Coarse-Grained Modeling Using Neural Networks Trained on Structural Data.
Journal of chemical theory and computation(2023)
摘要
We propose a method of bottom-up coarse-graining, in which interactions within a coarse-grained model are determined by an artificial neural network trained on structural data obtained from multiple atomistic simulations. The method uses ideas of the inverse Monte Carlo approach, relating changes in the neural network weights with changes in average structural properties, such as radial distribution functions. As a proof of concept, we demonstrate the method on a system interacting by a Lennard-Jones potential modeled by a simple linear network and a single-site coarse-grained model of methanol-water solutions. In the latter case, we implement a nonlinear neural network with intermediate layers trained by atomistic simulations carried out at different methanol concentrations. We show that such a network acts as a transferable potential at the coarse-grained resolution for a wide range of methanol concentrations, including those not included in the training set.
更多查看译文
关键词
neural networks,modeling,structural,coarse-grained
AI 理解论文
溯源树
样例
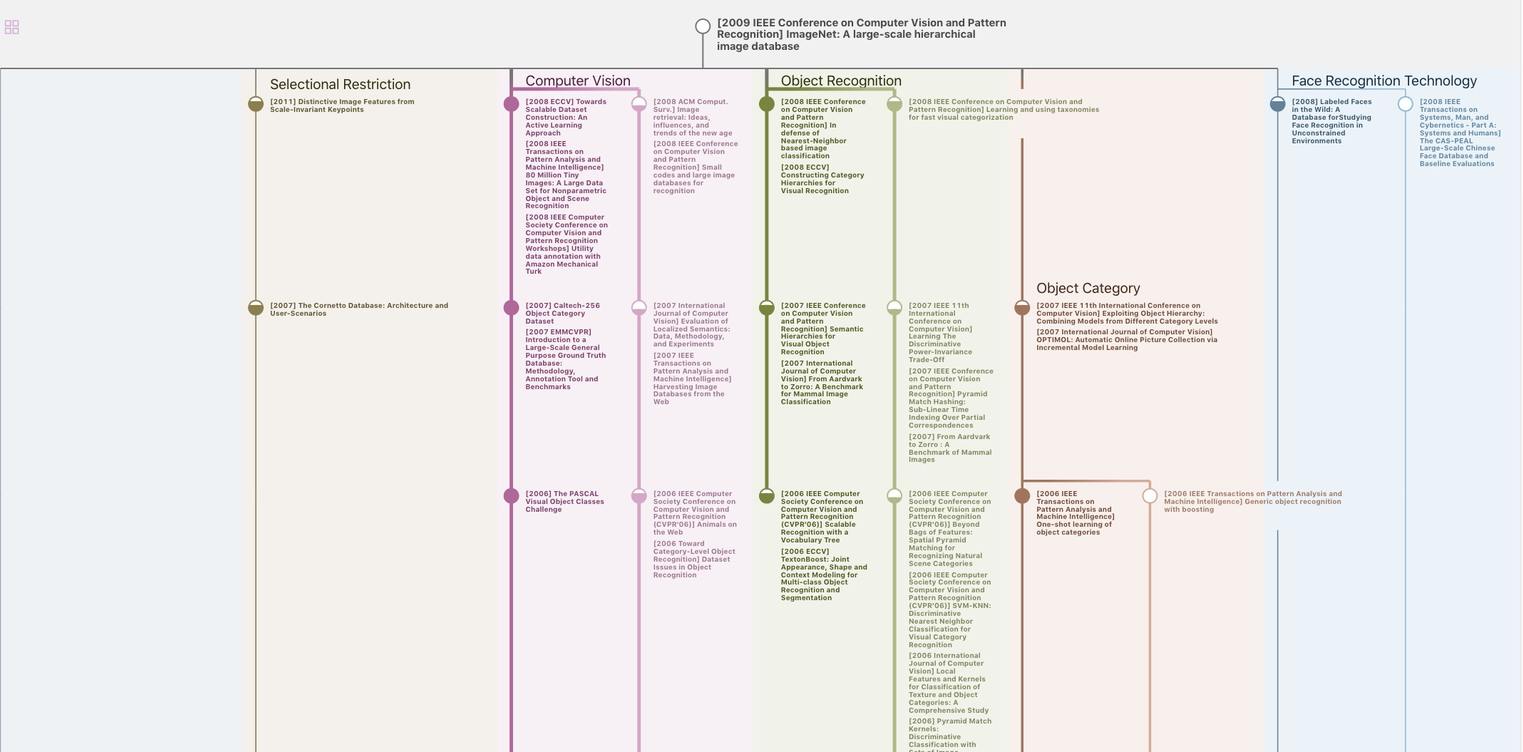
生成溯源树,研究论文发展脉络
Chat Paper
正在生成论文摘要