C2IMUFS: Complementary and Consensus Learning-Based Incomplete Multi-View Unsupervised Feature Selection
IEEE Transactions on Knowledge and Data Engineering(2023)
摘要
Multi-view unsupervised feature selection (MUFS) has been demonstrated as an effective technique to reduce the dimensionality of multi-view unlabeled data. The existing methods assume that all of views are complete. However, multi-view data are usually incomplete, i.e., a part of instances are presented on some views but not all views. Besides, learning the complete similarity graph, as an important promising technology in existing MUFS methods, cannot achieve due to the missing views. In this paper, we propose a complementary and consensus learning-based incomplete multi-view unsupervised feature selection method (C
$^{2}$
IMUFS) to address the aforementioned issues. Concretely, C
$^{2}$
IMUFS integrates feature selection into an extended weighted non-negative matrix factorization model equipped with adaptive learning of view-weights and a sparse
$\ell _{2,p}$
-norm, which can offer better adaptability and flexibility. By the sparse linear combinations of multiple similarity matrices derived from different views, a complementary learning-guided similarity matrix reconstruction model is presented to obtain the complete similarity graph in each view. Furthermore, C
$^{2}$
IMUFS learns a consensus clustering indicator matrix across different views and embeds it into a spectral graph term to preserve the local geometric structure. Comprehensive experimental results on real-world datasets demonstrate the effectiveness of C
$^{2}$
IMUFS compared with state-of-the-art methods.
更多查看译文
关键词
Complementary and consensus information, incomplete multi-view data, unsupervised feature selection, weighted non-negative matrix factorization
AI 理解论文
溯源树
样例
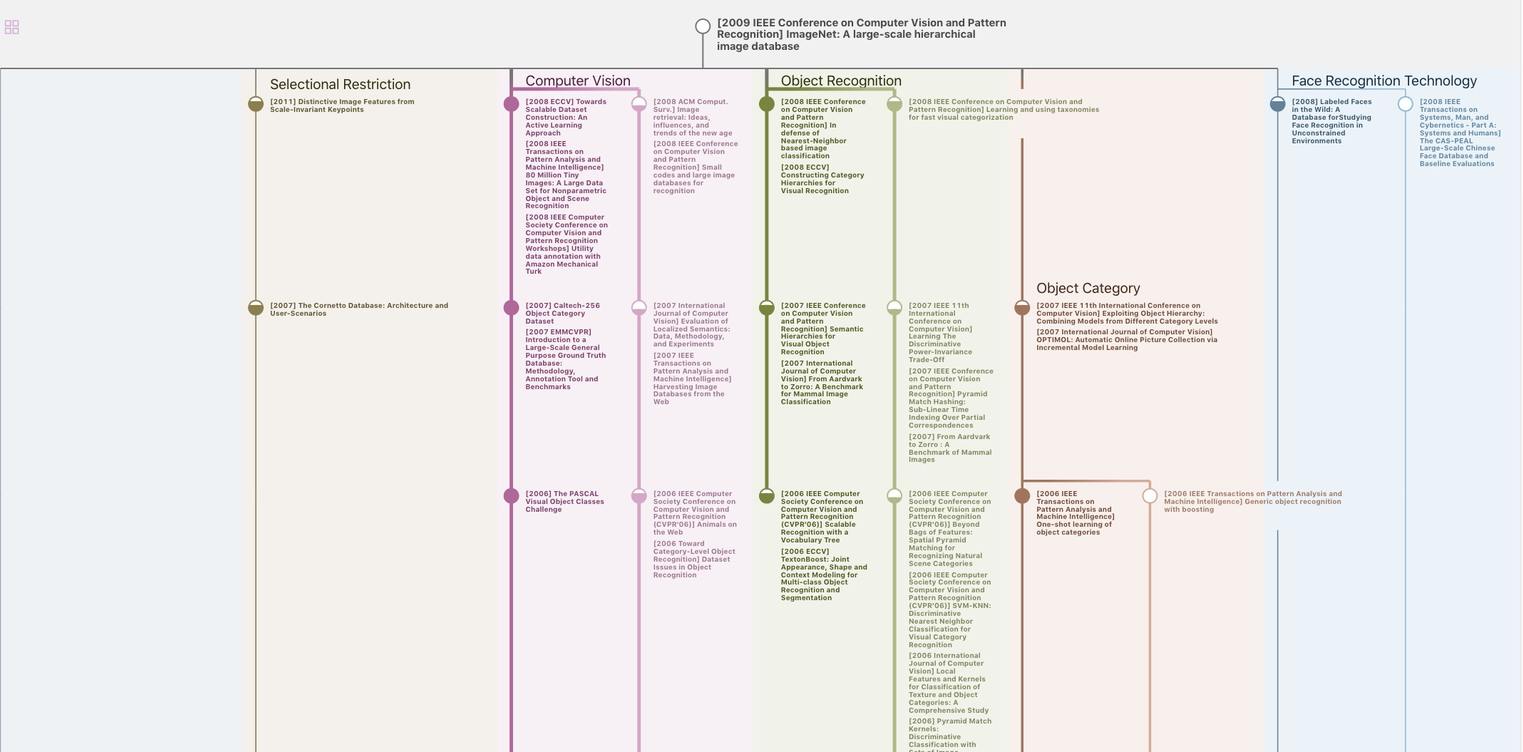
生成溯源树,研究论文发展脉络
Chat Paper
正在生成论文摘要