A motion recognition model for upper-limb rehabilitation exercises
Journal of Ambient Intelligence and Humanized Computing(2023)
摘要
In recent years, autonomous rehabilitation training systems with no or reduced supervision have attracted much research attentions. It is essential to automatically record and recognize motions so as to realize autonomous rehabilitation training. In this paper, we propose a motion recognition model of upper-limb rehabilitation exercise (MRURE) for post-strokes. Wearable inertial measurement unit (IMU) is used to collect motion data including tri-axial acceleration and angular velocity. A Mahalanobis distance-based dynamic time warping (MDDTW) technique and an improved metric learning algorithm Nearest Neighbors Constraint (NNC) are built to measure the similarity between motions. Then, the kNN classifier is adopted to classify motions based on Mahalanobis distance. In order to verify and validate the proposed motion recognition model, we conduct extensive experiments by motion samples collected from healthy participants. The overall recognition accuracy for five motions achieves 99.34%, which demonstrates that MRURE is effective for motion recognition of upper-limb exercises. The performance of MRURE is also compared with kNN based on Euclidean and Manhattan distance and the state-of-the-art motion recognition methods. Experiment results show that the accuracy of kNN based on Mahalanobis distance is better than that of Euclidean and Manhattan distance, and MRURE outperforms methods based on feature extraction.
更多查看译文
关键词
Inertial measurement unit (IMU),Mahalanobis distance,Motion recognition,Rehabilitation exercise
AI 理解论文
溯源树
样例
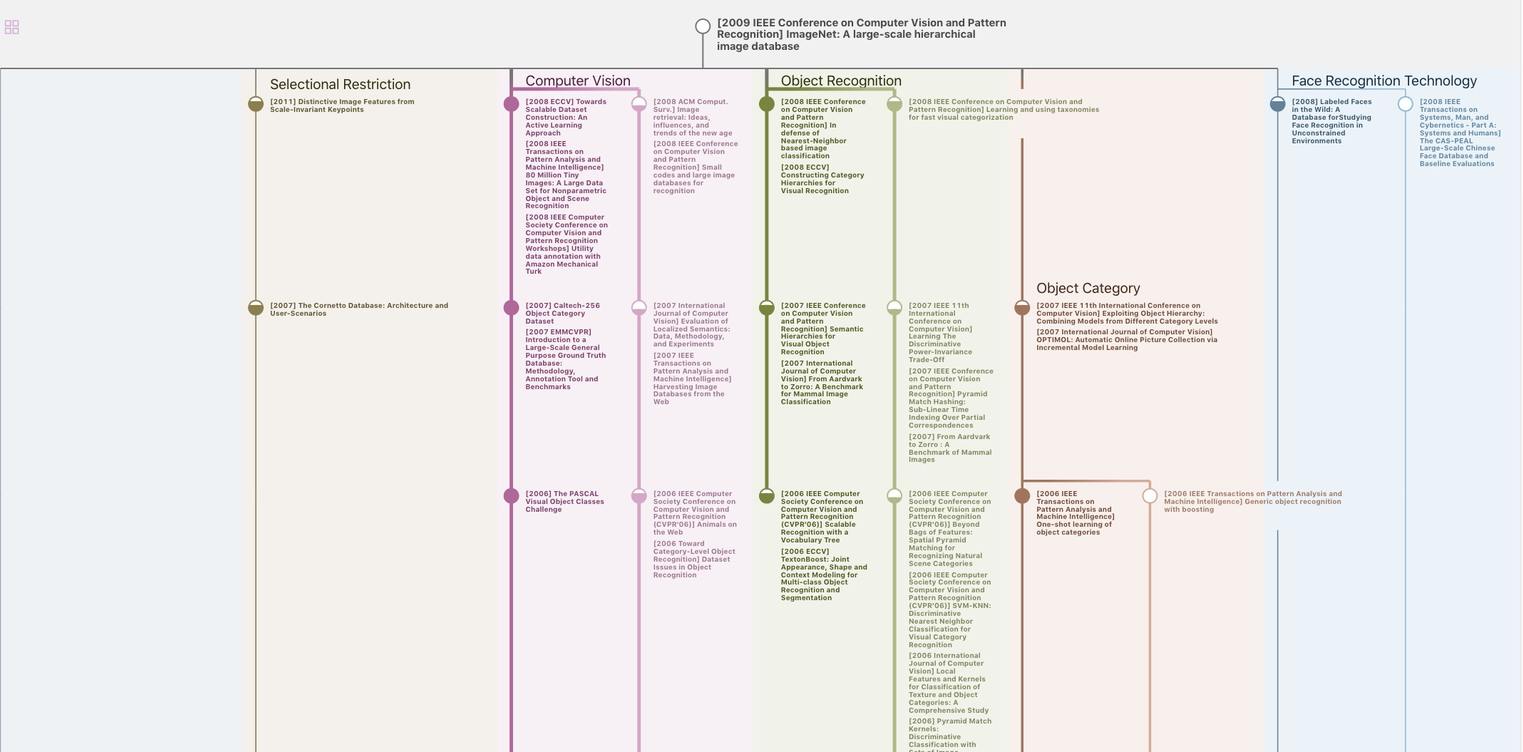
生成溯源树,研究论文发展脉络
Chat Paper
正在生成论文摘要