Dimensionality reduction for deep learning in infrared microscopy: a comparative computational survey.
The Analyst(2023)
摘要
While infrared microscopy provides molecular information at spatial resolution in a label-free manner, exploiting both spatial and molecular information for classifying the disease status of tissue samples constitutes a major challenge. One strategy to mitigate this problem is to embed high-dimensional pixel spectra in lower dimensions, aiming to preserve molecular information in a more compact manner, which reduces the amount of data and promises to make subsequent disease classification more accessible for machine learning procedures. In this study, we compare several dimensionality reduction approaches and their effect on identifying cancer in the context of a colon carcinoma study. We observe surprisingly small differences between convolutional neural networks trained on dimensionality reduced spectra compared to utilizing full spectra, indicating a clear tendency of the convolutional networks to focus on spatial rather than spectral information for classifying disease status.
更多查看译文
关键词
infrared microscopy,deep learning
AI 理解论文
溯源树
样例
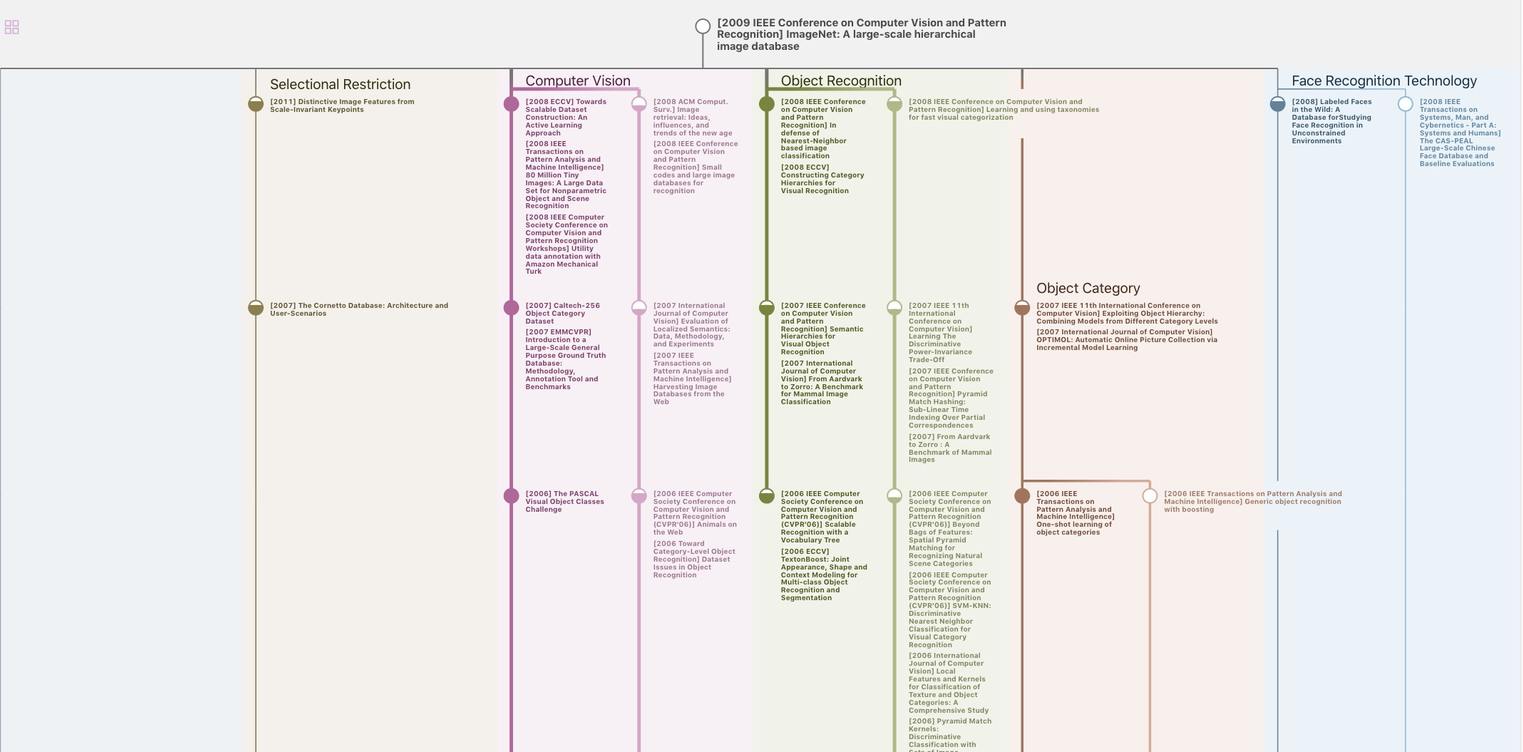
生成溯源树,研究论文发展脉络
Chat Paper
正在生成论文摘要