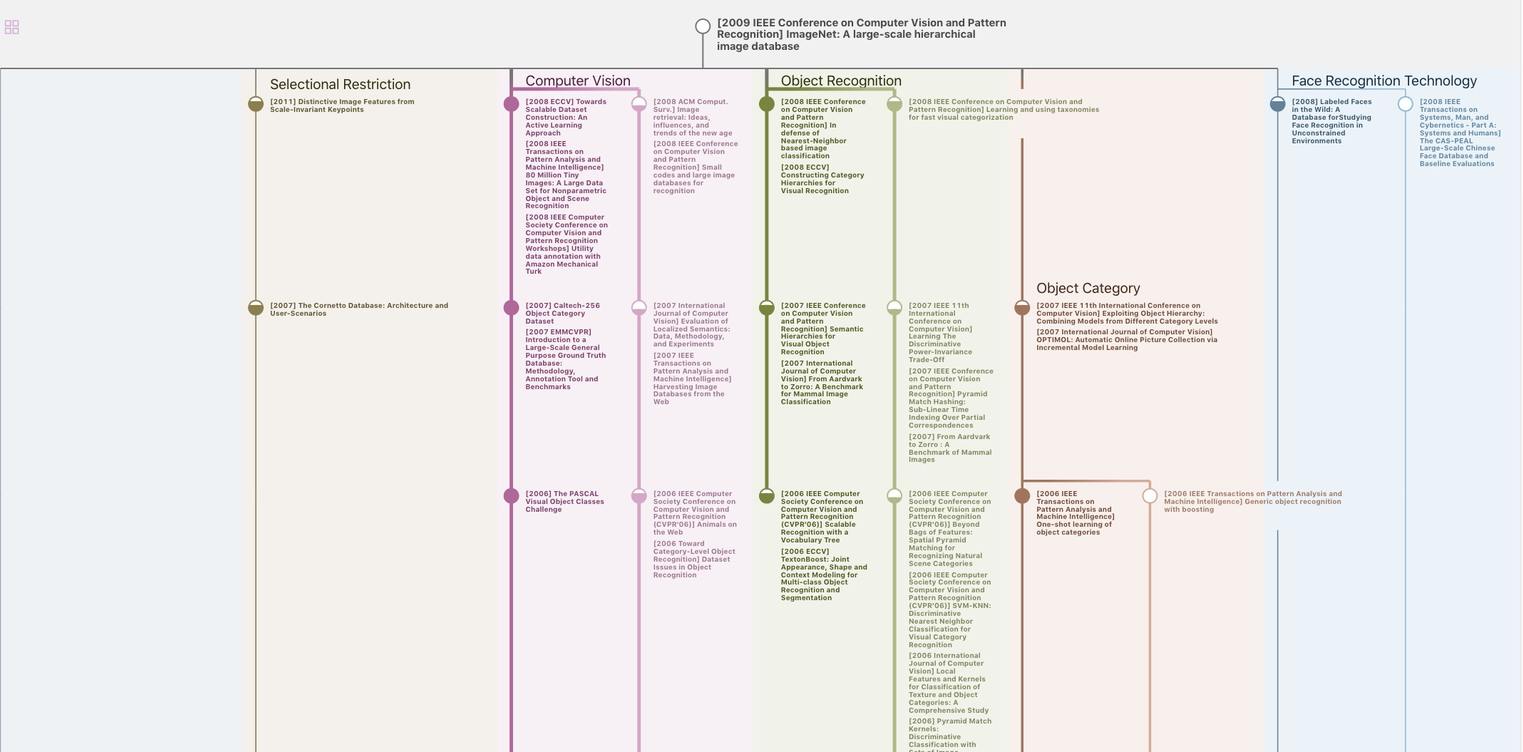
生成溯源树,研究论文发展脉络
Chat Paper
正在生成论文摘要