Toward Lossless Homomorphic Encryption for Scientific Computation.
CoRR(2023)
摘要
This paper presents a comprehensive investigation into encrypted computations using the CKKS (Cheon-Kim-Kim-Song) scheme, with a focus on multi-dimensional vector operations and real-world applications. Through two meticulously designed experiments, the study explores the potential of the CKKS scheme in Super Computing and its implications for data privacy and computational efficiency. The first experiment reveals the promising applicability of CKKS to matrix multiplication, indicating marginal differences in Euclidean distance and near-to-zero mean square error across various matrix sizes. The second experiment, applied to a wildfire dataset, illustrates the feasibility of using encrypted machine learning models without significant loss in accuracy. The insights gleaned from the research set a robust foundation for future innovations, including the potential for GPU acceleration in CKKS computations within TenSEAL. Challenges such as noise budget computation, accuracy loss in multiplication, and the distinct characteristics of arithmetic operations in the context of CKKS are also discussed. The paper serves as a vital step towards understanding the complexities and potentials of encrypted computations, with broad implications for secure data processing and privacy preservation in various scientific domains.
更多查看译文
关键词
lossless homomorphic encryption,scientific computation
AI 理解论文
溯源树
样例
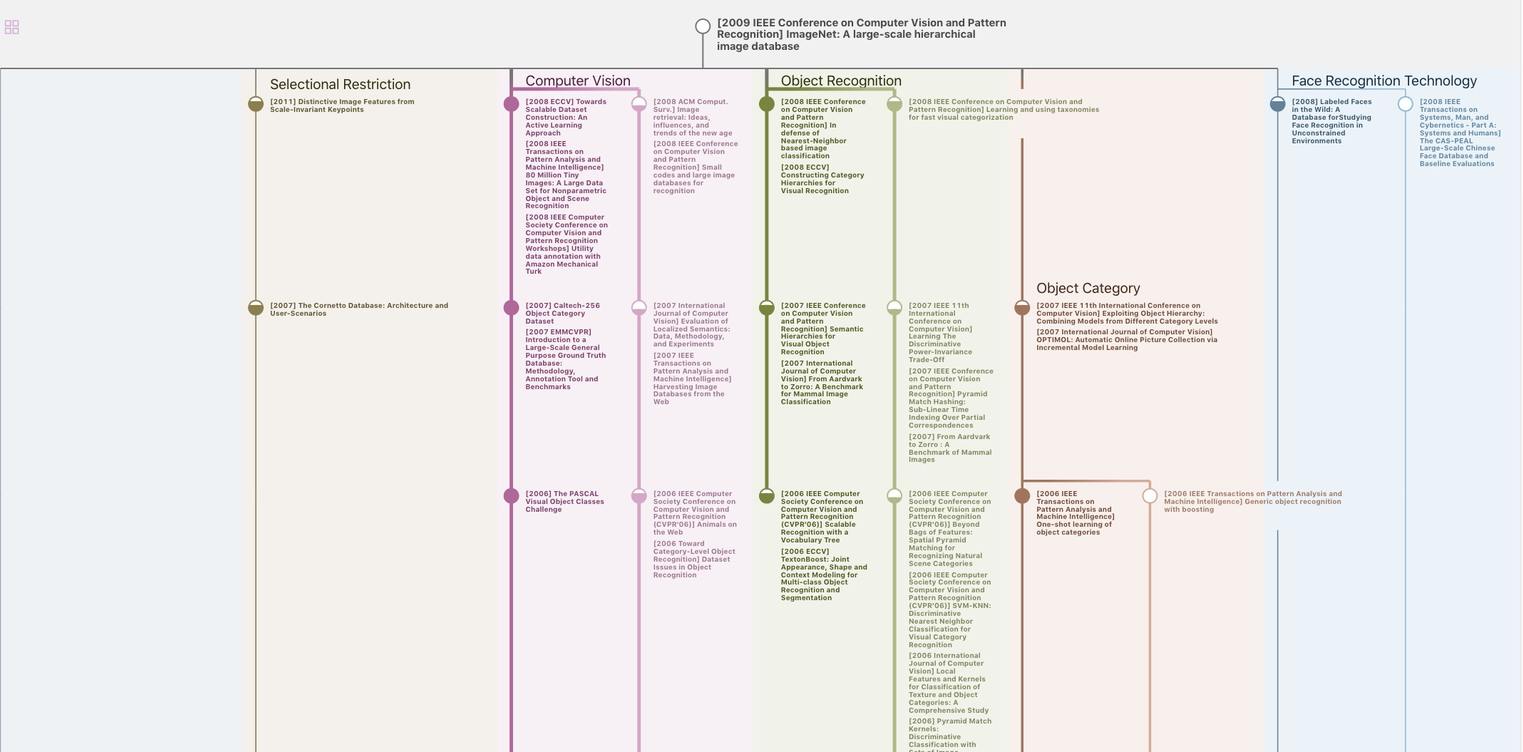
生成溯源树,研究论文发展脉络
Chat Paper
正在生成论文摘要