Revisiting the dynamics of gaseous ammonia and ammonium aerosols during the COVID-19 lockdown in urban Beijing using machine learning models.
The Science of the total environment(2023)
摘要
The concentration of atmospheric ammonia (NH3) in urban Beijing substantially decreased during the COVID-19 lockdown (24 January to 3 March 2020), likely due to the reduced human activities. However, quantifying the impact of anthropogenic interventions on NH3 dynamics is challenging, as both meteorology and chemistry mask the real changes in observed NH3 concentrations. Here, we applied machine learning techniques based on random forest models to decouple the impacts of meteorology and emission changes on the gaseous NH3 and ammonium aerosol (NH4+) concentrations in Beijing during the lockdown. Our results showed that the meteorological conditions were unfavorable during the lockdown and tended to cause an increase of 8.4 % in the NH3 concentration. In addition, significant reductions in NOx and SO2 emissions could also elevate NH3 concentrations by favoring NH3 gas-phase partitioning. However, the observed NH3 concentration significantly decreased by 35.9 % during the lockdown, indicating a significant reduction in emissions or enhanced chemical sinks. Rapid gas-to-particle conversion was indeed found during the lockdown. Thus, the observed reduced NH3 concentrations could be partially explained by the enhanced transformation into NH4+. Therefore, the sum of NH3 and NH4+ (collectively, NHx) is a more reliable tracer than NH3 or NH4+ alone to estimate the changes in NH3 emissions. Compared to that under the scenario without lockdowns, the NHx concentration decreased by 26.4 %. We considered that this decrease represents the real decrease in NH3 emissions in Beijing due to the lockdown measures, which was less of a decrease than that based on NH3 only (35.9 %). This study highlights the importance of considering chemical sinks in the atmosphere when applying machine learning techniques to link the concentrations of reactive species with their emissions.
更多查看译文
AI 理解论文
溯源树
样例
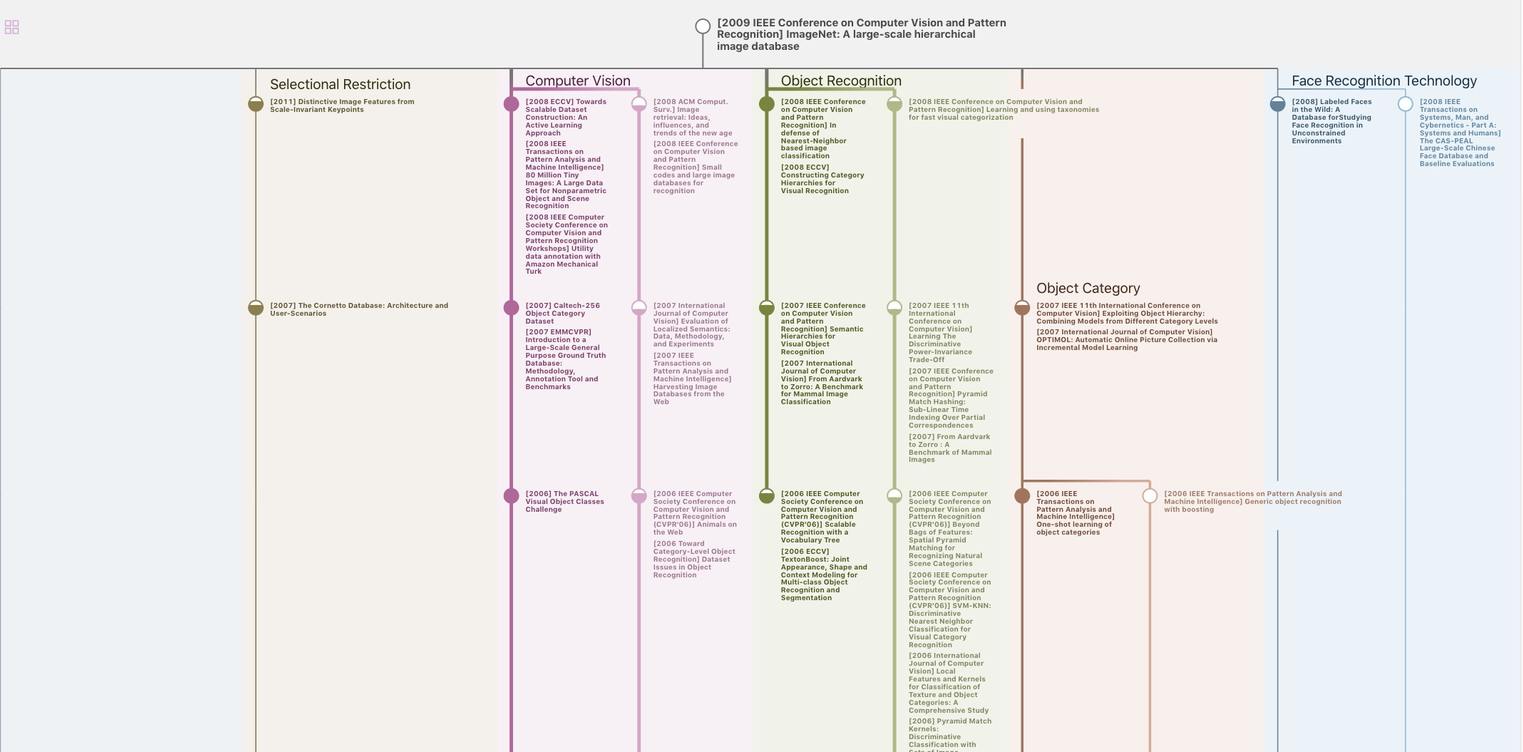
生成溯源树,研究论文发展脉络
Chat Paper
正在生成论文摘要