Real-time quantum dynamics of thermal states with neural thermofields
arXiv (Cornell University)(2023)
摘要
Solving the time-dependent quantum many-body Schr\"odinger equation is a challenging task, especially for states at a finite temperature, where the environment affects the dynamics. Most existing approximating methods are designed to represent static thermal density matrices, 1D systems, and/or zero-temperature states. In this work, we propose a method to study the real-time dynamics of thermal states in two dimensions, based on thermofield dynamics, variational Monte Carlo, and neural-network quantum states. To this aim, we introduce two novel tools: (i) a procedure to accurately simulate the cooling down of arbitrary quantum variational states from infinite temperature, and (ii) a generic thermal (autoregressive) recurrent neural-network (ARNNO) Ansatz that allows for direct sampling from the density matrix using thermofield basis rotations. We apply our technique to the transverse-field Ising model subject to an additional longitudinal field and demonstrate that the time-dependent observables, including correlation operators, can be accurately reproduced for a 4x4 spin lattice. We provide predictions of the real-time dynamics on a 6x6 lattice that lies outside the reach of exact simulations.
更多查看译文
关键词
thermal states,quantum,dynamics,neural,real-time
AI 理解论文
溯源树
样例
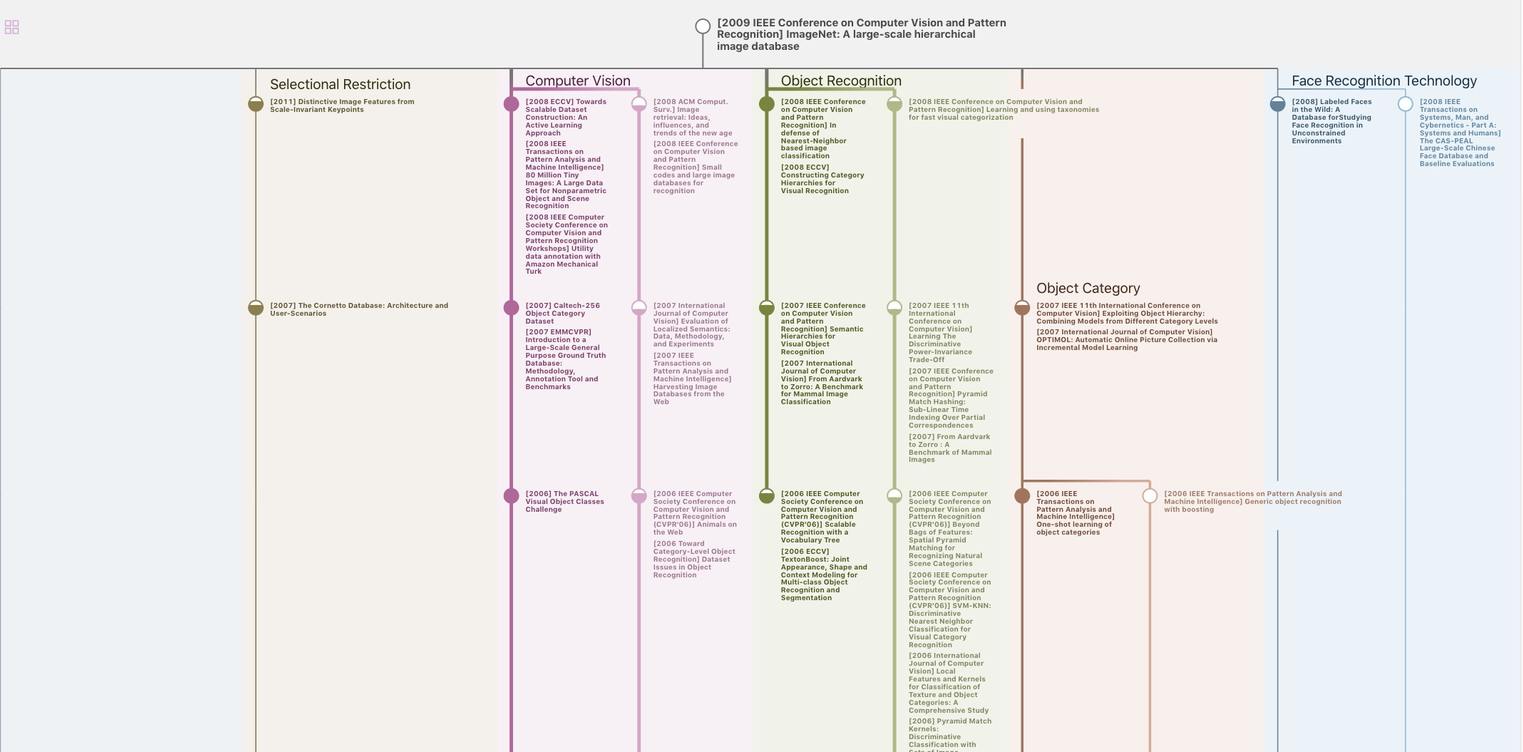
生成溯源树,研究论文发展脉络
Chat Paper
正在生成论文摘要