Predicting Expressibility of Parameterized Quantum Circuits Using Graph Neural Network.
International Conference on Quantum Computing and Engineering(2023)
摘要
Parameterized Quantum Circuits (PQCs) are essential to quantum machine learning and optimization algorithms. The expressibility of PQCs, which measures their ability to represent a wide range of quantum states, is a critical factor influencing their efficacy in solving quantum problems. However, the existing technique for computing expressibility relies on statistically estimating it through classical simulations, which requires many samples. In this work, we propose a novel method based on Graph Neural Networks (GNNs) for predicting the expressibility of PQCs. By leveraging the graph-based representation of PQCs, our GNN-based model captures intricate relationships between circuit parameters and their resulting expressibility. We train the GNN model on a comprehensive dataset of PQCs annotated with their expressibility values. Experimental evaluation on a four thousand random PQC dataset and IBM Qiskit's hardware efficient ansatz sets demonstrates the superior performance of our approach, achieving a root mean square error (RMSE) of 0.03 and 0.06, respectively.
更多查看译文
关键词
parameterized quantum circuits,neural network,graph
AI 理解论文
溯源树
样例
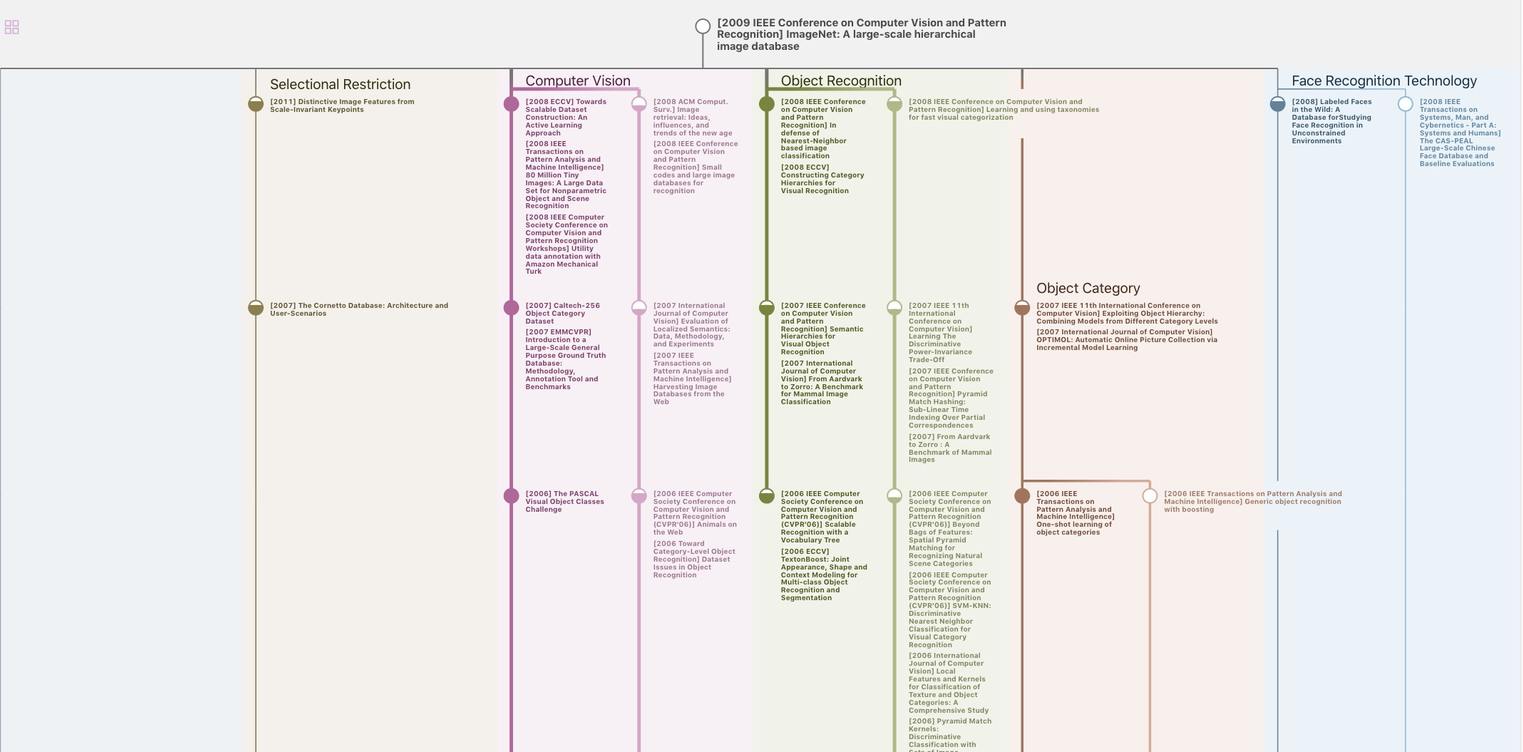
生成溯源树,研究论文发展脉络
Chat Paper
正在生成论文摘要