Multimodality Driven Impedance-Based Sim2Real Transfer Learning for Robotic Multiple Peg-in-Hole Assembly
IEEE TRANSACTIONS ON CYBERNETICS(2024)
摘要
Robotic rigid contact-rich manipulation in an unstructured dynamic environment requires an effective resolution for smart manufacturing. As the most common use case for the intelligence industry, a lot of studies based on reinforcement learning (RL) algorithms have been conducted to improve the performances of single peg-in-hole assembly. However, existing RL methods are difficult to apply to multiple peg-in-hole issues due to more complicated geometric and physical constraints. In addition, previously limited solutions for multiple peg-in-hole assembly are hard to transfer into real industrial scenarios flexibly. To effectively address these issues, this work designs a novel and more challenging multiple peg-in-hole assembly setup by using the advantage of the Industrial Metaverse. We propose a detailed solution scheme to solve this task. Specifically, multiple modalities, including vision, proprioception, and force/torque, are learned as compact representations to account for the complexity and uncertainties and improve the sample efficiency. Furthermore, RL is used in the simulation to train the policy, and the learned policy is transferred to the real world without extra exploration. Domain randomization and impedance control are embedded into the policy to narrow the gap between simulation and reality. Evaluation results demonstrate the effectiveness of the proposed solution, showcasing successful multiple peg-in-hole assembly and generalization across different object shapes in real-world scenarios.
更多查看译文
关键词
Task analysis,Robots,Impedance,Visualization,Metaverse,Shape,Service robots,Impedance control,multimodal learning,RL,robotic multiple peg-in-hole assembly,sim2real-based transfer learning
AI 理解论文
溯源树
样例
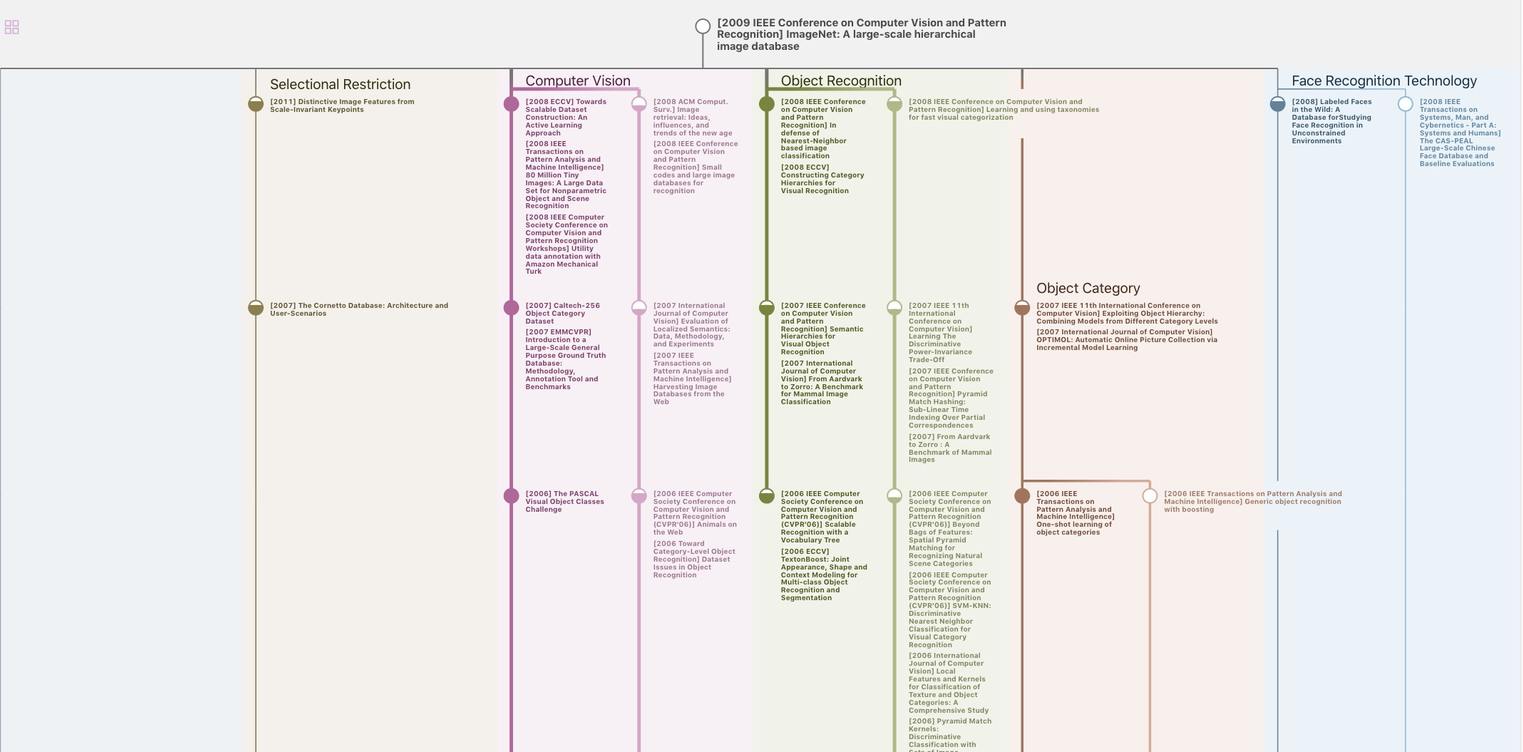
生成溯源树,研究论文发展脉络
Chat Paper
正在生成论文摘要